Learning to Select Instance: Simultaneous Transfer Learning and Clustering
Research and Development in Information Retrieval(2021)
摘要
ABSTRACTTransfer learning leverages knowledge from a source domain with rich data to a target domain with sparse data. However, the difference between the source and target data distribution weakens the transferability. To bridge this gap, we focus on selecting source instances that are closely related to and have the same distribution as the target domain. In this paper, we propose a novel Adaptive Clustering Transfer Learning (ACTL) method to improve transferability. Specifically, we simultaneously train the instance selector and the transfer learning model. The selector adaptively conducts clustering on the training data and learns the weights for source instances. The weight will activate or inhibit the contribution of the corresponding source instance during transfer learning. Meanwhile, the transfer learning model guides the selector to learn the weight appropriately according to the objective function. To evaluate the effectiveness of our method, we conduct experiments on two different tasks including recommender system and text matching. Experimental results show that our method consistently outperforms competing methods and the selected source instances share a similar data distribution with the target domain.
更多查看译文
关键词
transfer learning, instance selection, clustering
AI 理解论文
溯源树
样例
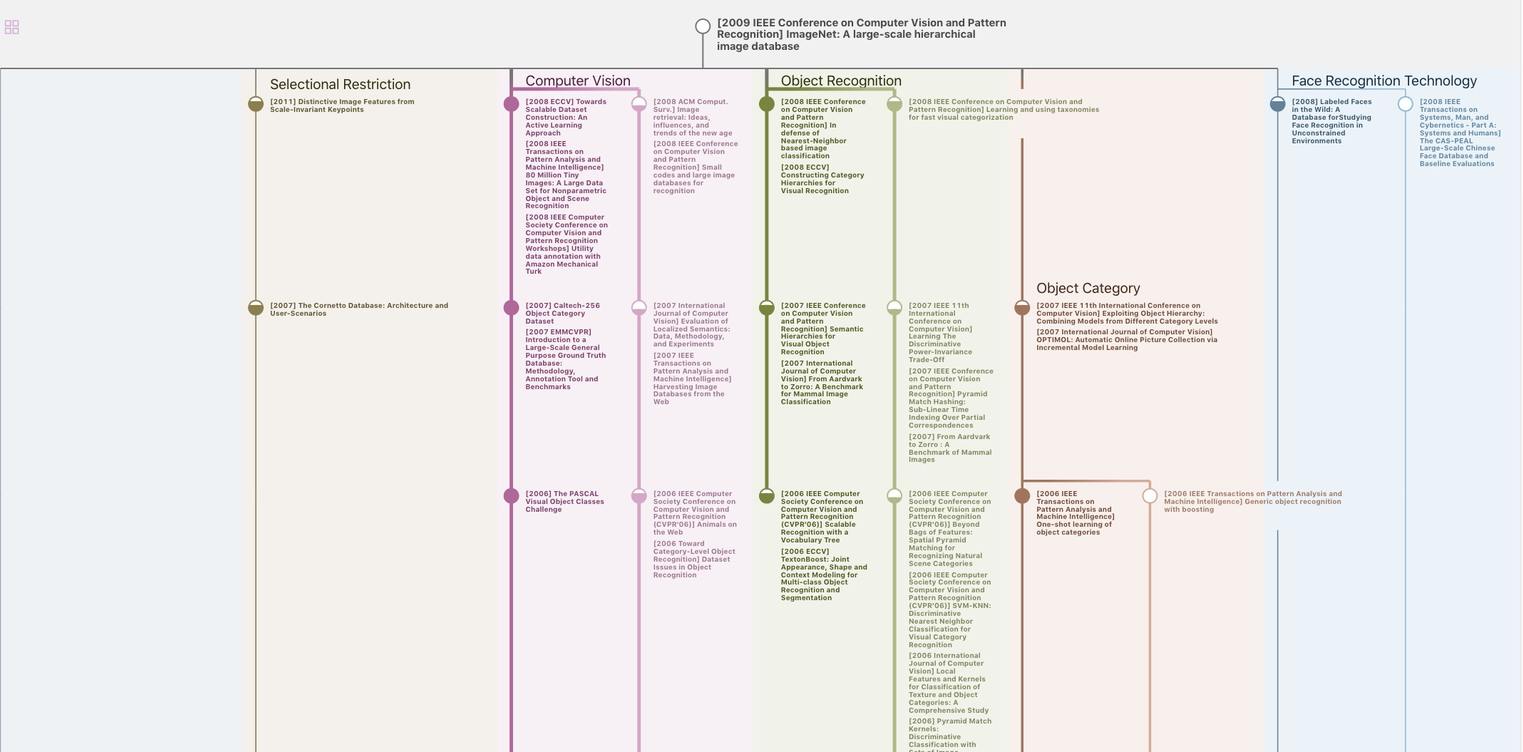
生成溯源树,研究论文发展脉络
Chat Paper
正在生成论文摘要