DeepLoRa: Learning Accurate Path Loss Model for Long Distance Links in LPWAN
IEEE CONFERENCE ON COMPUTER COMMUNICATIONS (IEEE INFOCOM 2021)(2021)
摘要
LoRa (Long Range) is an emerging wireless technology that enables long-distance communication and keeps low power consumption. Therefore, LoRa plays a more and more important role in Low-Power Wide-Area Networks (LPWANs), which easily extend many large-scale Internet of Things (IoT) applications in diverse scenarios (e.g., industry, agriculture, city). In lots of environments where various types of land-covers usually exist, it is challenging to precisely predict a LoRa link's path loss. As a result, how to deploy LoRa gateways to ensure reliable coverage and develop precise fingerprint-based localization becomes a difficult issue in practice. In this paper, we propose DeepLoRa, a deep learning-based approach to accurately estimate the path loss of long-distance links in complex environments. Specifically, DeepLoRa relies on remote sensing to automatically recognize land-cover types along a LoRa link. Then, DeepLoRa utilizes Bi-LSTM (Bidirectional Long Short Term Memory) to develop a land-cover aware path loss model. We implement DeepLoRa and use the data gathered from a real LoRaWAN deployment on campus to evaluate its performance extensively in terms of estimation accuracy and model transferability. The results show that DeepLoRa reduces the estimation error to less than 4 dB, which is 2 x smaller than state-of-the-art models.
更多查看译文
关键词
Wireless networks, Low power wide area networks, Attenuation measurement, Propagation losses, Recurrent neural networks
AI 理解论文
溯源树
样例
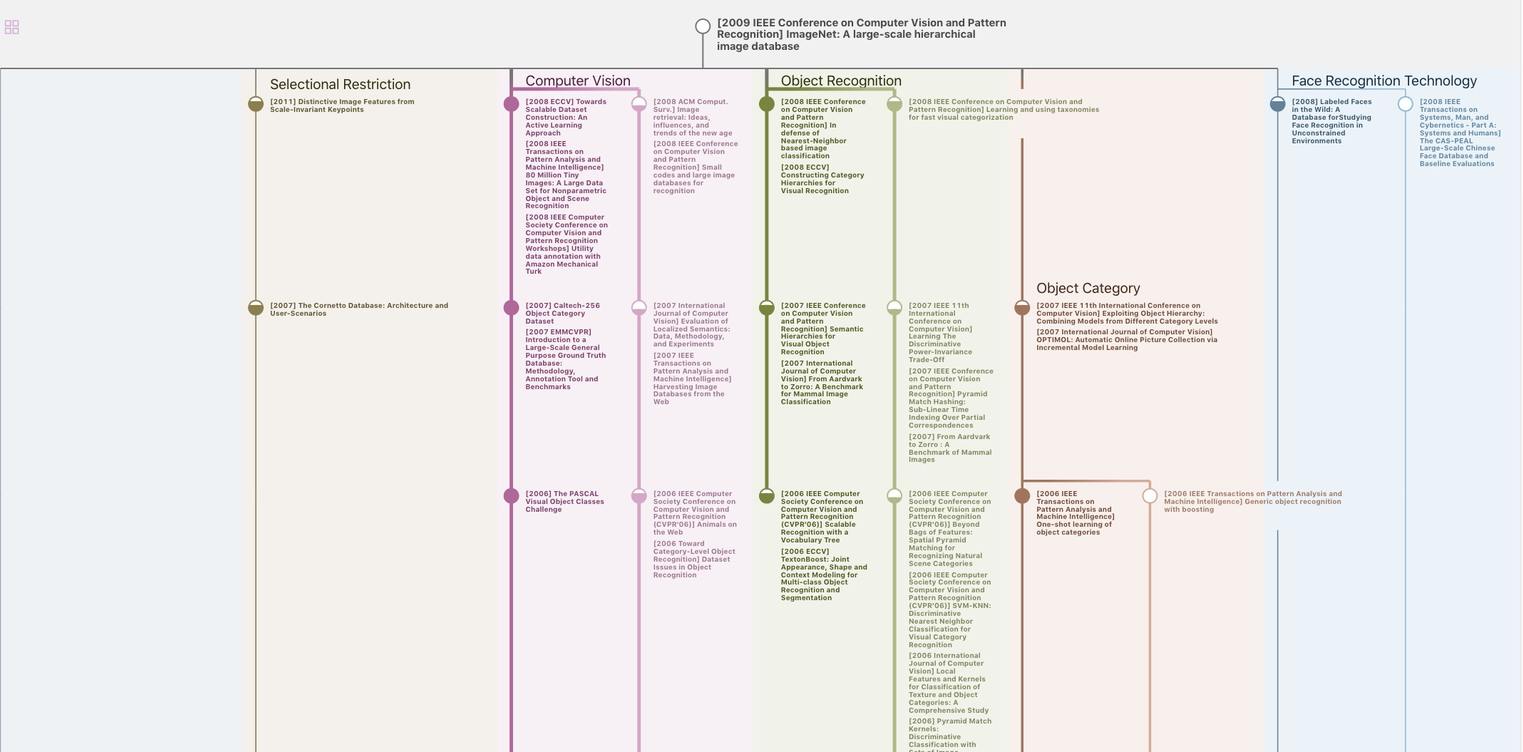
生成溯源树,研究论文发展脉络
Chat Paper
正在生成论文摘要