Underestimation Refinement: A General Enhancement Strategy for Exploration in Recommendation Systems
Research and Development in Information Retrieval(2021)
摘要
ABSTRACTClick-through rate (CTR) prediction based on deep neural networks has made significant progress in recommendation systems. However, these methods often suffer from CTR underestimation due to insufficient impressions for long-tail items. When formalizing CTR prediction as a contextual bandit problem, exploration methods provide a natural solution addressing this issue. In this paper, we first benchmark state-of-the-art exploration methods in the recommendation system setting. We find that the combination of gradient-based uncertainty modeling and Thompson Sampling achieves a significant advantage. On the basis of the benchmark, we further propose a general enhancement strategy, Underestimation Refinement (UR), which explicitly incorporates the prior knowledge that insufficient impressions likely leads to CTR underestimation. This strategy is applicable to almost all the existing exploration methods. Experimental results validate UR's effectiveness, achieving consistent improvement across all baseline exploration methods.
更多查看译文
关键词
Recommendation System, Contextual Bandit
AI 理解论文
溯源树
样例
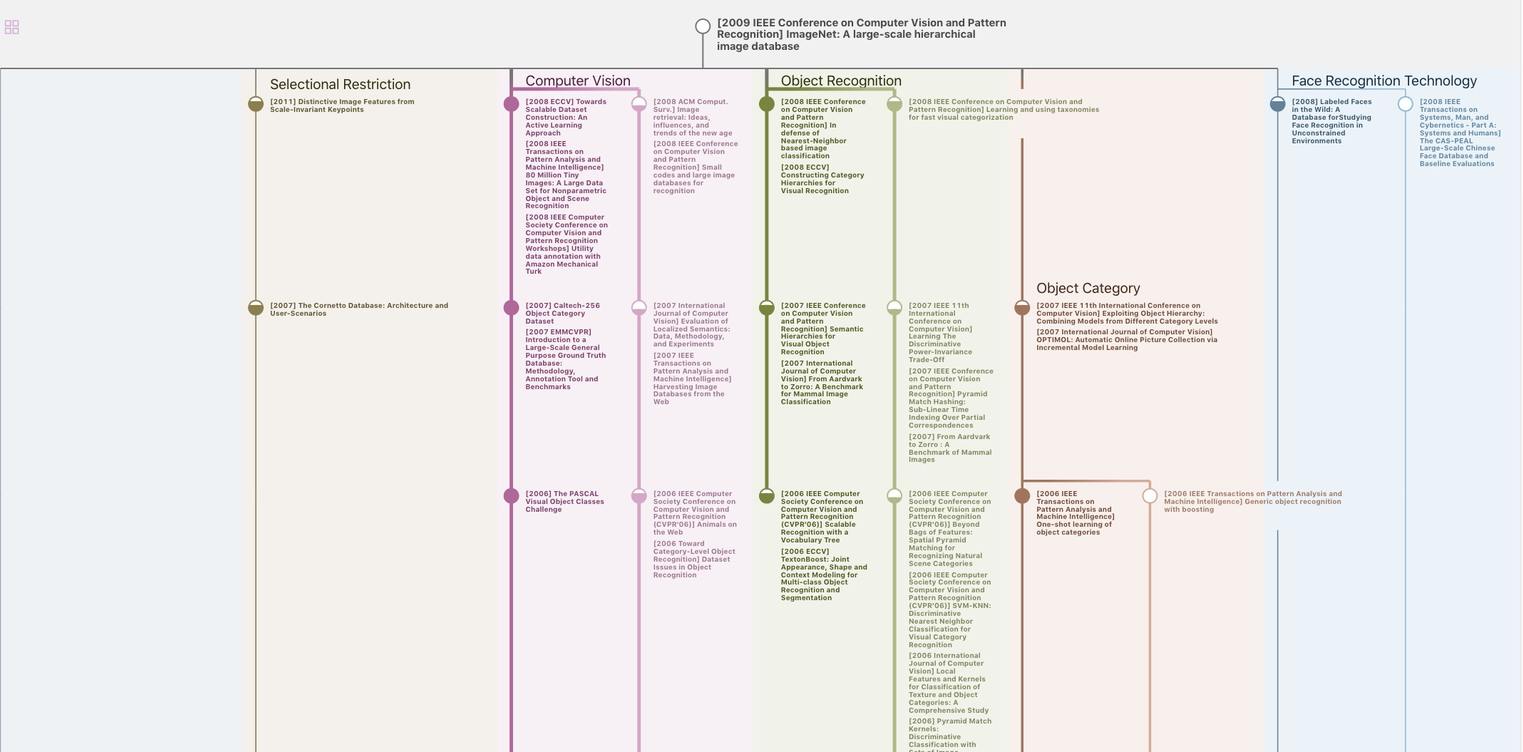
生成溯源树,研究论文发展脉络
Chat Paper
正在生成论文摘要