Estimation Of Root Zone Soil Moisture From Ground And Remotely Sensed Soil Information With Multisensor Data Fusion And Automated Machine Learning
REMOTE SENSING OF ENVIRONMENT(2021)
摘要
Root zone soil moisture (RZSM) estimation and monitoring based on high spatial resolution remote sensing information such as obtained with an Unmanned Aerial System (UAS) is of significant interest for field-scale precision irrigation management, particularly in water-limited regions of the world. To date, there is no accurate and widely accepted model that relies on UAS optical surface reflectance observations for RZSM estimation at high spatial resolution. This study is aimed at the development of a new approach for RZSM estimation based on the fusion of high spatial resolution optical reflectance UAS observations with physical and hydraulic soil information integrated into Automated Machine Learning (AutoML). The H2O AutoML platform includes a number of advanced machine learning algorithms that efficiently perform feature selection and automatically identify complex relationships between inputs and outputs. Twelve models combining UAS optical observations with various soil properties were developed in a hierarchical manner and fed into AutoML to estimate surface, near-surface, and root zone soil moisture. The addition of independently measured surface and near-surface soil moisture information to the hierarchical models to improve RZSM estimation was investigated. The accuracy of soil moisture estimates was evaluated based on a comparison with Time Domain Reflectometry (TDR) sensors that were deployed to monitor surface, near-surface and root zone soil moisture dynamics. The obtained results indicate that the consideration of physical and hydraulic soil properties together with UAS optical observations improves soil moisture estimation, especially for the root zone with a RMSE of about 0.04 cm3 cm-3. Accurate RZSM estimates were obtained when measured surface and near-surface soil moisture data was added to the hierarchical models, yielding RMSE values below 0.02 cm3 cm-3 and R and NSE values above 0.90. The generated high spatial resolution RZSM maps clearly capture the spatial variability of soil moisture at the field scale. The presented framework can aid farm scale precision irrigation management via improving the crop water use efficiency and reducing the risk of groundwater contamination.
更多查看译文
关键词
Soil moisture, Soil properties, Automated machine learning, UAS, Remote sensing
AI 理解论文
溯源树
样例
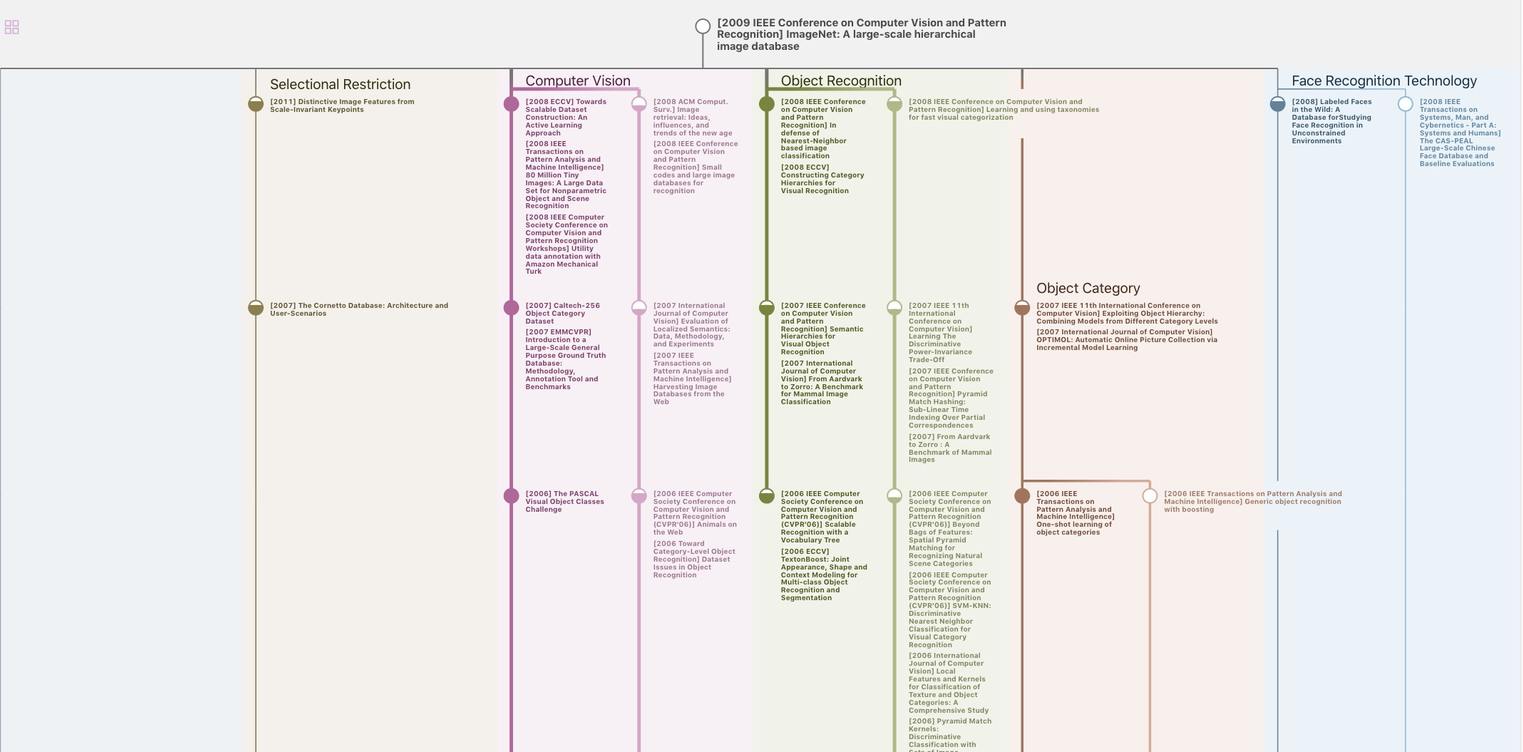
生成溯源树,研究论文发展脉络
Chat Paper
正在生成论文摘要