Fight Fire with Fire: Towards Robust Recommender Systems via Adversarial Poisoning Training
Research and Development in Information Retrieval(2021)
摘要
ABSTRACTRecent studies have shown that recommender systems are vulnerable, and it is easy for attackers to inject well-designed malicious profiles into the system, leading to biased recommendations. We cannot deny these data's rationality, making it imperative to establish a robust recommender system. Adversarial training has been extensively studied for robust recommendations. However, traditional adversarial training adds small perturbations to the parameters (inputs), which do not comply with the poisoning mechanism in the recommender system. Thus for the practical models that are very good at learning existing data, it does not perform well. To address the above limitations, we propose adversarial poisoning training (APT). It simulates the poisoning process by injecting fake users (ERM users) who are dedicated to minimizing empirical risk to build a robust system. Besides, to generate ERM users, we explore an approximation approach to estimate each fake user's influence on the empirical risk. Although the strategy of "fighting fire with fire" seems counterintuitive, we theoretically prove that the proposed APT can boost the upper bound of poisoning robustness. Also, we deliver the first theoretical proof that adversarial training holds a positive effect on enhancing recommendation robustness. Through extensive experiments with five poisoning attacks on four real-world datasets, the results show that the robustness improvement of APT significantly outperforms baselines. It is worth mentioning that APT also improves model generalization in most cases.
更多查看译文
关键词
Robust Recommender Systems, Adversarial Training, Poisoning Attacks
AI 理解论文
溯源树
样例
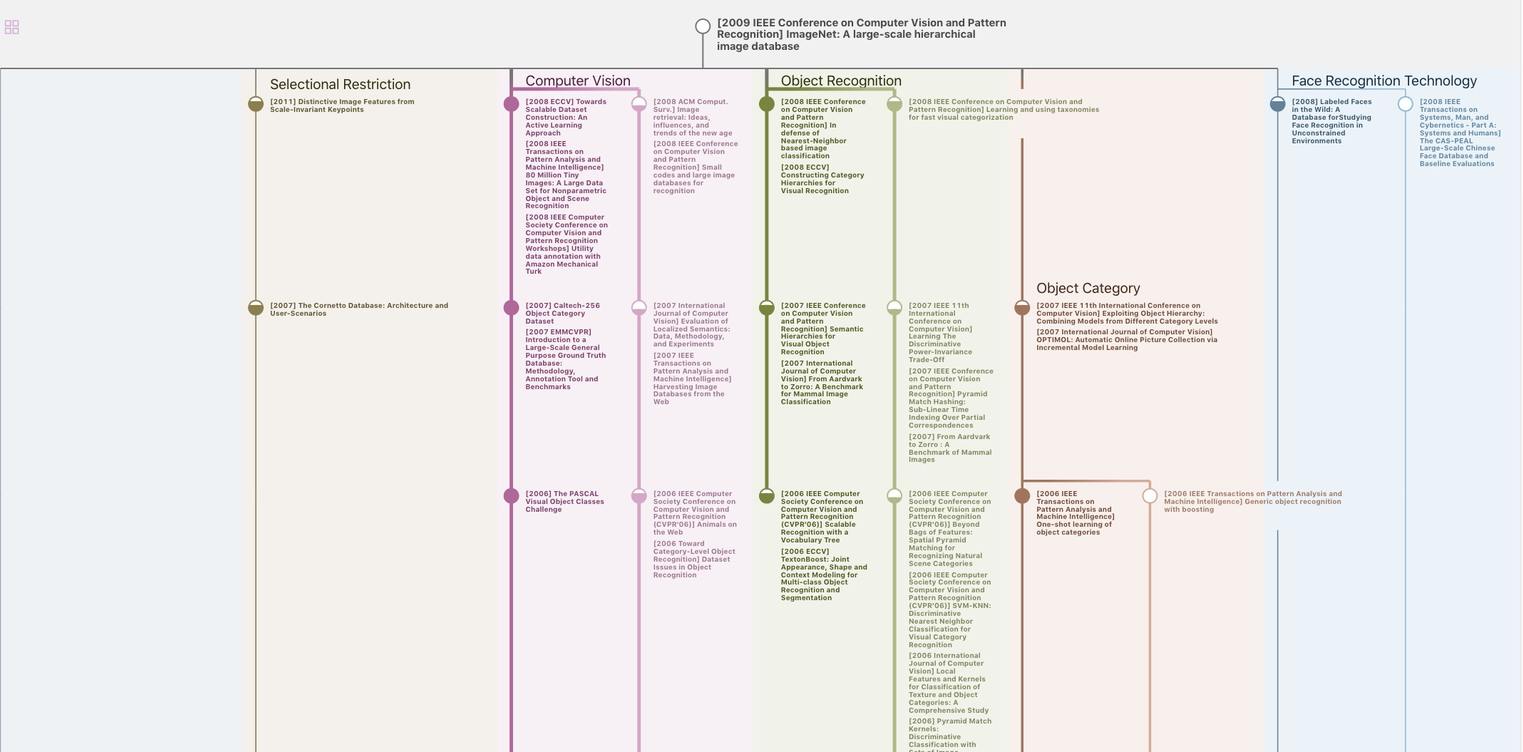
生成溯源树,研究论文发展脉络
Chat Paper
正在生成论文摘要