Modeling Laser-Driven Ion Acceleration With Deep Learning
PHYSICS OF PLASMAS(2021)
摘要
Developments in machine learning promise to ameliorate some of the challenges of modeling complex physical systems through neural-network-based surrogate models. High-intensity, short-pulse lasers can be used to accelerate ions to mega-electronvolt energies, but to model such interactions requires computationally expensive techniques such as particle-in-cell simulations. Multilayer neural networks allow one to take a relatively sparse ensemble of simulations and generate a surrogate model that can be used to rapidly search the parameter space of interest. In this work, we created an ensemble of over 1,000 simulations modeling laser-driven ion acceleration and developed a surrogate to study the resulting parameter space. A neural-network-based approach allows for rapid feature discovery not possible for traditional parameter scans given the computational cost. A notable observation made during this study was the dependence of ion energy on the pre-plasma gradient length scale. While this methodology harbors great promise for ion acceleration, it has ready application to all topics in which large-scale parameter scans are restricted by significant computational cost or relatively large, but sparse, domains.
更多查看译文
AI 理解论文
溯源树
样例
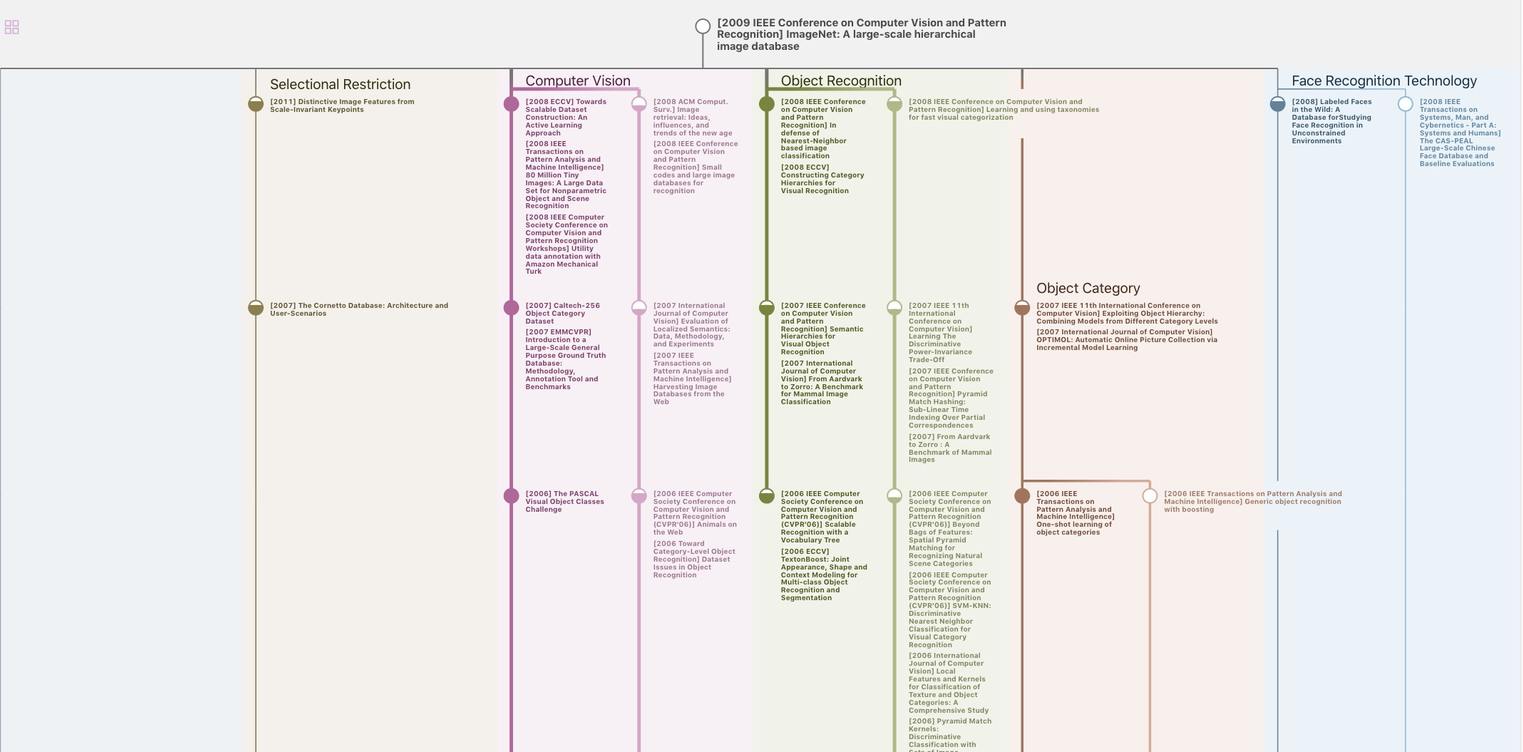
生成溯源树,研究论文发展脉络
Chat Paper
正在生成论文摘要