Multivariate Time Series Modelling For Urban Air Quality
URBAN CLIMATE(2021)
摘要
We introduce a spatio-temporal model to represent development of atmospheric pollution in an urban area. An important element of this is that recorded measurements are often incomplete which undermines time-series approaches. We identify the multiple imputation by chained equation (MICE) method as effective to complete data sequences synthetically. Following on from this, we develop a vector autoregressive moving average (VARMA) model for the spatio-temporal development of atmospheric pollution in urban areas. This model was fitted to hourly measurements of four pollutants (NO, NO2, NOx and PM10) for the whole of the calendar year 2017 at 30 stations across London, completed by MICE as required. We show by cross-validation that the VARMA model is more effective than other formulations, including the Kriging method of spatial interpolation, and seasonal ARMA models for individual stations with either daily or weekly trends. The resulting model can be used for prediction of air quality in different periods and as the basis for assessment of policy interventions such as increasing vehicle emission standards, and traffic management and control policies such as low and ultra-low emission zones.
更多查看译文
关键词
Air quality modelling, Multivariate time series analysis, Spatio-temporal analysis, Vector time-series imputation
AI 理解论文
溯源树
样例
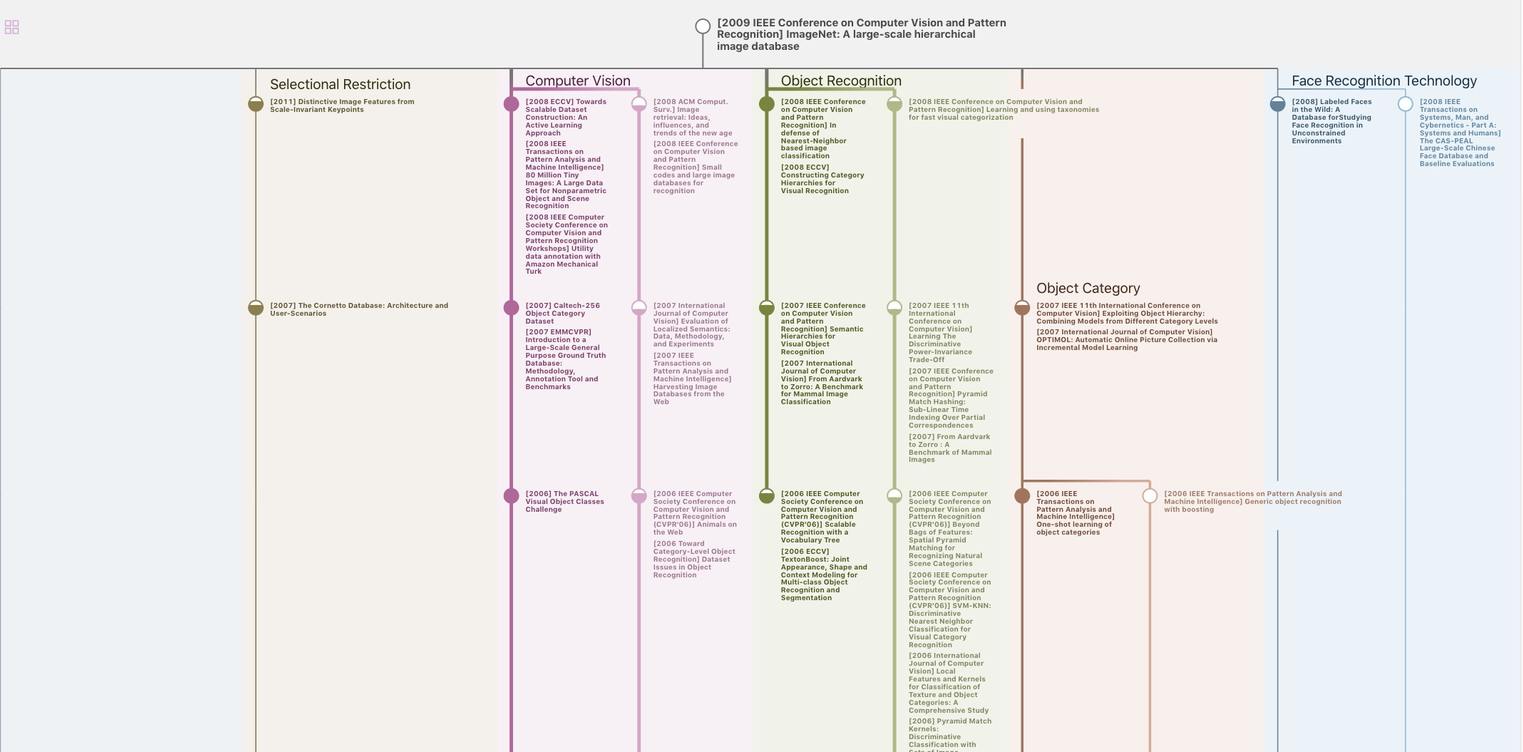
生成溯源树,研究论文发展脉络
Chat Paper
正在生成论文摘要