Novel Three-Stage Feature Fusion Method of Multimodal Data for Bearing Fault Diagnosis
IEEE TRANSACTIONS ON INSTRUMENTATION AND MEASUREMENT(2021)
摘要
Bearing faults are among the most common causes of machine failures. Therefore, bearing fault diagnosis should be performed reliably and rapidly. Currently, many types of modal data for monitoring the running state of bearings are available. They include data from different kinds of sensors and various domains (such as time and frequency domains). However, obtaining fault features with high quality from single-modal data is difficult because of the complex working conditions. Therefore, extracting and integrating complementary fault features from multimodal monitoring data are problems that remain to be solved. To address these issues, this study considers vibration and torque signals and proposes a novel three-stage feature fusion method of multimodal data for the fault diagnosis of bearings. The method is called attention-based multidimensional concatenated convolutional neural network. The attention mechanism can learn global information and assign different weights to feature maps to highlight important features. The fused features are then used in fault classification via softmax regression. The effectiveness of the proposed method is verified through the Paderborn data set. Results show that diagnostic accuracy is significantly improved from 96.4% to 99.8%.
更多查看译文
关键词
Attention mechanism, bearing faults, complementary fault features, fault diagnosis, feature fusion
AI 理解论文
溯源树
样例
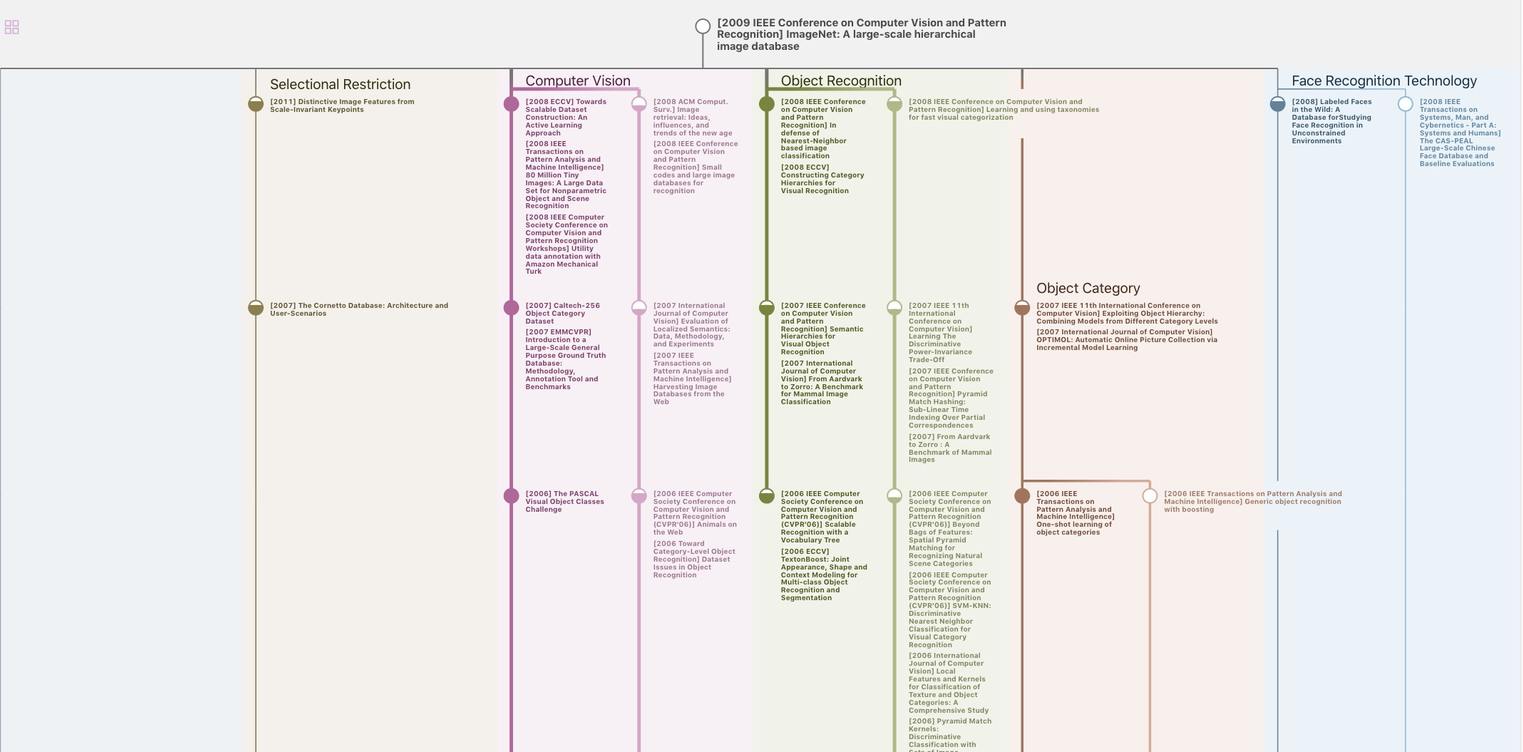
生成溯源树,研究论文发展脉络
Chat Paper
正在生成论文摘要