Single-scale siamese network based RGB-D object tracking with adaptive bounding boxes
Neurocomputing(2021)
摘要
Most of the excellent methods for visual object tracking are based on RGB videos. With the popularity of depth cameras, the research on RGB-D(RGB+depth) tracking has gradually gained extensive attention. The depth map provides more available information for dealing with intractable tracking problems. How to make full use of depth maps to construct a better tracker is the foremost problem to be settled. The fully-convolutional siamese network shows excellent performance in 2D tracking, but still cannot achieve satisfying tracking performance in complex scenarios. Therefore, we have proposed the RGB-D tracker integrated with the single-scale siamese network as well as the adaptive bounding boxes, which achieves stable tracking performance under the challenges such as occlusion, scale variation and background clutter. Our proposed adaptive strategy enables the bounding box to adjust automatically when the target appearance changes during the tracking, instead of multi-scale input in the siamese network. We design an effective algorithm to quickly obtain the target depth and construct the 3D local visual field to eliminate the interference from background and similar objects. In addition, the total occlusion handling approach combined with RGB and depth information has achieved more reliable occlusion detection and target recovery. Our presented object tracker, including the strategies of 3D local visual field, adaptive bounding boxes and occlusion handling, has been evaluated on two widely utilized RGB-D tracking benchmarks and achieves suprior performance especially for the situations of occlusion and pedestrian detection.
更多查看译文
关键词
Visual object tracking,RGB-D videos,Siamese network,Occlusion handling,Adaptive strategy
AI 理解论文
溯源树
样例
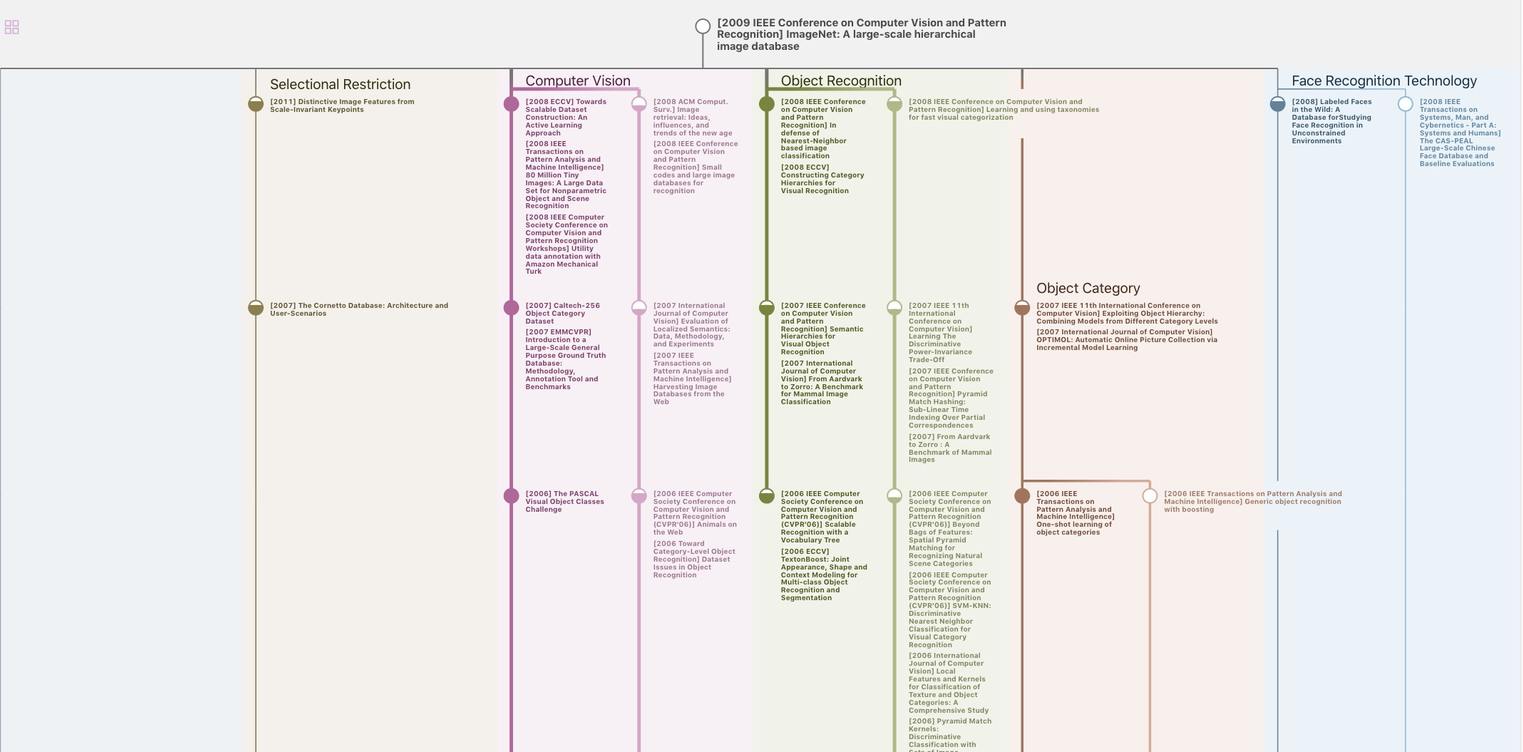
生成溯源树,研究论文发展脉络
Chat Paper
正在生成论文摘要