Self-distillation for German and Dutch dependency parsing
computational linguistics in the netherlands(2020)
摘要
In this paper, we explore self-distillation as a means to improve statistical dependency parsing models for Dutch and German over purely supervised training. Self-distillation (Furlanello et al. 2018) trains a new student model on the output of an existing (weaker) teacher model. In contrast to most previous work on self-distillation, we perform distillation using a large, unannotated corpus. We show that in dependency parsing as sequence labeling (Spoustov´a and Spousta 2010, Strzyz et al. 2019), self-distillation plus finetuning provides large improvements over models that use supervised training. We carry out experiments on the German T¨uBa-D/Z universal dependency (UD) treebank (C¸ ¨oltekin et al. 2017) and the UD conversion of the Dutch Lassy Small treebank (Bouma and van Noord 2017). We find that self-distillation improves German parsing accuracy of a bidirectional LSTM parser from 92.23 to 94.33 Labeled Attachment Score (LAS). Similarly, on Dutch we see improvement from 89.89 to 91.84 LAS.
更多查看译文
AI 理解论文
溯源树
样例
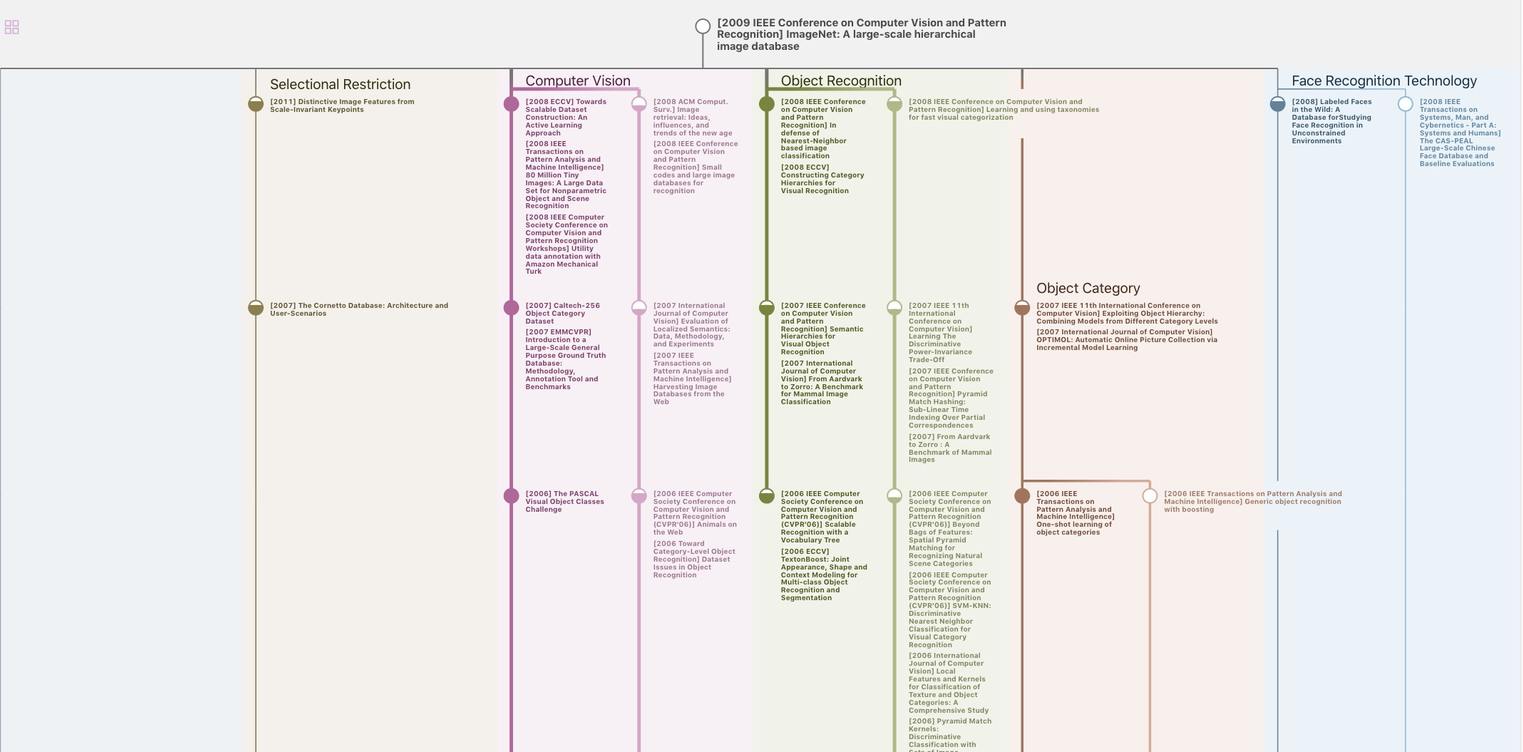
生成溯源树,研究论文发展脉络
Chat Paper
正在生成论文摘要