Predicting Tropical Cyclogenesis Using a Deep Learning Method From Gridded Satellite and ERA5 Reanalysis Data in the Western North Pacific Basin
IEEE Transactions on Geoscience and Remote Sensing(2022)
摘要
This article proposes a deep learning model to predict tropical cyclogenesis (TCG) from gridded satellite and ERA5 reanalysis data in the western North Pacific basin. The proposed model contains two modules. First, convolutional neural network (CNN)-based deep features are extracted for each predictor, and then, the extracted features are fused with two fully connected layers to differentiate and investigate the relationship between predictors and TCG. The experimental data of this study are composed of 3232 developing tropical cluster clouds and 6657 nondeveloping ones; 90% of the collected data are utilized to train the model, and the rest are used to evaluate the trained model. Totally, nine predictors have been considered for the study, and the results show that the brightness temperature (IR), relative vorticity (Vo), and geopotential height (Z) perform better than the other predictors. A combined model with six predictors [IR, Z, RH (relative humidity), Vo, WS
10 m
(wind speed at the height of ten meters above the surface of the Earth), and mslp (mean sea-level pressure)] achieves the best TCG predicting performance, i.e., 97.1% of developing tropical cyclones are detected at a probability threshold of 0.13 with a false alarm rate of 20.3%. The experimental results demonstrate that the proposed method is superior to the existing methods and also indicate that the fusion of satellite and reanalysis data is a promising method to predict TCG.
更多查看译文
关键词
Convolutional neural network (CNN),fusion of satellite images and ERA5 reanalysis data,tropical cyclogenesis (TCG) prediction
AI 理解论文
溯源树
样例
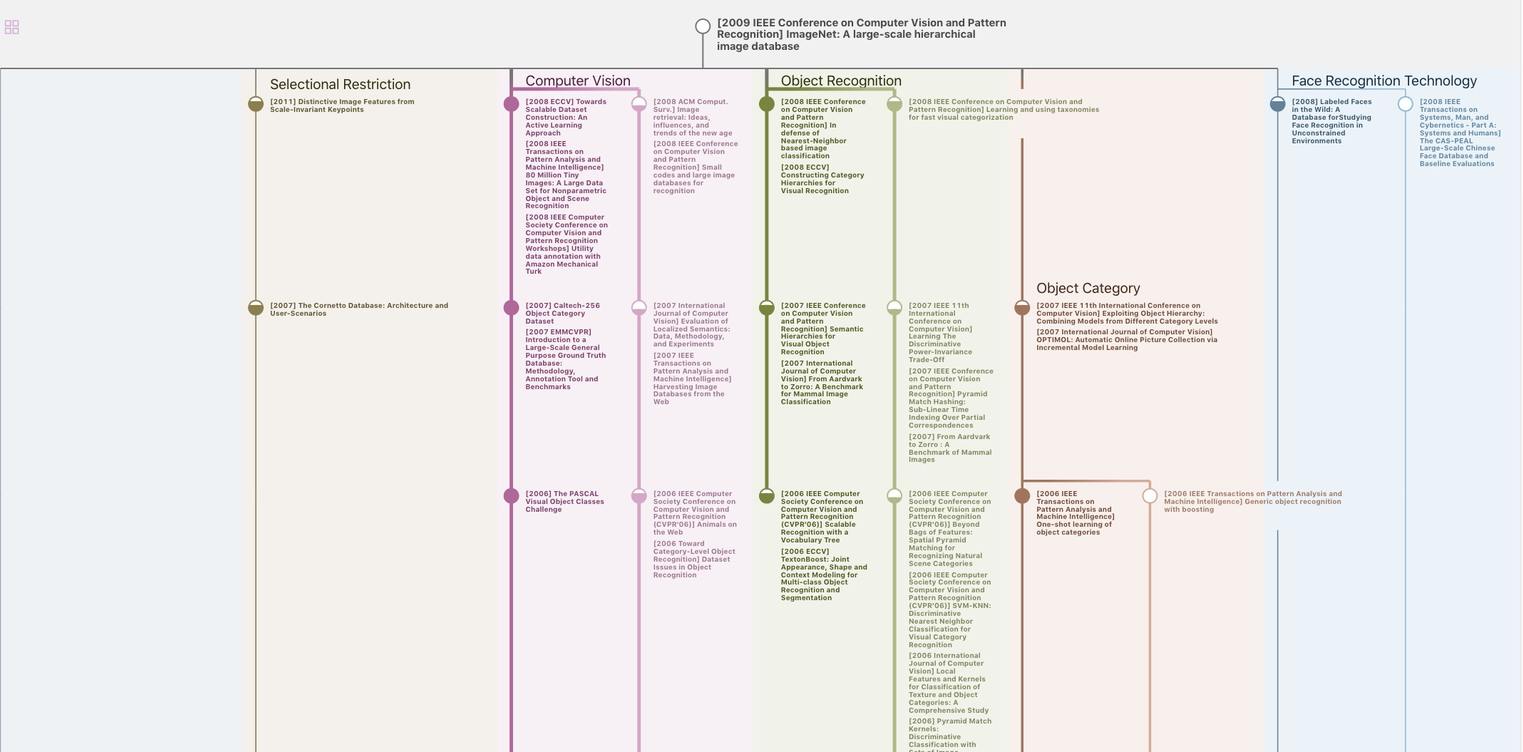
生成溯源树,研究论文发展脉络
Chat Paper
正在生成论文摘要