Revisiting Dropout: Escaping Pressure For Training Neural Networks With Multiple Costs
ELECTRONICS(2021)
摘要
A common approach to jointly learn multiple tasks with a shared structure is to optimize the model with a combined landscape of multiple sub-costs. However, gradients derived from each sub-cost often conflicts in cost plateaus, resulting in a subpar optimum. In this work, we shed light on such gradient conflict challenges and suggest a solution named Cost-Out, which randomly drops the sub-costs for each iteration. We provide the theoretical and empirical evidence of the existence of escaping pressure induced by the Cost-Out mechanism. While simple, the empirical results indicate that the proposed method can enhance the performance of multi-task learning problems, including two-digit image classification sampled from MNIST dataset and machine translation tasks for English from and to French, Spanish, and German WMT14 datasets.
更多查看译文
关键词
multitask learning, gradient conflict, Cost-Out, escaping pressure, dropout
AI 理解论文
溯源树
样例
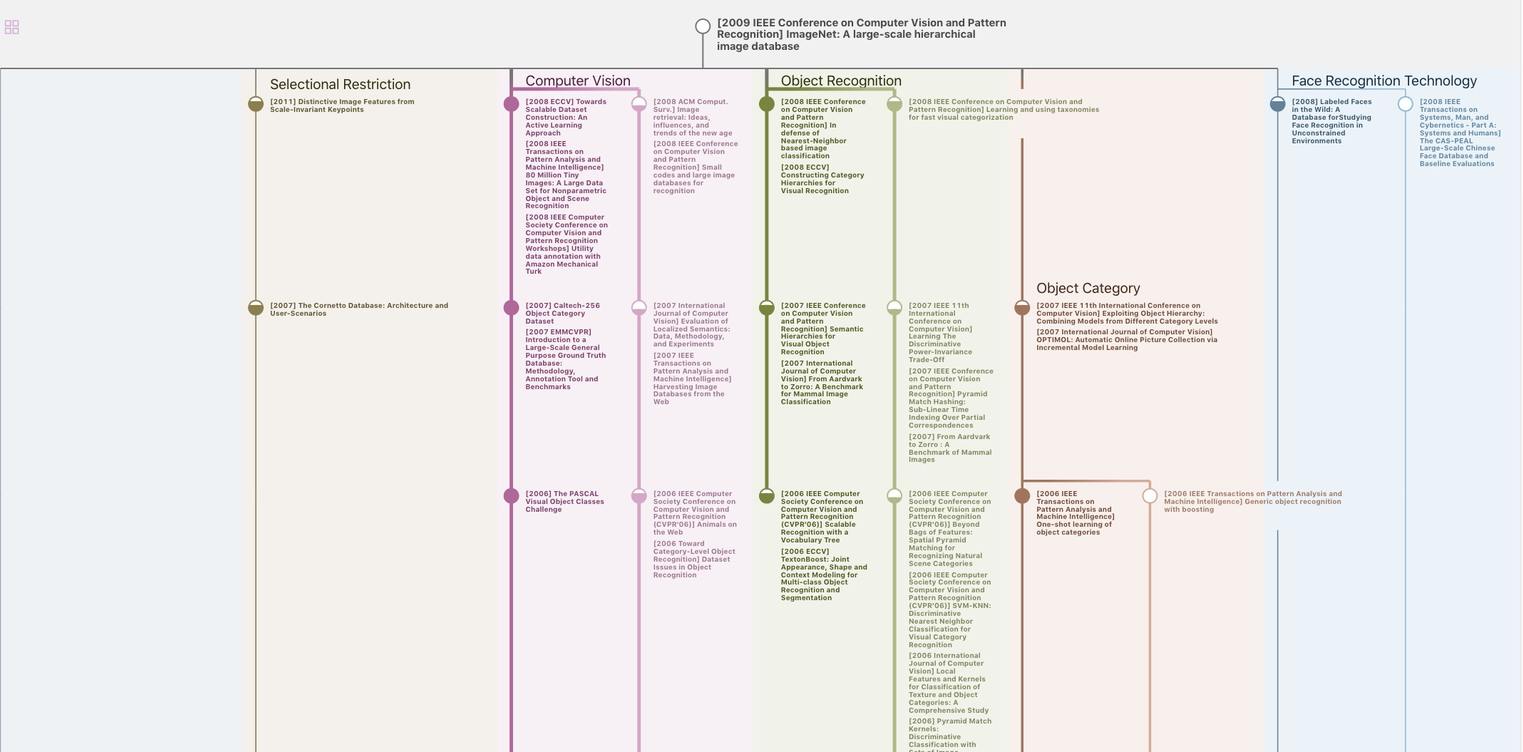
生成溯源树,研究论文发展脉络
Chat Paper
正在生成论文摘要