A Discrete Wavelet Transform-Based Voice Activity Detection and Noise Classification With Sub-Band Selection
2021 IEEE INTERNATIONAL SYMPOSIUM ON CIRCUITS AND SYSTEMS (ISCAS)(2021)
摘要
A real-time discrete wavelet transform-based adaptive voice activity detector and sub-band selection for feature extraction are proposed for noise classification, which can be used in a speech processing pipeline. The voice activity detection and sub-band selection rely on wavelet energy features and the feature extraction process involves the extraction of mel-frequency cepstral coefficients from selected wavelet sub-bands and mean absolute values of all sub-bands. The method combined with a feedforward neural network with two hidden layers could be added to speech enhancement systems and deployed in hearing devices such as cochlear implants. In comparison to the conventional short-time Fourier transform-based technique, it has higher F-1 scores and classification accuracies (with a mean of 0.916 and 90.1%, respectively) across five different noise types (babble, factory, pink, Volvo (car) and white noise), a significantly smaller feature set with 21 features, reduced memory requirement, faster training convergence and about half the computational cost.
更多查看译文
关键词
Discrete wavelet transform, mel-frequency cepstral coefficients, multilayer perceptron, noise classification, sub-band selection, voice activity detection
AI 理解论文
溯源树
样例
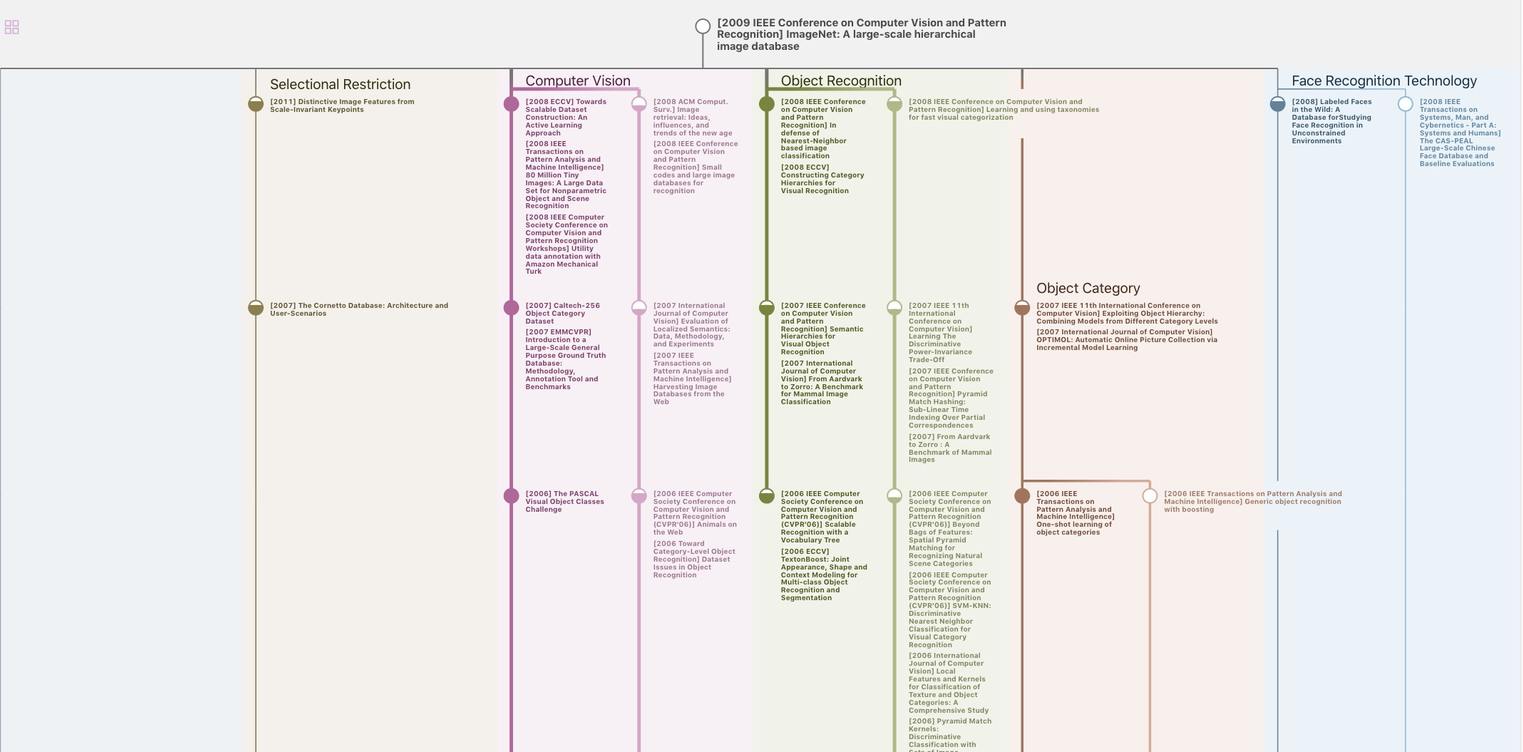
生成溯源树,研究论文发展脉络
Chat Paper
正在生成论文摘要