Non-Intrusive Load Monitoring Using Factorial Hidden Markov Model Based On Adaptive Density Peak Clustering
ENERGY AND BUILDINGS(2021)
摘要
With the goal of achieving carbon neutrality, the technology of Non-Intrusive Load Monitoring (NILM) has gained widespread attention as an efficient energy-saving way. Hidden Markov Model (HMM) based methods are popular in the field of NILM, yet the traditional HMM-based methods need prior knowledge of the appliance's working states. In this paper, we propose an Adaptive Density Peak Clustering (ADPC) algorithm that could automatically determine the working states of appliance based on its power consumption. Then we combine the ADPC and Factorial Hidden Markov Model (FHMM) to create an Adaptive Density Peak Clustering-Factorial Hidden Markov Model (ADPC-FHMM), which reduces the dependence of prior information and is more applicable in real world scenarios. Case studies are conducted on two publicly available datasets, and the results show that the proposed model outperforms its counterparts on the metrics of Accuracy, F-measure and MAE.(c) 2021 Elsevier B.V. All rights reserved.
更多查看译文
关键词
Carbon neutrality, NILM, HMM, ADPC-FHMM, Load disaggregation
AI 理解论文
溯源树
样例
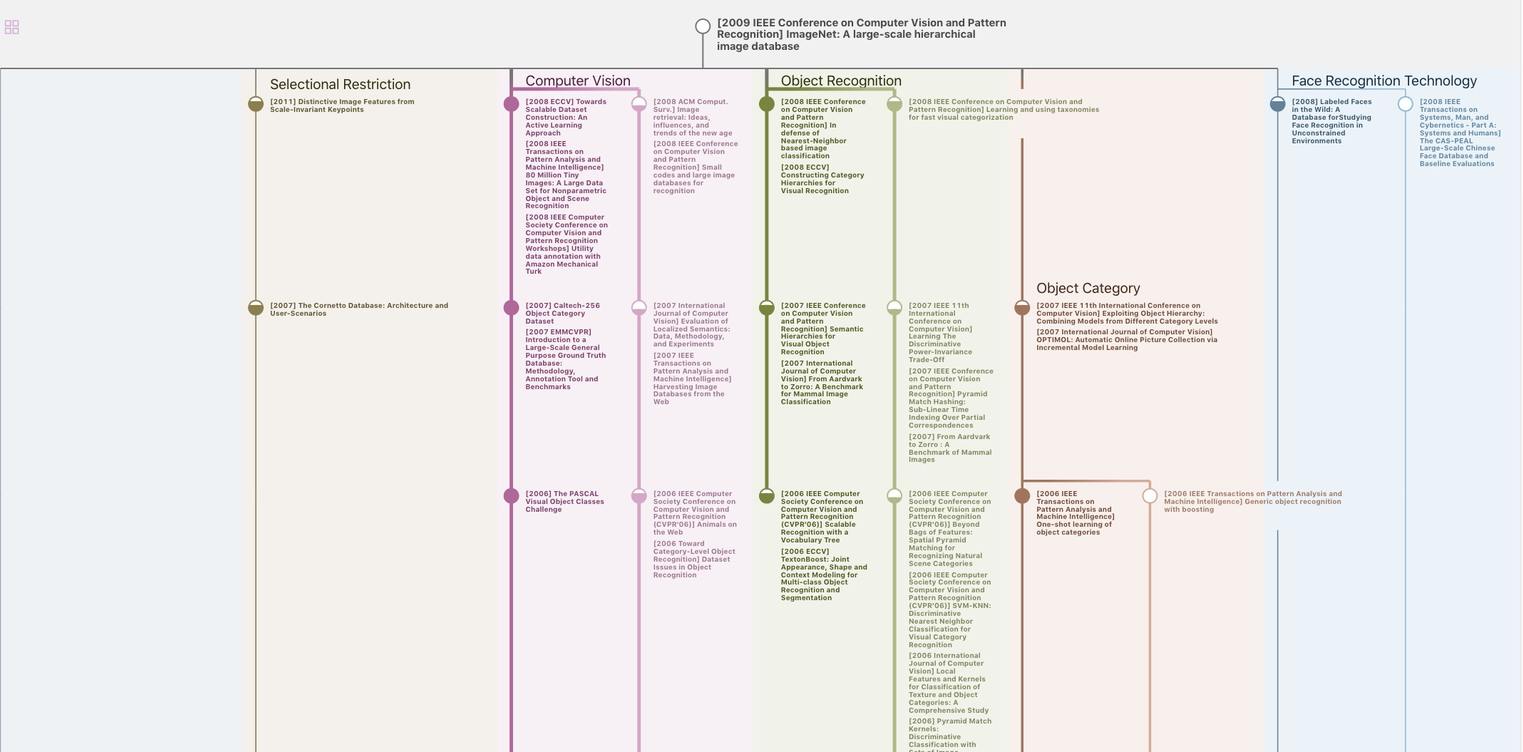
生成溯源树,研究论文发展脉络
Chat Paper
正在生成论文摘要