HOOPS: Human-in-the-Loop Graph Reasoning for Conversational Recommendation
Research and Development in Information Retrieval(2021)
摘要
ABSTRACTThere is increasing recognition of the need for human-centered AI that learns from human feedback. However, most current AI systems focus more on the model design, but less on human participation as part of the pipeline. In this work, we propose a Human-in-the-Loop (HitL) graph reasoning paradigm and develop a corresponding dataset named HOOPS for the task of KG-driven conversational recommendation. Specifically, we first construct a KG interpreting diverse user behaviors and identify pertinent attribute entities for each user--item pair. Then we simulate the conversational turns reflecting the human decision making process of choosing suitable items tracing the KG structures transparently. We also provide a benchmark method with reported performance on the dataset to ascertain the feasibility of HitL graph reasoning for recommendation using our developed dataset, and show that it provides novel opportunities for the research community.
更多查看译文
关键词
Human-in-the-Loop Learning, Graph Reasoning, Recommender Systems, Conversational Recommendation, Explainable Recommendation
AI 理解论文
溯源树
样例
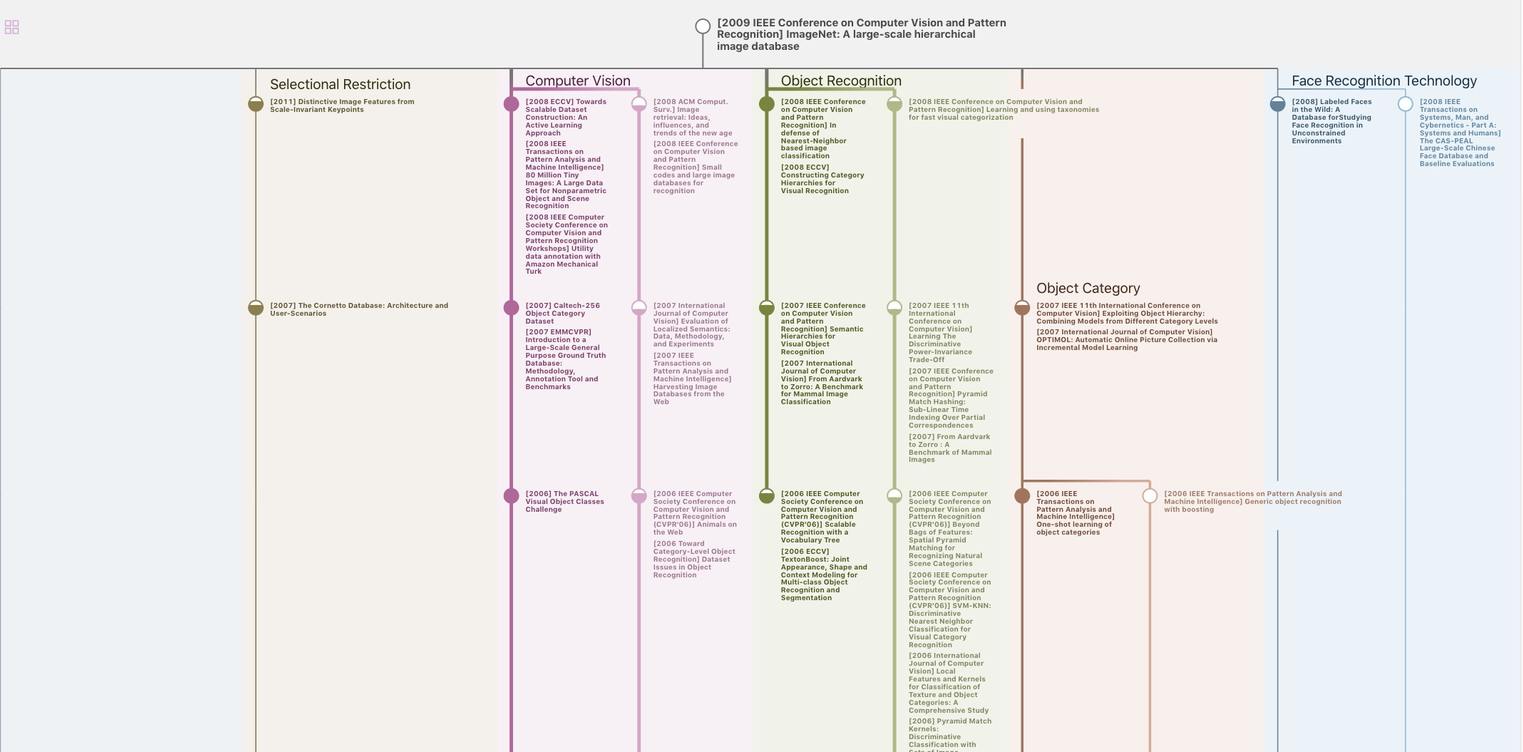
生成溯源树,研究论文发展脉络
Chat Paper
正在生成论文摘要