A novel self-attention deep subspace clustering
INTERNATIONAL JOURNAL OF MACHINE LEARNING AND CYBERNETICS(2021)
摘要
Most of the existing deep subspace clustering methods leverage convolutional autoencoders to obtain feature representation for non-linear data points. These methods commonly adopt the structure of a few convolutional layers because stacking many convolutional layers may cause computationally inefficient and optimization difficulties. However, long-range dependencies can hardly be captured when convolutional operations are not repeated enough, thus affect the quality of feature extraction which the performance of deep subspace clustering method highly lies in. To deal with this issue, we propose a novel self-attention deep subspace clustering (SADSC) model, which learns more favorable data representations by introducing self-attention mechanisms into convolutional autoencoders. Specifically, SADSC leverages three convolutional layers and add the self-attention layers after the first and third ones in encoders, then decoders have symmetric structures. The self-attention layers maintain the variable input sizes and can be easily combined with different convolutional layers in autoencoder. Experimental results on the handwritten recognition, face and object clustering datasets demonstrate the advantages of SADSC over the state-of-the-art deep subspace clustering models.
更多查看译文
关键词
Deep subspace clustering,Convolutional autoencoder,Self-attention
AI 理解论文
溯源树
样例
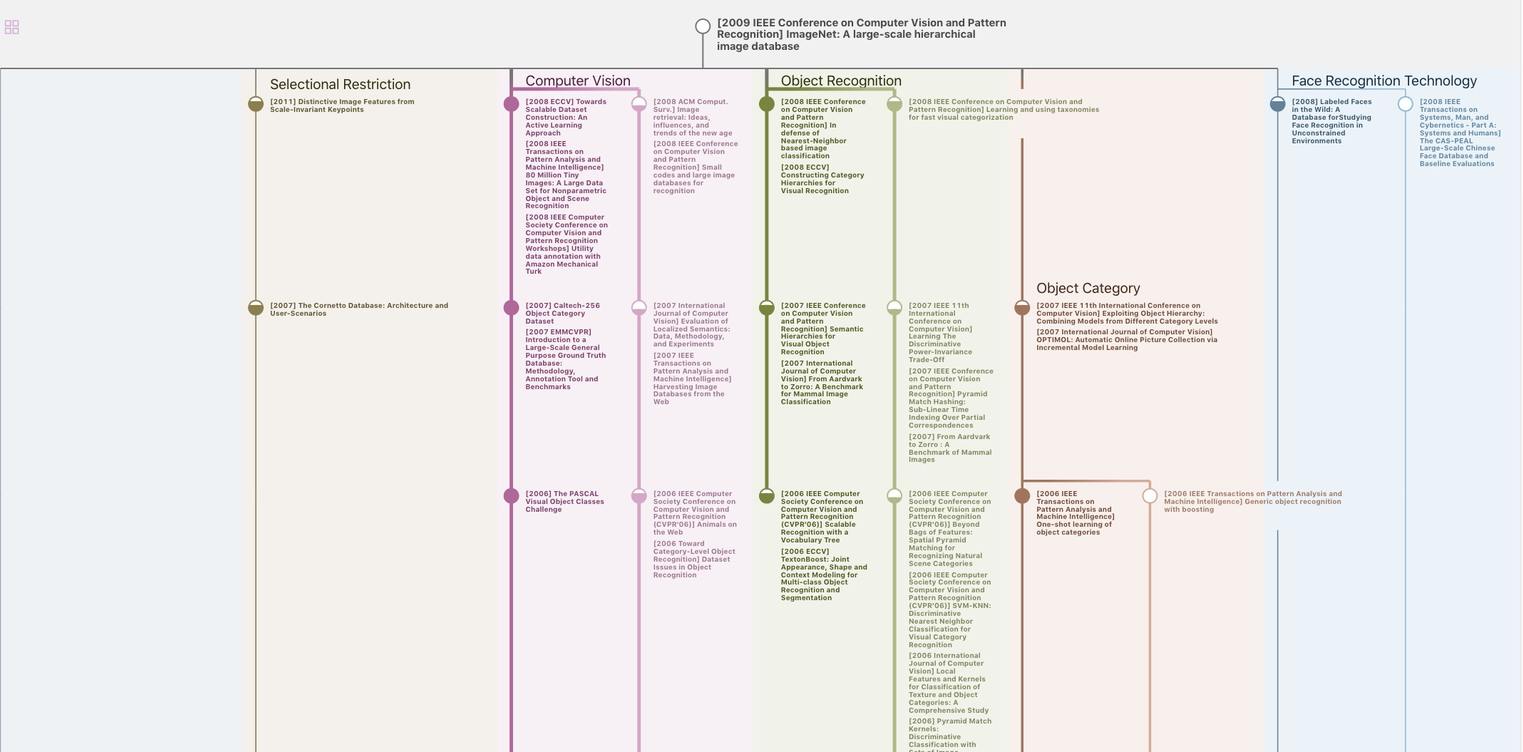
生成溯源树,研究论文发展脉络
Chat Paper
正在生成论文摘要