Evolutionary Neural Architecture Search For Remaining Useful Life Prediction
APPLIED SOFT COMPUTING(2021)
摘要
With the advent of Industry 4.0, making accurate predictions of the remaining useful life (RUL) of industrial components has become a crucial aspect in predictive maintenance (PdM). To this aim, various Deep Neural Network (DNN) models have been proposed in the recent literature. However, while the architectures of these models have a large impact on their performance, they are usually determined empirically. To exclude the time-consuming process and the unnecessary computational cost of manually engineering these models, we present a Neural Architecture Search (NAS) technique based on an Evolutionary Algorithm (EA) applied to optimize the architecture of a DNN used to predict the RUL. The EA explores the combinatorial parameter space of a multi-head Convolutional Neural Network with Long Short Term Memory (CNN-LSTM) to search for the best architecture. In particular, our method requires minimum computational resources by making use of an early stopping policy and a history of the evaluated architectures. We dub the proposed method ENAS-PdM. To our knowledge, this is the first work where an EA-based NAS is used to optimize a CNN-LSTM architecture in the field of PdM. In our experiments, we use the well-established Commercial Modular Aero-Propulsion System Simulation (C-MAPSS) dataset from NASA. Compared to the current state-of-the-art, our method obtains better results in terms of two different metrics, RMSE and Score, when aggregating across all the C-MAPSS sub-datasets. Without aggregation, we achieve lower RMSE in 3 out of 4 sub-datasets. Our experimental results verify that the proposed method is a reliable tool for obtaining state-of-the-art RUL predictions and as such it can have a strong impact in several industrial applications, especially those with limited available computing power. (C) 2021 Elsevier B.V. All rights reserved.
更多查看译文
关键词
Evolutionary algorithm, Convolutional neural network, Long short term memory, Remaining useful life, C-MAPSS
AI 理解论文
溯源树
样例
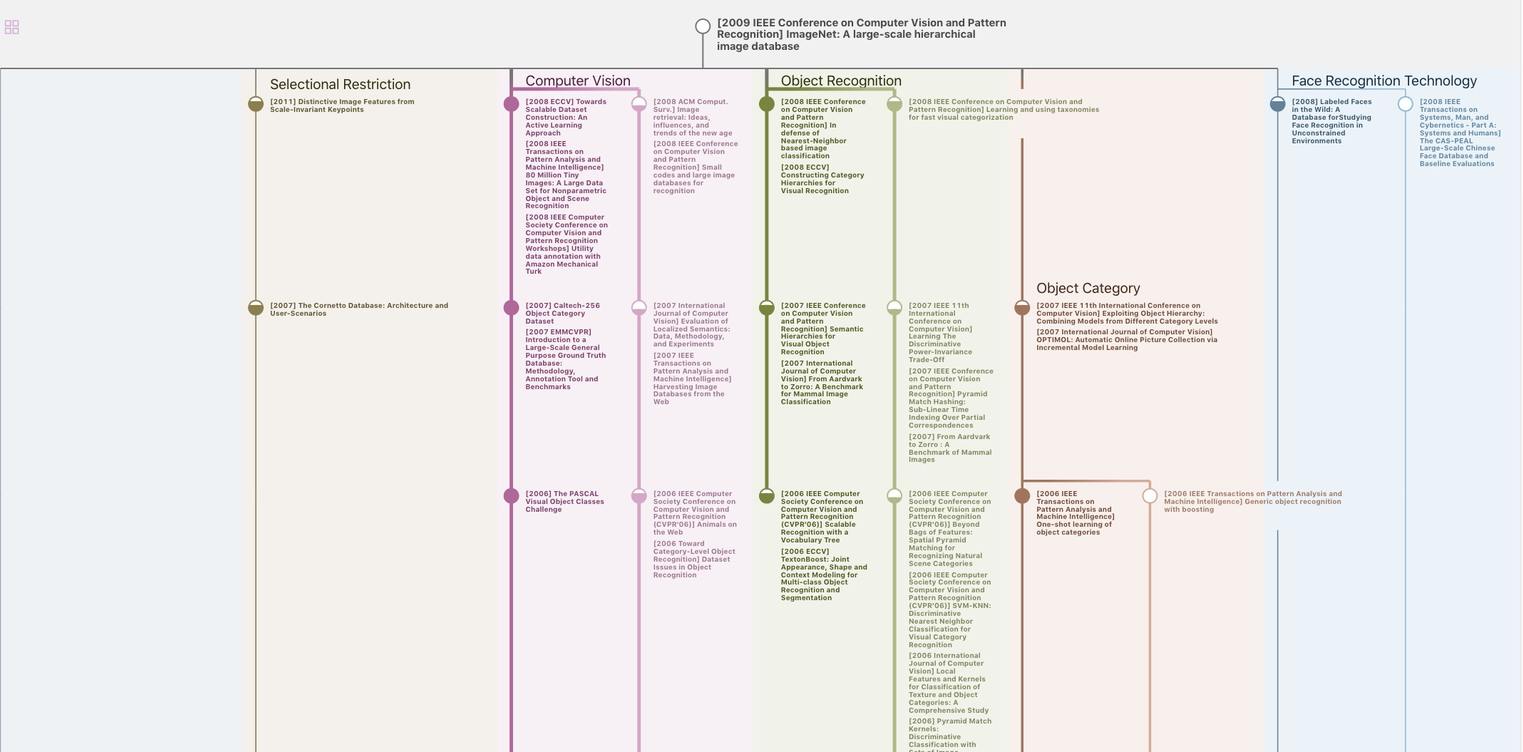
生成溯源树,研究论文发展脉络
Chat Paper
正在生成论文摘要