AUV Surfacing Control With Adversarial Attack Against DLaaS Framework
IEEE TRANSACTIONS ON COMPUTERS(2024)
摘要
In this study, we consider surfacing control problems for the autonomous underwater vehicle in three-dimensional space under emergencies. Most model-based controllers cannot effectively solve these problems due to the unknown task environment model. Moreover, existing deep reinforcement learning (DRL) methods have some limitations, such as slow convergence or overestimation bias. For these purposes, we propose a model-free DRL algorithm based on the Deep Deterministic Policy Gradient within the paradigm of deep learning as a service (DLaaS). The algorithm combines existing expert episodes as demonstration and transitions from the interaction between the agent and task environment, which potentially reduce risk from adversarial attack. We propose a variable delay learning mechanism to improve the performance of the proposed algorithm. The simulation results show that our method can converge faster and has a more robust performance with adversarial attack than the existing DRL method under the premise of solving the AUV surfacing control tasks for safety.
更多查看译文
关键词
AUV surfacing control,deep reinforcement learning,learning from demonstration,delay learning
AI 理解论文
溯源树
样例
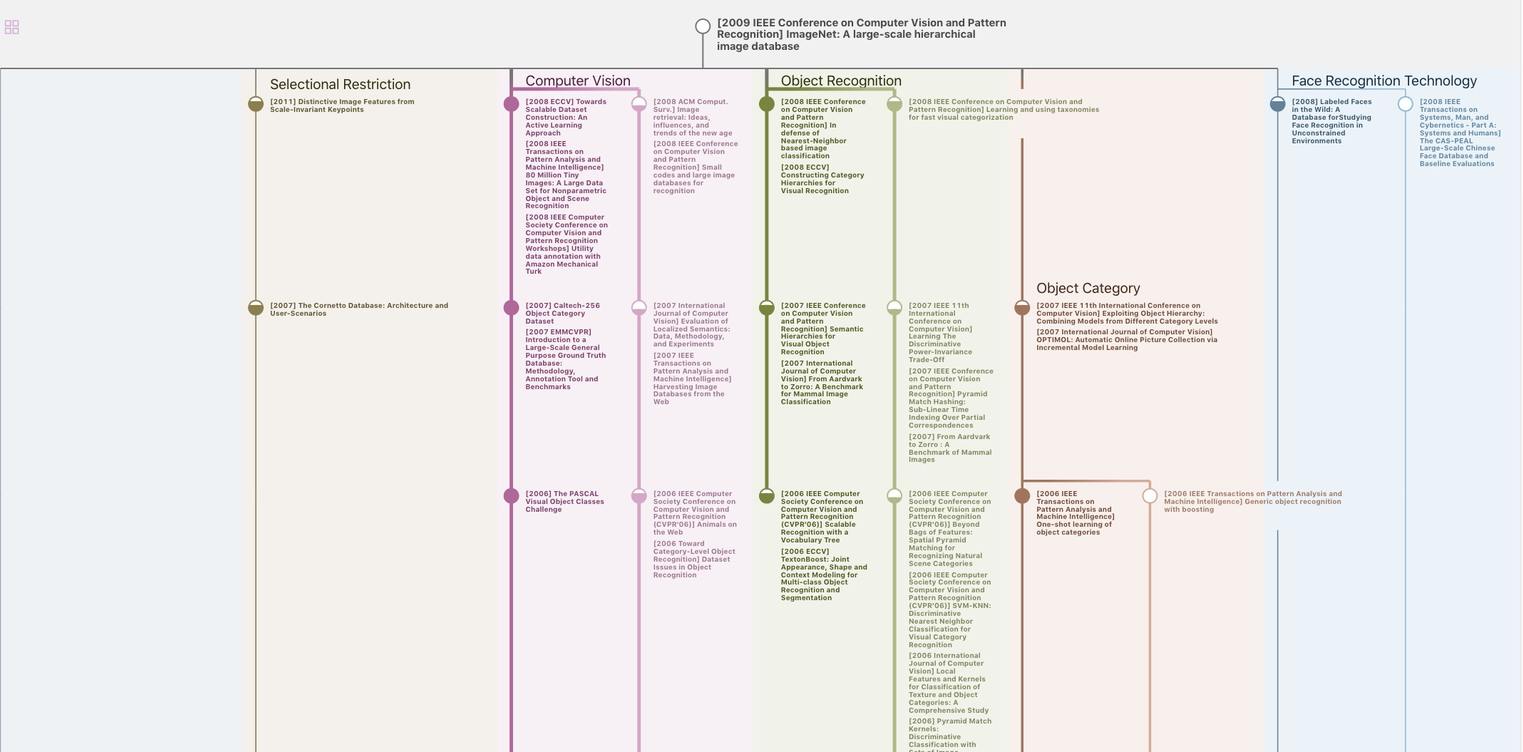
生成溯源树,研究论文发展脉络
Chat Paper
正在生成论文摘要