A GAPSO-Enhanced Extreme Learning Machine Method for Tool Wear Estimation in Milling Processes Based on Vibration Signals
INTERNATIONAL JOURNAL OF PRECISION ENGINEERING AND MANUFACTURING-GREEN TECHNOLOGY(2021)
摘要
Tools are the most vulnerable components in milling processes conducted using numerical control milling machines, and their wear condition directly influences work-product quality and operational safety. As such, tool wear estimation is an essential component of NC milling operations. This study addresses this issue by proposing an extreme learning machine (ELM) method enhanced by a hybrid genetic algorithm and particle swarm optimization (GAPSO) approach for conducting tool wear estimation based on workpiece vibration signals. Here, a few feature parameters in the time, frequency, and time–frequency (Ensemble empirical mode decomposition, EEMD) domains of the workpiece vibration signals are extracted as the input of the ELM model. Then, the initialized weights and thresholds of the ELM model are optimized based on the GAPSO approach with training dataset. Finally, tool wear is estimated using the optimized ELM model with testing dataset. The effectiveness of the proposed method is verified by its application to vibration signals collected from two milling tool wear experiments (an open-access benchmark dataset and a milling tool wear experiment) by comparison to the ELM, GA-ELM, and PSO-ELM methods. The results indicate that the estimation accuracy and optimization efficiency of the proposed method outperforms that of other three methods.
更多查看译文
关键词
Milling tool wear estimation, Extreme learning machine, Genetic algorithm, Particle swarm optimization
AI 理解论文
溯源树
样例
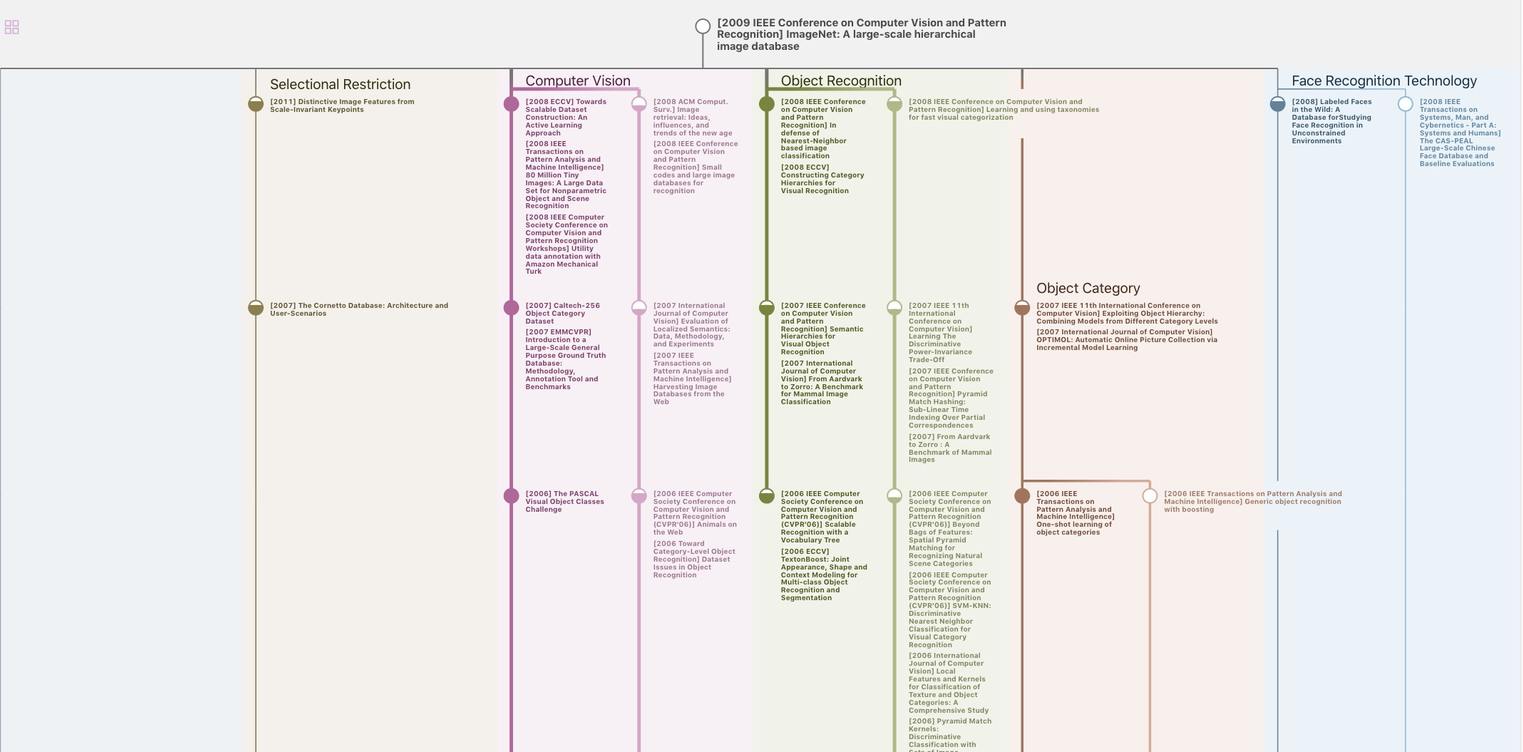
生成溯源树,研究论文发展脉络
Chat Paper
正在生成论文摘要