Rainfall-Runoff Modeling Using Lstm-Based Multi-State-Vector Sequence-To-Sequence Model
JOURNAL OF HYDROLOGY(2021)
摘要
Rainfall-runoff modeling is a challenging and important nonlinear time series problem in hydrological sciences. Recently, among the data-driven rainfall-runoff models, those ones based on the long short-term memory (LSTM) network show good performance. Furthermore, LSTM-based sequence-to-sequence (LSTM-S2S) models achieve promising performance for multi-step-ahead runoff predictions. In this paper, for multi-day-ahead runoff predictions, we propose a novel data-driven model named LSTM-based multi-state-vector sequence-to-sequence (LSTM-MSV-S2S) rainfall-runoff model, which contains m multiple state vectors for m-step-ahead runoff predictions. It differs from the existing LSTM-S2S rainfall-runoff models using only one state vector and is more appropriate for multi-day-ahead runoff predictions. To show its performance and advantages, we compare it with two LSTM-S2S models by testing them on 673 basins of the Catchment Attributes and Meteorology for LargeSample Studies (CAMELS) data set. The results show that our LSTM-MSV-S2S model has better performance in general and thus using multiple state vectors is more appropriate for multi-day-ahead runoff predictions.
更多查看译文
关键词
Rainfall-runoff model, Long short-term memory, Sequence-to-sequence, Recurrent neural network
AI 理解论文
溯源树
样例
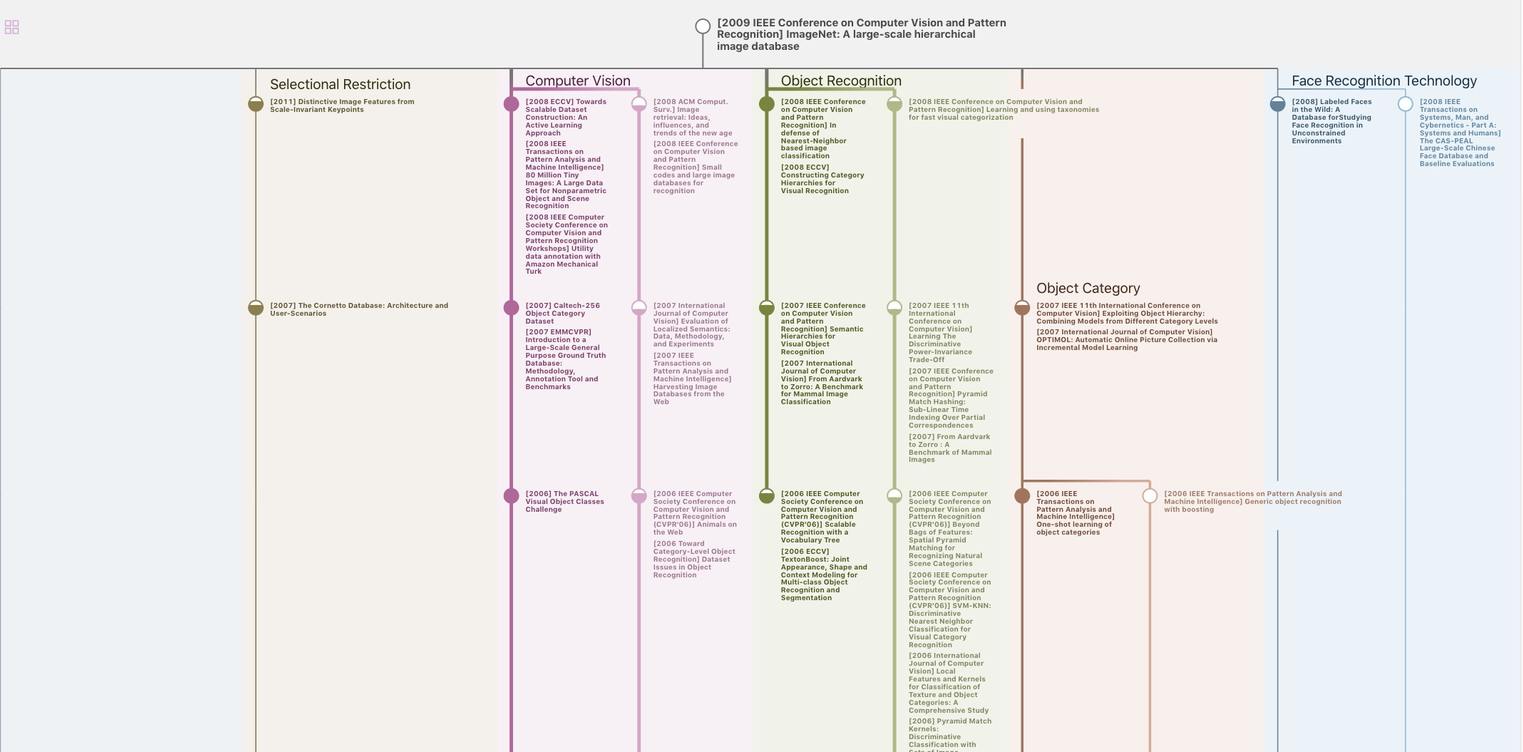
生成溯源树,研究论文发展脉络
Chat Paper
正在生成论文摘要