Compressed Sensing By Phase Change Memories: Coping With Encoder Non-Linearities
2021 IEEE INTERNATIONAL SYMPOSIUM ON CIRCUITS AND SYSTEMS (ISCAS)(2021)
摘要
Several recent works have shown the advantages of using phase-change memory (PCM) in developing brain-inspired computing approaches. In particular, PCM cells have been applied to the direct computation of matrix-vector multiplications in the analog domain. However, the intrinsic nonlinearity of these cells with respect to the applied voltage is detrimental. In this paper we consider a PCM array as the encoder in a Compressed Sensing (CS) acquisition system, and investigate the effect of the non-linearity of the cells. We introduce a CS decoding strategy that is able to compensate for PCM nonlinearities by means of an iterative approach. At each step, the current signal estimate is used to approximate the average behaviour of the PCM cells used in the encoder. Monte Carlo simulations relying on a PCM model extracted from an STMicrolectronics 90 nm BCD chip validate the performance of the algorithm with various degrees of nonlinearities, showing up to 35 dB increase in median performance as compared to standard decoding procedures.
更多查看译文
关键词
phase change memories,encoder nonlinearities,phase-change memory,brain-inspired computing approaches,particular cells,PCM cells,direct computation,matrix-vector multiplications,analog domain,intrinsic nonlinearity,applied voltage,PCM array,Compressed Sensing acquisition system,CS decoding strategy,PCM nonlinearities,iterative approach,PCM model,size 90.0 nm,noise figure 35.0 dB
AI 理解论文
溯源树
样例
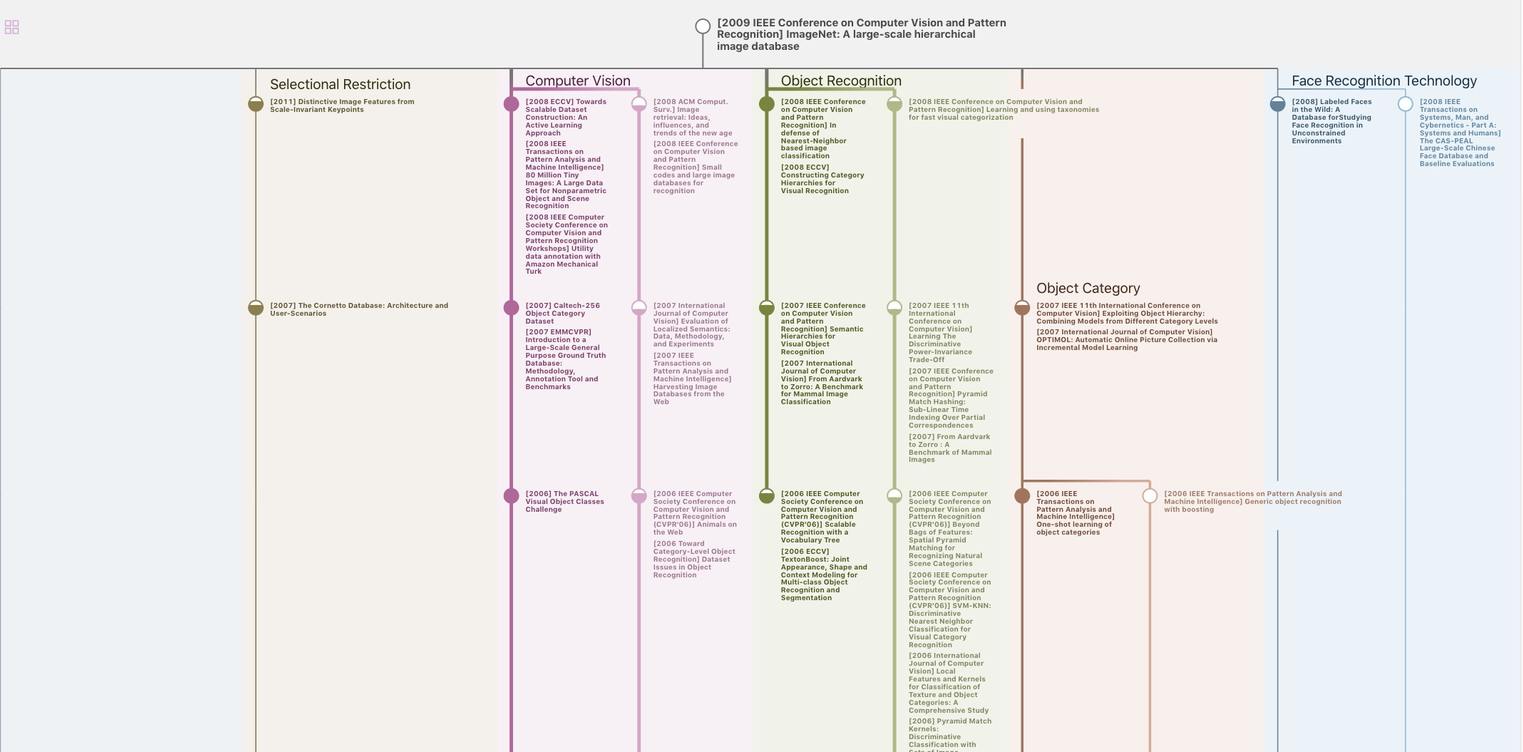
生成溯源树,研究论文发展脉络
Chat Paper
正在生成论文摘要