Total Variation Regularized Weighted Tensor Ring Decomposition for Missing Data Recovery in High-Dimensional Optical Remote Sensing Images
IEEE GEOSCIENCE AND REMOTE SENSING LETTERS(2022)
摘要
Due to sensor malfunction and atmosphere disturbances, high-dimensional optical remote sensing (HORS) images often suffer from information missing, such as dead pixels and thick clouds. Tensor decomposition methods have been used to estimate the missing data of HORS images. However, most existing models hardly consider the inherent properties and effective structural information of HORS images. To this end, we propose a novel total variation (TV) regularized weighted tensor ring (TR) decomposition model to recover the missing content of HORS images. The TR decomposition has the powerful low-rank (LR) representation ability to recover the HORS data by employing three low-dimensional tensors, i.e., TR factors. An initialization step and proper weights are designed to enhance the flexibility for exploring different LR properties of TR factors. To further preserve the spatial smoothness, the local spatial TV from three directions is incorporated into the TR decomposition framework. Furthermore, an augmented Lagrange multiplier (ALM) algorithm is designed for solving the resulting optimization problem. Experiments on HORS images demonstrate the performances of the proposed method over the current state-of-the-art baselines.
更多查看译文
关键词
Tensors, TV, Optical sensors, Optical imaging, Optimization, Matrix decomposition, Image reconstruction, Augmented Lagrange multiplier (ALM), high-dimensional optical remote sensing (HORS), low-rank (LR) property, tensor ring (TR) decomposition, total variation (TV) from three directions, TR factors initialization, weights
AI 理解论文
溯源树
样例
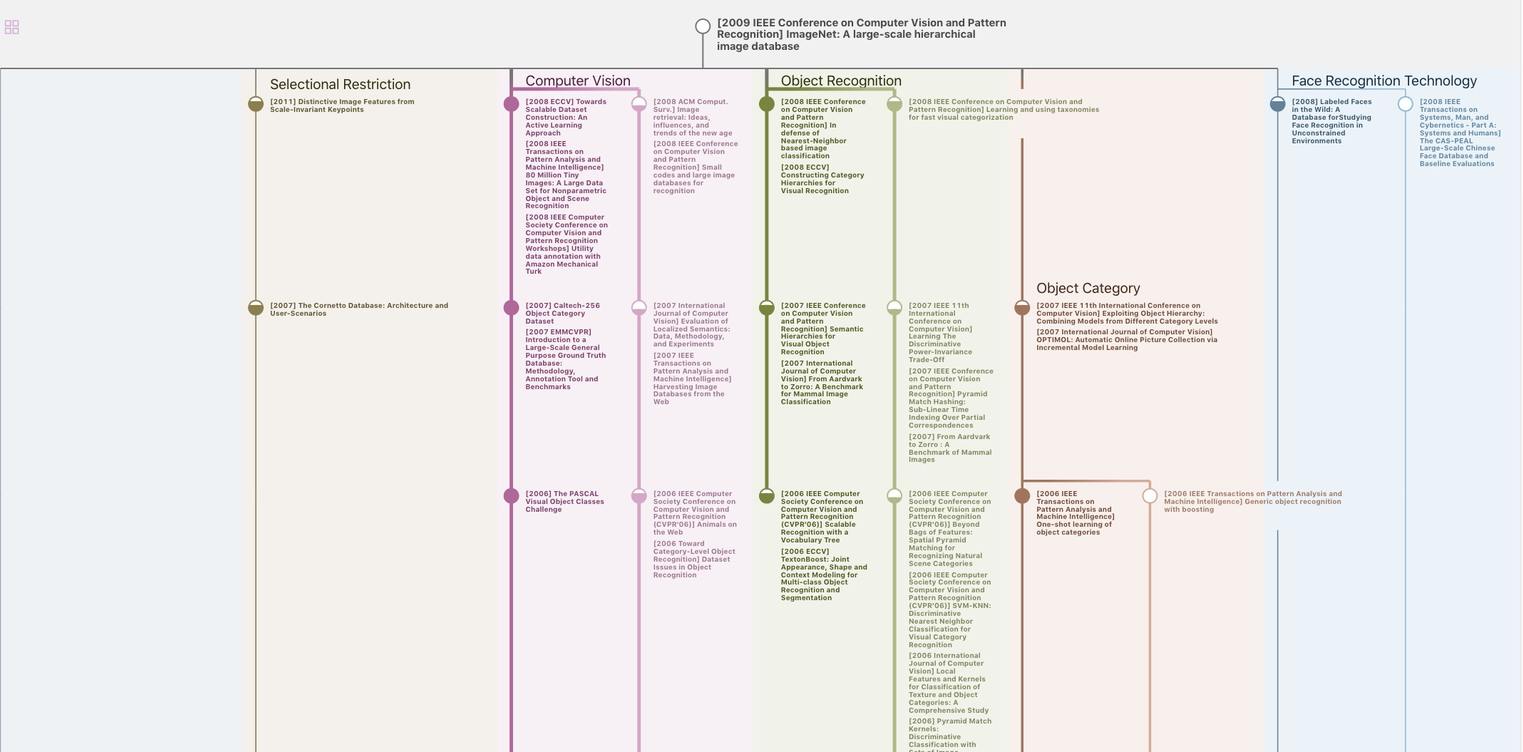
生成溯源树,研究论文发展脉络
Chat Paper
正在生成论文摘要