One Transistor One Electrolyte‐Gated Transistor Based Spiking Neural Network for Power‐Efficient Neuromorphic Computing System
Advanced Functional Materials(2021)
摘要
Neuromorphic computing powered by spiking neural networks (SNN) provides a powerful and efficient information processing paradigm. To harvest the advantage of SNNs, compact and low-power synapses that can reliably practice local learning rules are required, posing significant challenges to the conventional silicon-based platform in terms of area- and energy-efficiency, as well as computing throughput. Here, electrolyte-gated transistors (EGTs) paired with transistors are employed to implement power-efficient neuromorphic computing systems. The one-transistor-one-EGT (1T1E) synapse not only alleviates the self-discharging of EGT but also provides a flexible and efficient way to practice the important spike-timing-dependent plasticity learning rule. Based on that, an SNN with a temporal coding scheme is implemented for associative memory that can learn and recover images of handwritten digits with high robustness. Thanks to the temporal coding scheme and low operation current of EGTs, the energy-efficiency of 1T1E-based SNN is approximate to 30x lower than that of the prevalent rate coding scheme, and the peak performance is estimated to be 2 pJ/SOP (picojoule per synaptic operation) at the training phase and 80 TOPs(-1) W-1 (tera operations per second per watt) at inference phase, respectively. These results pave the way for power-efficient neuromorphic computing systems with wide applications for edge computing.
更多查看译文
关键词
associative memory, electrolyte‐, gated transistors, ion intercalation, neuromorphic computing, spiking neural networks
AI 理解论文
溯源树
样例
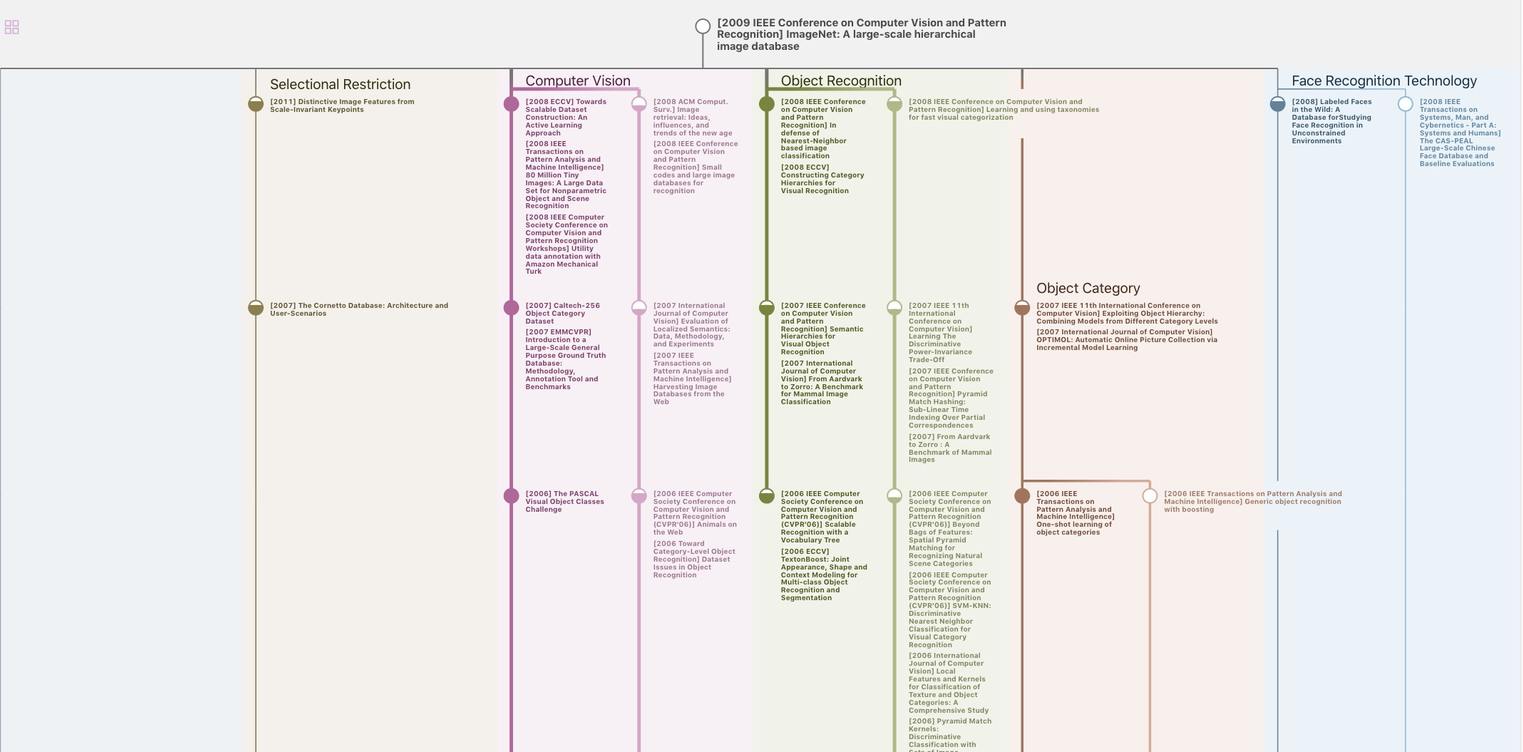
生成溯源树,研究论文发展脉络
Chat Paper
正在生成论文摘要