Combination Of Network And Molecule Structure Accurately Predicts Competitive Inhibitory Interactions
COMPUTATIONAL AND STRUCTURAL BIOTECHNOLOGY JOURNAL(2021)
摘要
Mining of metabolite-protein interaction networks facilitates the identification of design principles underlying the regulation of different cellular processes. However, identification and characterization of the regulatory role that metabolites play in interactions with proteins on a genome-scale level remains a pressing task. Based on availability of high-quality metabolite-protein interaction networks and genome-scale metabolic networks, here we propose a supervised machine learning approach, called CIRI that determines whether or not a metabolite is involved in a competitive inhibitory regulatory interaction with an enzyme. First, we show that CIRI outperforms the naive approach based on a structural similarity threshold for a putative competitive inhibitor and the substrates of a metabolic reaction. We also validate the performance of CIRI on several unseen data sets and databases of metabolite-protein interactions not used in the training, and demonstrate that the classifier can be effectively used to predict competitive inhibitory interactions. Finally, we show that CIRI can be employed to refine predictions about metabolite-protein interactions from a recently proposed PROMIS approach that employs metabolomics and proteomics profiles from size exclusion chromatography in E. coli to predict metaboliteprotein interactions. Altogether, CIRI fills a gap in cataloguing metabolite-protein interactions and can be used in directing future machine learning efforts to categorize the regulatory type of these interactions. (C) 2021 The Author(s). Published by Elsevier B.V. on behalf of Research Network of Computational and Structural Biotechnology.
更多查看译文
关键词
Metabolite-protein interactions, Genome-scale metabolic models, Supervised machine learning
AI 理解论文
溯源树
样例
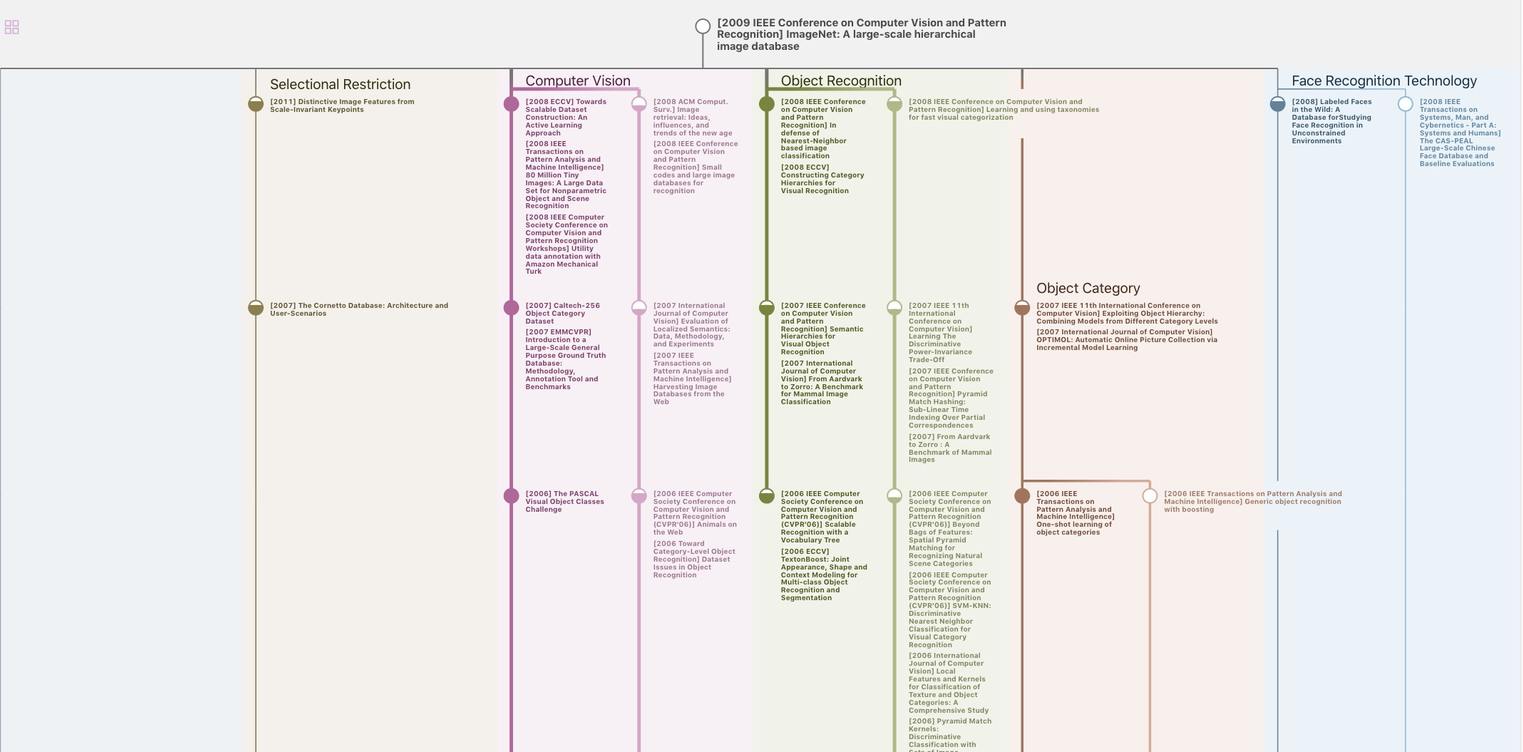
生成溯源树,研究论文发展脉络
Chat Paper
正在生成论文摘要