Active and Compact Entropy Search for High-Dimensional Bayesian Optimization
IEEE Transactions on Knowledge and Data Engineering(2023)
摘要
Entropy search and its derivative methods are one class of Bayesian Optimization methods that achieve active exploration of black-box functions. They maximize the information gain about the position in the input space where the black-box function gets the global optimum. However, existing entropy search methods suffer from harassment caused by high dimensional optimization problems. On the one hand, the computation for estimating entropies increases exponentially as dimensions increase, which limits the applicability of entropy search to high dimensional problems. On the other hand, many high-dimensional problems have the property that a large number of dimensions have little influence on the objective function, but currently there is no compress mechanism to exclude these redundant dimensions. In this work, we propose Active Compact Entropy Search (AcCES) to fix the above two defects. Under the guidance of historical evaluation, we bring forward a novel acquisition function that considers the correlation between dimensions in entropy search, which is ignored by existing Bayesian Optimization methods. The correlation term added in the acquisition function will help discover the potential correlation between dimensions. In order to build a more compact input space, redundant dimensions are compressed by exploiting inter-dimensional correlations. We use Pearson Correlation Coefficient and curve fitting to represent the inter-dimensional correlations. Extensive experiments on several benchmarks demonstrate that AcCES achieves higher query efficiency as well as optimal results after convergence than existing entropy search methods.
更多查看译文
关键词
Bayesian optimization,entropy search,high dimensionality
AI 理解论文
溯源树
样例
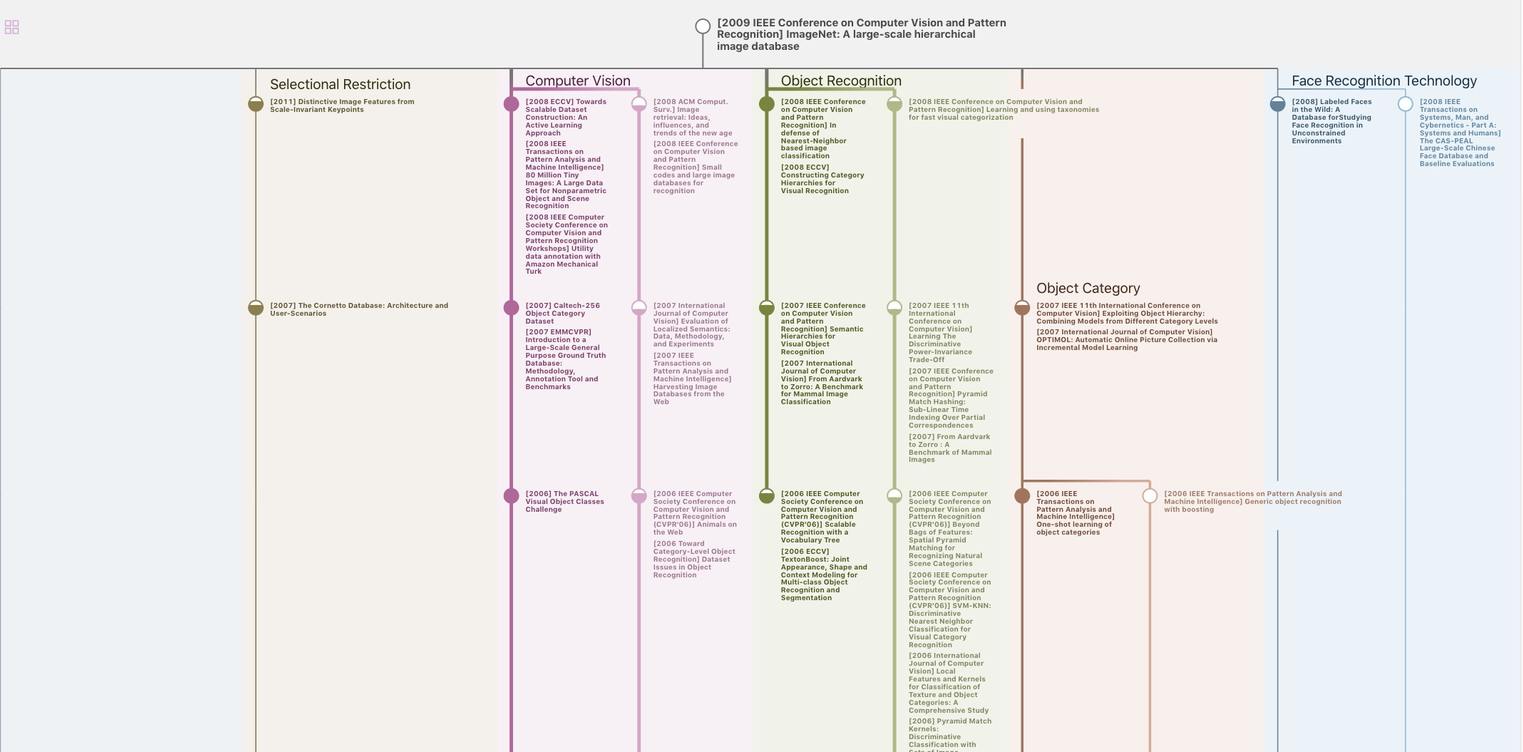
生成溯源树,研究论文发展脉络
Chat Paper
正在生成论文摘要