Revisiting the Tag Relevance Prediction Problem
Research and Development in Information Retrieval(2021)
摘要
ABSTRACTTraditionally, recommender systems provide a list of suggestions to a user based on past interactions with items of this user. These recommendations are usually based on user preferences for items and generated with a delay. Critiquing recommender systems allow users to provide immediate feedback to recommendations with tags and receive a new set of recommendations in response. However, these systems often require rich item descriptions that contain relevance scores indicating the strength, with which a tag applies to an item. For example, this relevance score could indicate how violent the movie "The Godfather" is on a scale from 0 to 1. Retrieving these data is a very demanding process, as it requires users to explicitly indicate the degree to which a tag applies to an item. This process can be improved with machine learning methods that predict tag relevance. In this paper, we explore the dataset from a different study, where the authors collected relevance scores on movie-tag pairs. In particular, we define the tag relevance prediction problem, explore the inconsistency of relevance scores provided by users as a challenge of this problem and present a method, which outperforms the state-of-the-art method for predicting tag relevance. We found a moderate inconsistency of user relevance scores. We also found that users tend to disagree more on subjective tags, such as "good acting", "bad plot" or "quotable" than on objective tags, such as "animation", "cars" or "wedding", but the disagreement of users regarding objective tags is also moderate.
更多查看译文
关键词
recommender systems, critiquing recommender systems, tag relevance prediction, tagging
AI 理解论文
溯源树
样例
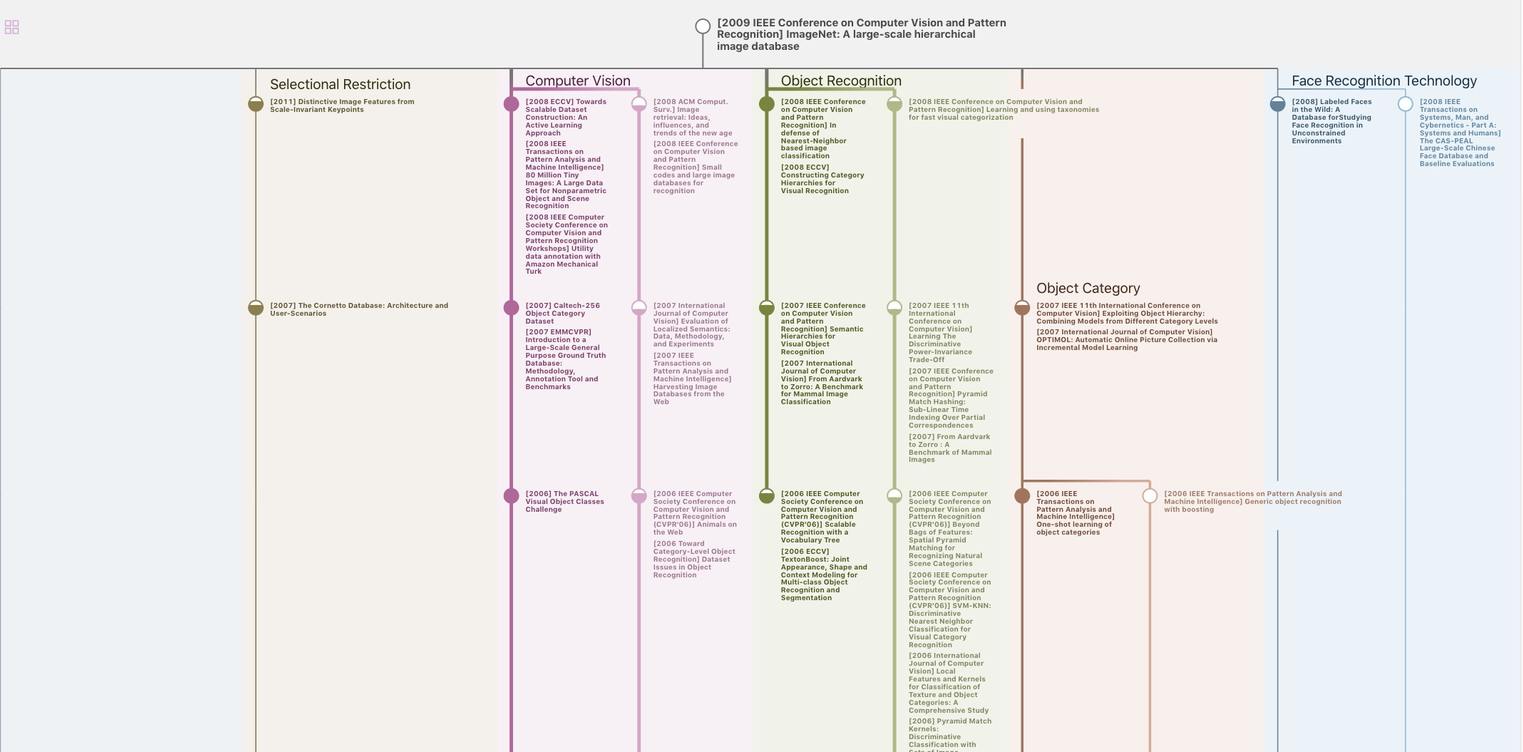
生成溯源树,研究论文发展脉络
Chat Paper
正在生成论文摘要