Sparsity-Enhanced Convolutional Decomposition: A Novel Tensor-Based Paradigm for Blind Hyperspectral Unmixing
IEEE TRANSACTIONS ON GEOSCIENCE AND REMOTE SENSING(2022)
摘要
Blind hyperspectral unmixing (HU) has long been recognized as a crucial component in analyzing the hyperspectral imagery (HSI) collected by airborne and spaceborne sensors. Due to the highly ill-posed problems of such a blind source separation scheme and the effects of spectral variability in hyperspectral imaging, the ability to accurately and effectively unmixing the complex HSI still remains limited. To this end, this article presents a novel blind HU model, called sparsity-enhanced convolutional decomposition (SeCoDe), by jointly capturing spatial-spectral information of HSI in a tensor-based fashion. SeCoDe benefits from two perspectives. On the one hand, the convolutional operation is employed in SeCoDe to locally model the spatial relation between the targeted pixel and its neighbors, which can be well explained by spectral bundles that are capable of addressing spectral variabilities effectively. It maintains, on the other hand, physically continuous spectral components by decomposing the HSI along with the spectral domain. With sparsity-enhanced regularization, an alternative optimization strategy with alternating direction method of multipliers (ADMM)-based optimization algorithm is devised for efficient model inference. Extensive experiments conducted on three different data sets demonstrate the superiority of the proposed SeCoDe compared to previous state-of-the-art methods. We will also release the code at https://github.com/danfenghong/IEEE_TGRS_SeCoDe to encourage the reproduction of the given results.
更多查看译文
关键词
Tensors, Hyperspectral imaging, Convolutional codes, Task analysis, Optimization, Encoding, Context modeling, Blind hyperspectral unmixing (HU), convolutional sparse coding (CSC), spectral bundles, spectral variability (SV), tensor decomposition
AI 理解论文
溯源树
样例
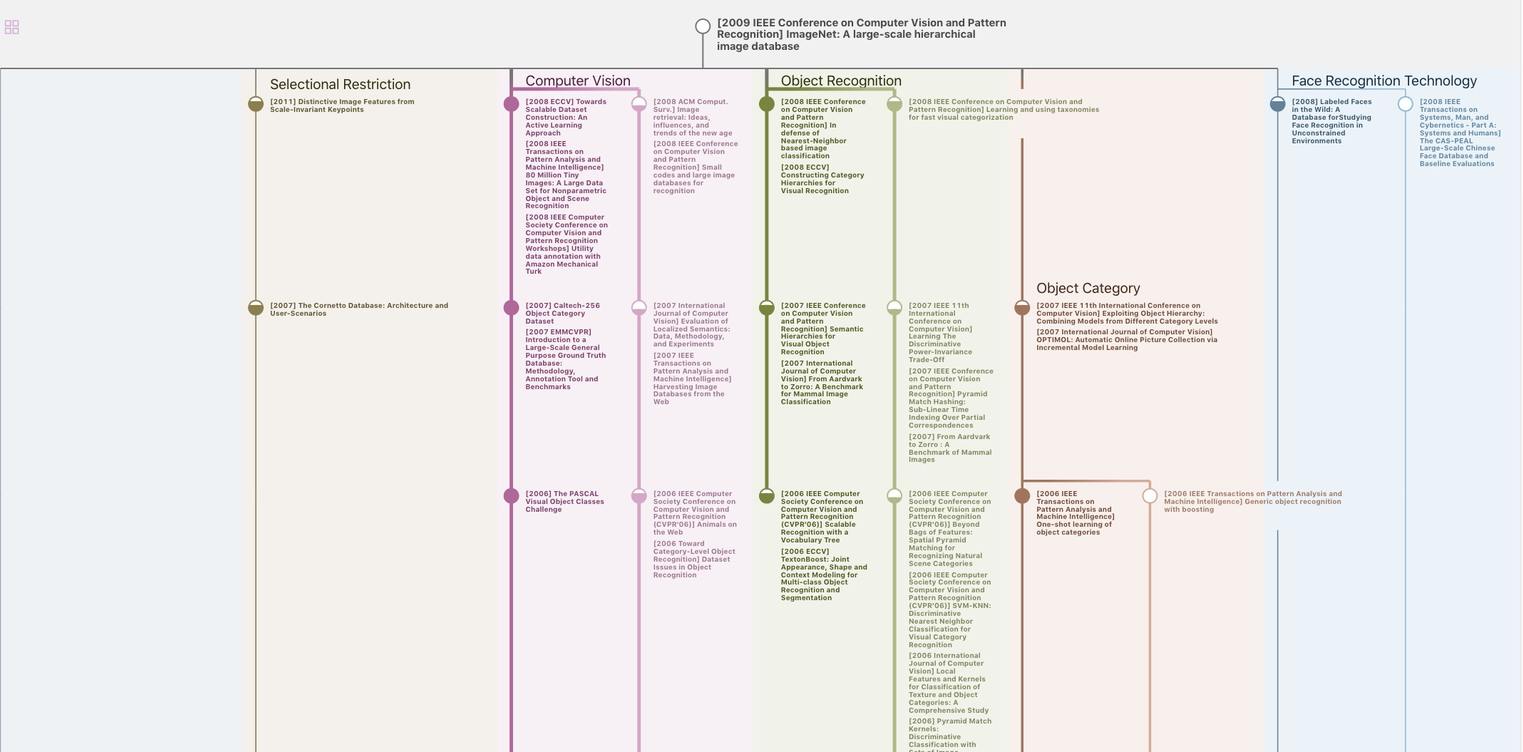
生成溯源树,研究论文发展脉络
Chat Paper
正在生成论文摘要