Adaptive Hash Attention and Lower Triangular Network for Hyperspectral Image Classification
IEEE TRANSACTIONS ON GEOSCIENCE AND REMOTE SENSING(2022)
摘要
Convolutional neural networks (CNNs), a kind of feedforward neural network with a deep structure, are one of the representative methods in hyperspectral image (HSI) classification. However, redundant information and interclass interference are common and challenging problems in HSI classification. In addition, if the spectral and spatial information is not properly extracted and analyzed, it will affect the classification performance of the network to a great extent. Aiming at these issues, this article proposes an HSI classification method based on an adaptive hash attention mechanism and a lower triangular network (AHA-LT). First, the attention mechanism is introduced in the preprocessing stage, which is composed of the spectral attention module and the adaptive hash spatial attention module in series. Then, the data processed by the attention mechanism are introduced into the lower triangular network (LTNet) to obtain the fused high-dimensional semantic features. Finally, we compress the features and obtain the output classification results through several fully connected layers. Among them, LTNet is composed of 2-D-3-D CNN and multiscale features. The network integrates the characteristics of multibranch, feature fusion, feature compression, and skip connections. Extensive experiments on four widely used HSI data sets show that the proposed method can obtain a great improvement in performance compared with the existing methods.
更多查看译文
关键词
Feature extraction, Convolution, Hyperspectral imaging, Task analysis, Image coding, Germanium, Data models, 2-D convolutional neural network (CNN), 3-D CNN, deep learning, feature fusion, hash encoding, hyperspectral image (HSI) classification, spectral-spatial attention mechanism, skip connection
AI 理解论文
溯源树
样例
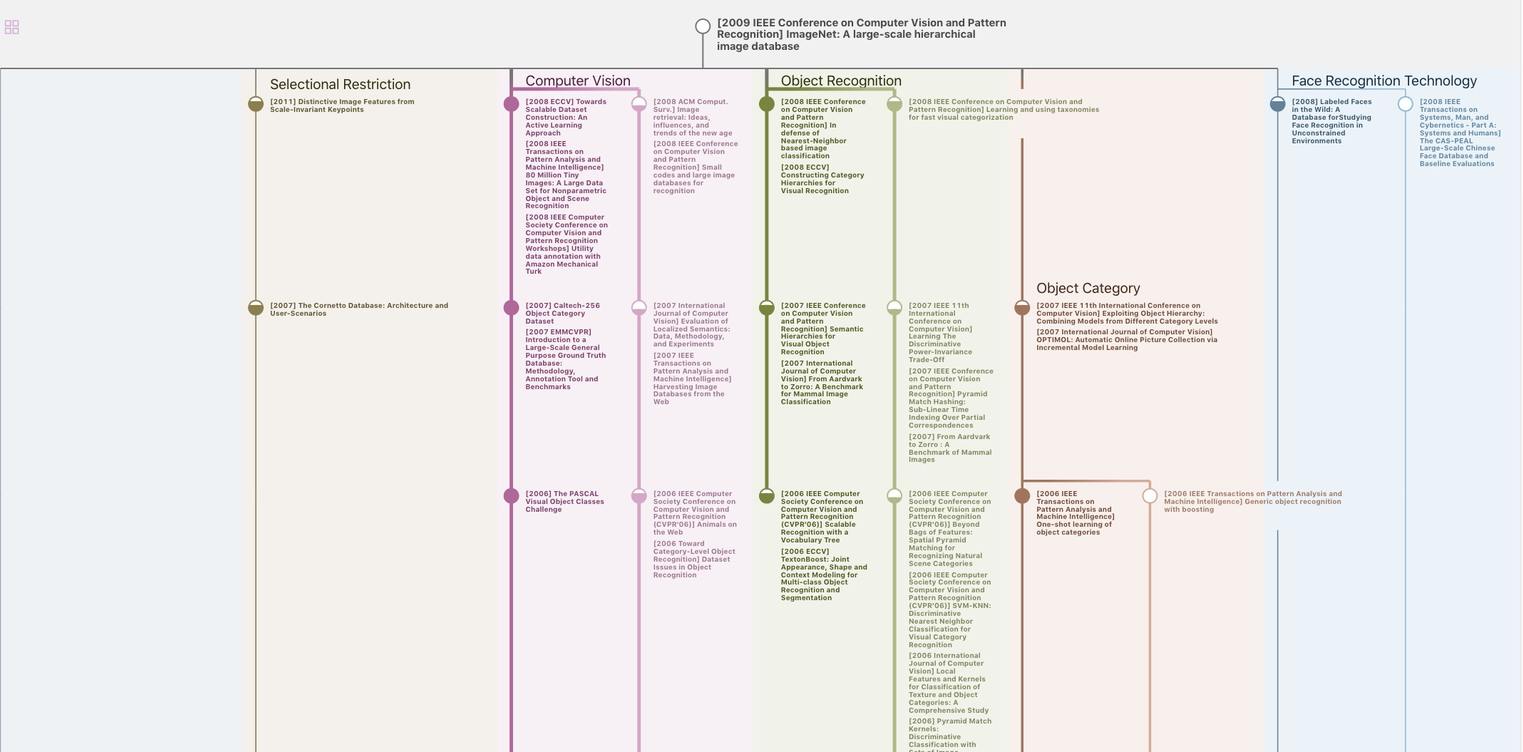
生成溯源树,研究论文发展脉络
Chat Paper
正在生成论文摘要