Historical Land Cover Classification From Corona Imagery Using Convolutional Neural Networks And Geometric Moments
INTERNATIONAL JOURNAL OF REMOTE SENSING(2021)
摘要
Historical CORONA imagery, a very high-resolution panchromatic imagery captured during 1960-72, has a lot of unexplored potentials to represent the land cover of the pre-Landsat era. This pre-Landsat land cover can be a valuable input to many long-term environmental modelling problems. While automated binary change detection and multi-class classification by manual photo-interpretation have been the two most popular applications of CORONA imagery, automated multi-class classification of CORONA imagery has not been explored. In this research, we propose two supervised classification methods capable of extracting spatial features for the classification of CORONA imagery. The first method utilizes two-dimensional convolutional neural networks (2D-CNN) for classification, whereas the second method involves stacking the CORONA imagery with its texture features obtained through geometric moments (GM) and performing pixel-wise classification using the random forest (RF) classifier and is termed as GM-RF. The proposed methods are tested on two study sites - the critical zone observatory (CZO) managed by the Indian Institute of Technology Kanpur in Uttar Pradesh (India) and a site near Chhapra in Bihar (India). For estimating the capabilities of the proposed methods and to compare the proposed methods with other methods, overall accuracy and Cohen's kappa coefficient (k) along with z-scores statistics are used. The effects of various hyperparameters (e.g. percentage of training data, patch size, order of geometric moments) on accuracy are also evaluated. The classification accuracies achieved through the 2D-CNN and GM-RF method in terms of are higher than 90% for both study sites indicating that the proposed methods can classify CORONA imagery with little domain knowledge about the study areas, and hence can be used in a variety of applications.
更多查看译文
AI 理解论文
溯源树
样例
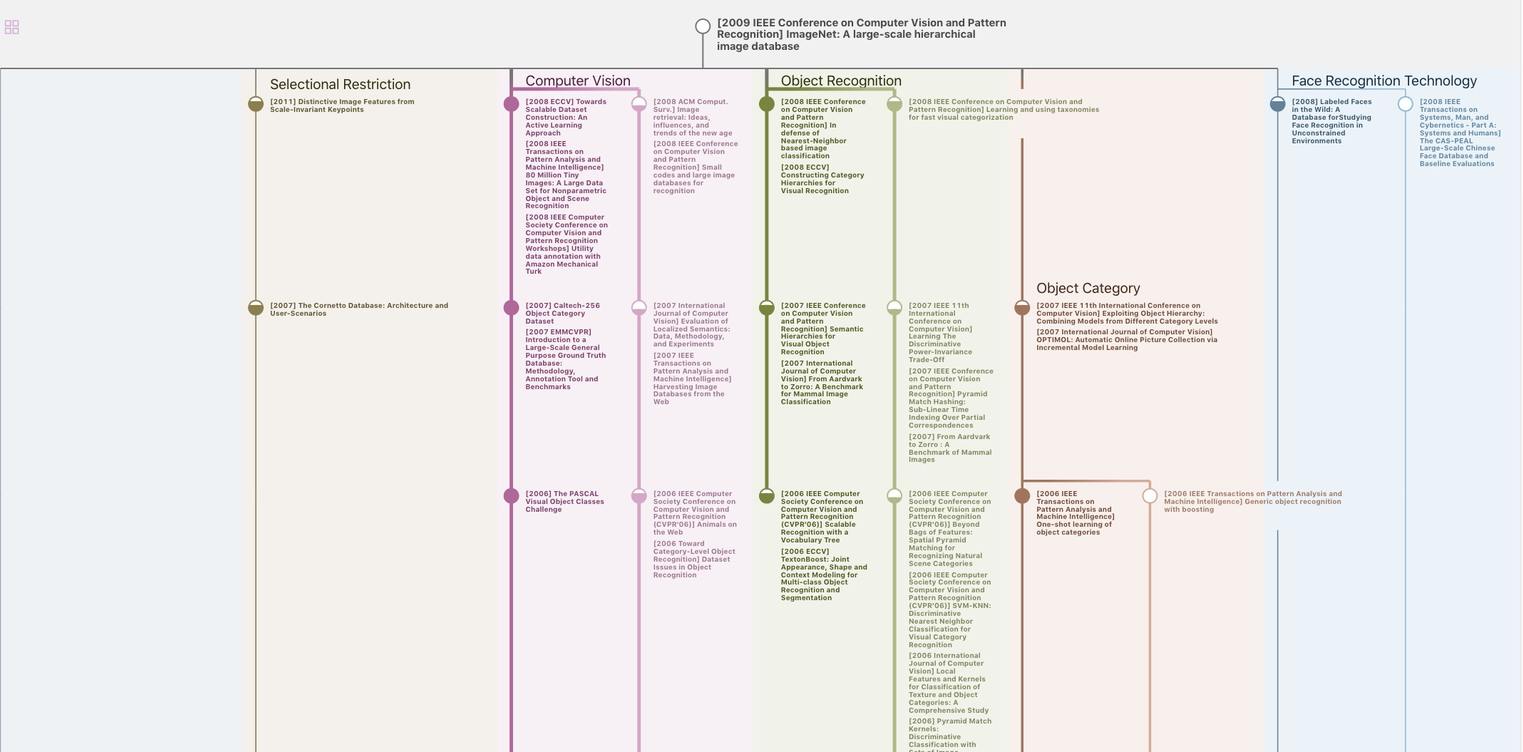
生成溯源树,研究论文发展脉络
Chat Paper
正在生成论文摘要