A Game-Theoretic Approach For Robust Federated Learning
INTERNATIONAL JOURNAL OF ENGINEERING(2021)
摘要
Federated learning enables aggregating models trained over a large number of clients by sending the models to a central server, while data privacy is preserved since only the models are sent. Federated learning techniques are considerably vulnerable to poisoning attacks. In this paper, we explore the threat of poisoning attacks and introduce a game-based robust federated averaging algorithm to detect and discard bad updates provided by the clients. We model the aggregating process with a mixed-strategy game that is played between the server and each client. The valid actions of the clients are to send good or bad updates while the server can accept or ignore these updates as its valid actions. By employing the Nash Equilibrium property, the server determines the probability of providing good updates by each client. The experimental results show that our proposed game-based aggregation algorithm is significantly more robust to faulty and noisy clients in comparison with the most recently presented methods. According to these results, our algorithm converges after a maximum of 30 iterations and can detect 100% of the bad clients for all the investigated scenarios. In addition, the accuracy of the proposed algorithm is at least 15.8% and 2.3% better than the state of the art for flipping and noisy scenarios, respectively.
更多查看译文
关键词
Federated Learning, Game Theory, Byzantine Model, Adaptive Averaging
AI 理解论文
溯源树
样例
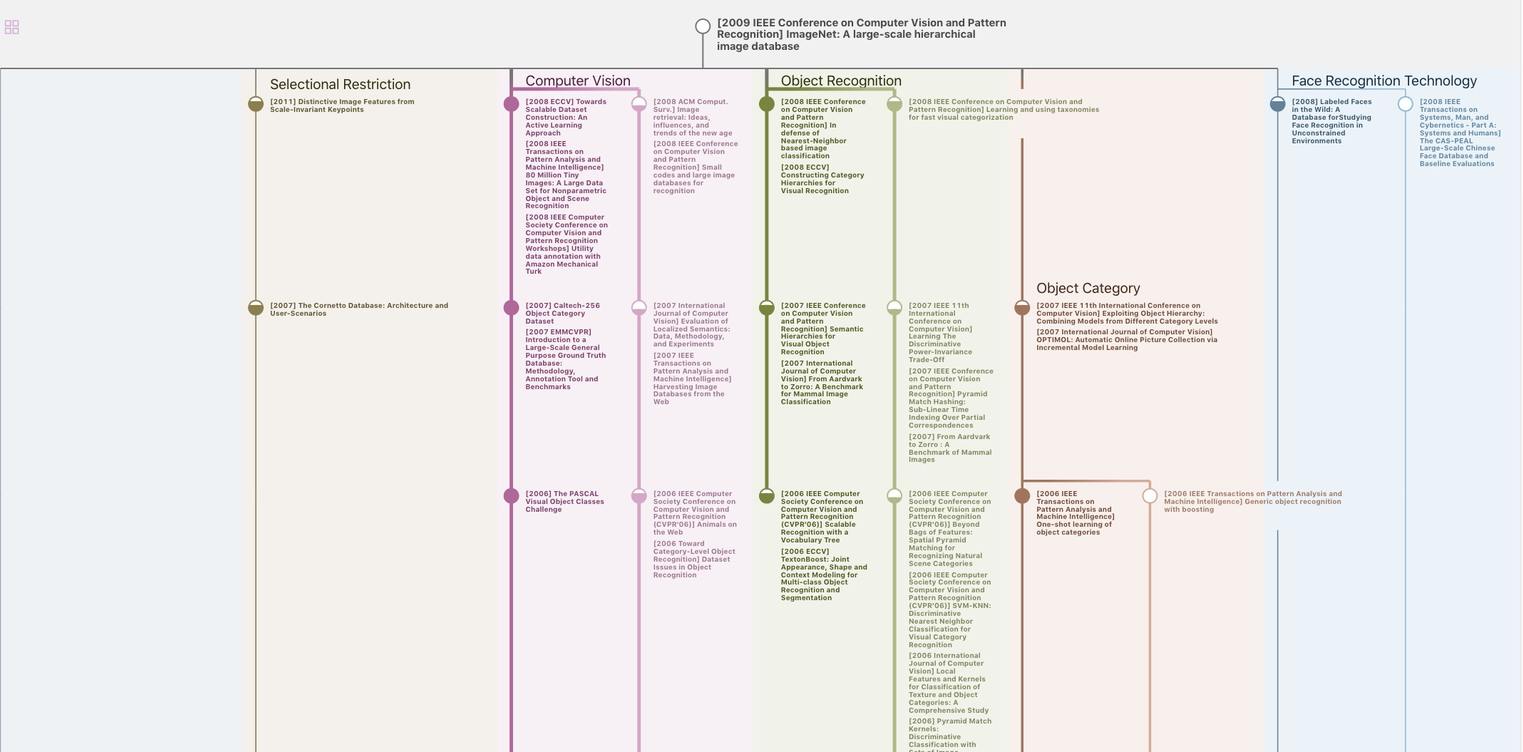
生成溯源树,研究论文发展脉络
Chat Paper
正在生成论文摘要