Food tray sealing fault detection using hyperspectral imaging and PCANet
IFAC-PapersOnLine(2020)
摘要
Abstract Food trays are very common in shops and supermarkets. Fresh food packaged in trays must be correctly sealed to protect the internal atmosphere and avoid contamination or deterioration. Due to the speed of production, it is not possible to have human quality inspection. Thus, automatic fault detection is a must to reach high production volume. This work describes a deep neural network based on Principal Component Analysis Network (PCANet) for food tray sealing fault detection. The input data come from hyperspectral cameras, showing more characteristics than regular industrial cameras or the human eye as they capture the spectral properties for each pixel. The proposed classification algorithm is divided into three main parts. In the first part, a single image is extracted from the hypercube by using pixel-level fusion method: the cube hyperspectral images are transformed into two-dimensional images to use as the input to the PCANet. Second, a PCANet structure is applied to the fused image. The PCANet has two filter bank layers and one binarization layer (three stages), obtaining a feature vector. Finally, a classification algorithm is used, having the feature vector as input data. The SVM and KNN classifiers were used. The database used in this work is provided by food industry professionals, containing eleven types of contamination in the seal area of the food tray and using metallic opaque cover film. Obtained results show that the design of our framework proposed achieves accuracy of 90% (87% F-measure) and 89% (89% F-measure) for SVM and KNN, respectively. Computation time for classification shows that a food tray speed of 65 trays per second could be reached. As a final result, the influence of the dataset size is analyzed, having PCANet a similar behavior for an extended and a reduced dataset.
更多查看译文
关键词
Image processing, PCANet Classifier, Neural networks, Food Industry, Food packaging, quality inspection
AI 理解论文
溯源树
样例
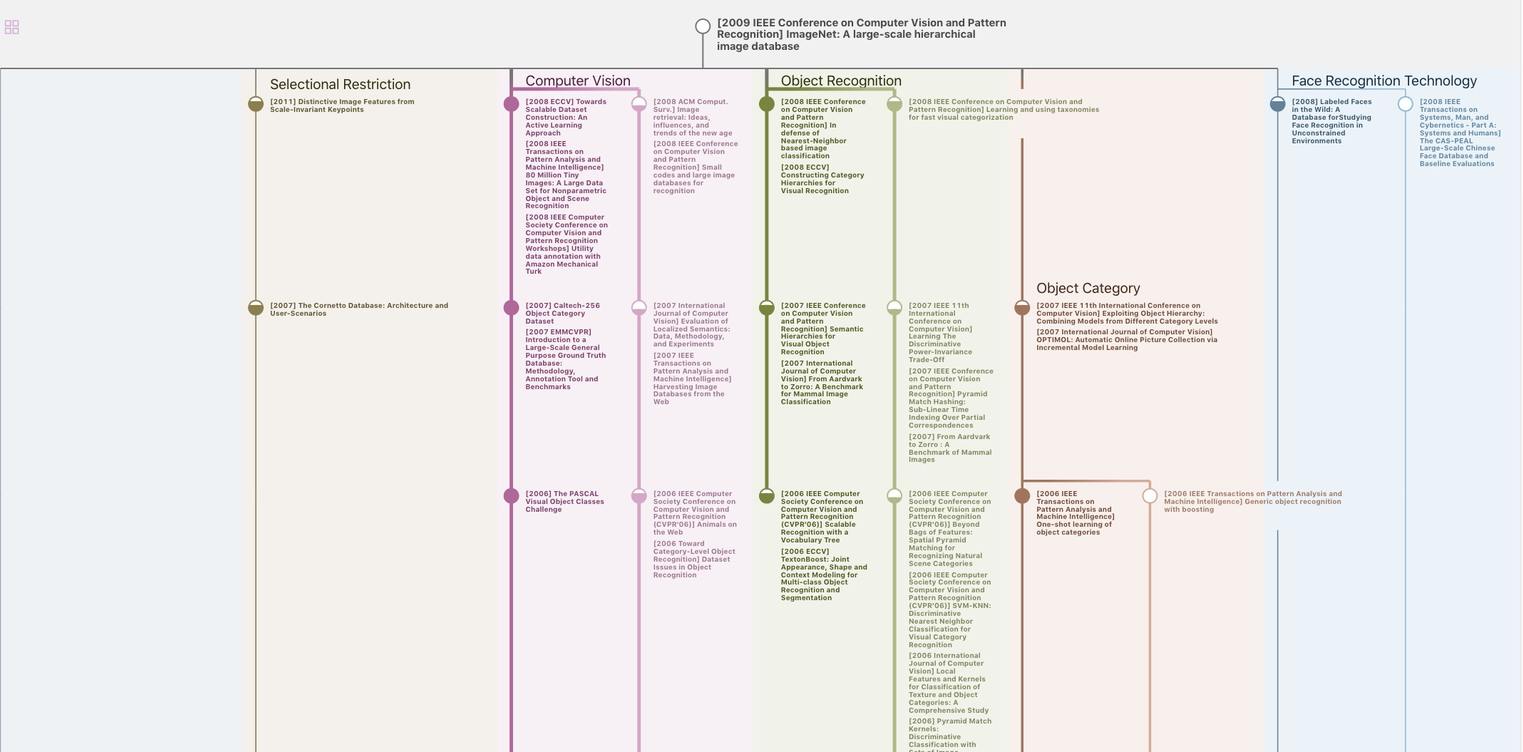
生成溯源树,研究论文发展脉络
Chat Paper
正在生成论文摘要