Soil organic carbon mapping in cultivated land using model ensemble methods
ARCHIVES OF AGRONOMY AND SOIL SCIENCE(2022)
摘要
The soil organic carbon (SOC) pool is larger than the biotic and atmospheric carbon pools, and understanding cultivated land SOC is critical for evaluating soil fertility and local agricultural management. Digital soil mapping (DSM) efficiently predicts soil properties by utilizing spatially distributed information. To date, many machine learning techniques have been applied in DSM, but model ensemble methods have rarely been used. In this study, two model ensemble methods, including the Granger-Ramanathan averaging and Bayesian model averaging, and three individual mathematical algorithms, such as the random forest, quantile regression forests, and multivariate adaptive regression splines models, were used to estimate SOC at a local level with limited soil samples. The prediction performances of the five methods were compared using an independent validation dataset composed of field observations of soil samples. The results showed that the prediction accuracy of the ensemble methods was higher than that of the individual methods (Delta R-2 = 0.02 to 0.21). Compared to the other methods, the Granger-Ramanathan method had a lower root mean square error (RMSE) and mean absolute error and a higher prediction uncertainty. Therefore, the results of this study show the effectiveness of using ensemble methods in mapping SOC with limited soil samples.
更多查看译文
关键词
Digital soil mapping, soil organic carbon, random forest, model ensemble
AI 理解论文
溯源树
样例
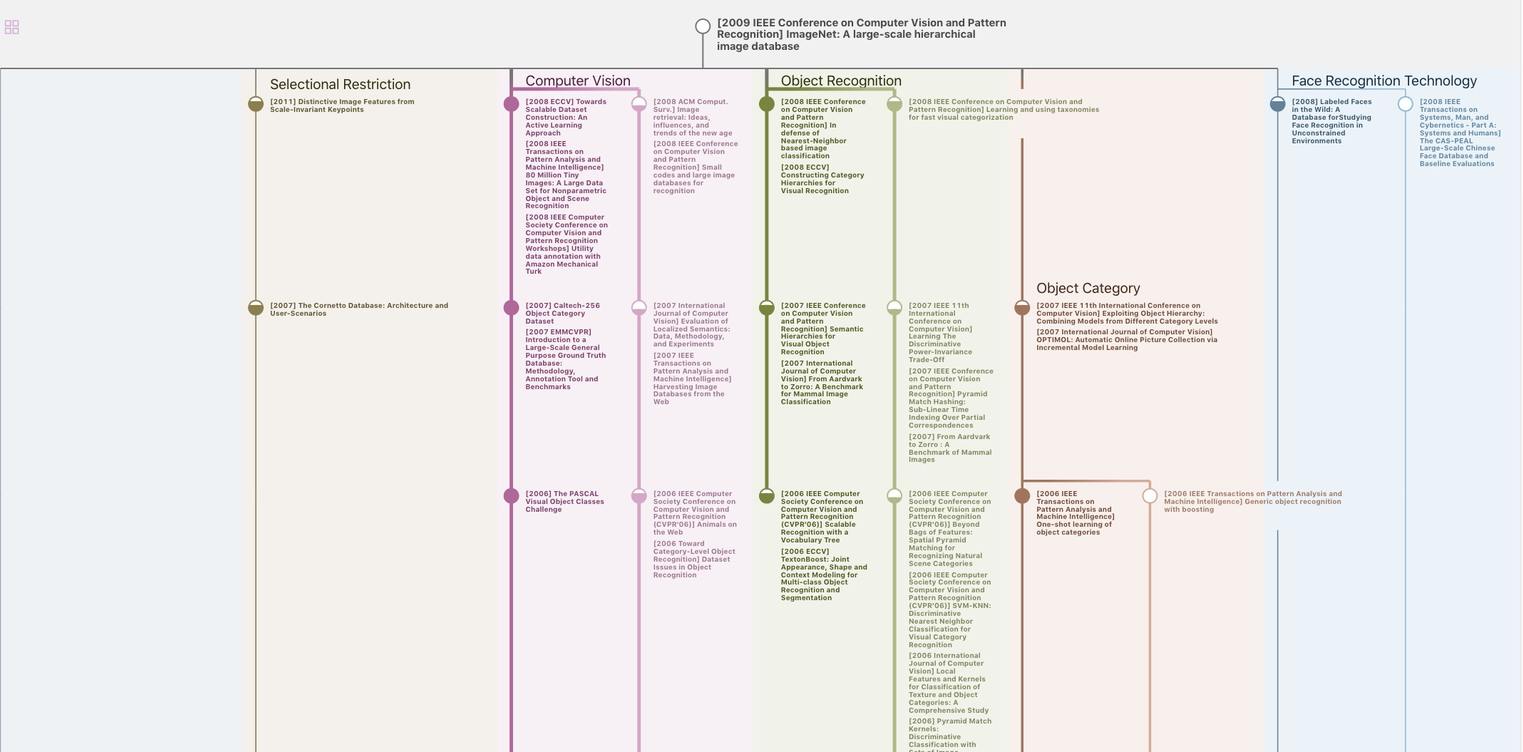
生成溯源树,研究论文发展脉络
Chat Paper
正在生成论文摘要