variational combinatorial sequential monte carlo methods for bayesian phylogenetic inference.
UAI(2021)
摘要
Bayesian phylogenetic inference is often conducted via local or sequential search over topologies and branch lengths using algorithms such as random-walk Markov chain Monte Carlo (MCMC) or Combinatorial Sequential Monte Carlo (CSMC). However, when MCMC is used for evolutionary parameter learning, convergence requires long runs with inefficient exploration of the state space. We introduce Variational Combinatorial Sequential Monte Carlo (VCSMC), a powerful framework that establishes variational sequential search to learn distributions over intricate combinatorial structures. We then develop nested CSMC, an efficient proposal distribution for CSMC and prove that nested CSMC is an exact approximation to the (intractable) locally optimal proposal. We use nested CSMC to define a second objective, VNCSMC which yields tighter lower bounds than VCSMC. We show that VCSMC and VNCSMC are computationally efficient and explore higher probability spaces than existing methods on a range of tasks.
更多查看译文
关键词
bayesian,variational
AI 理解论文
溯源树
样例
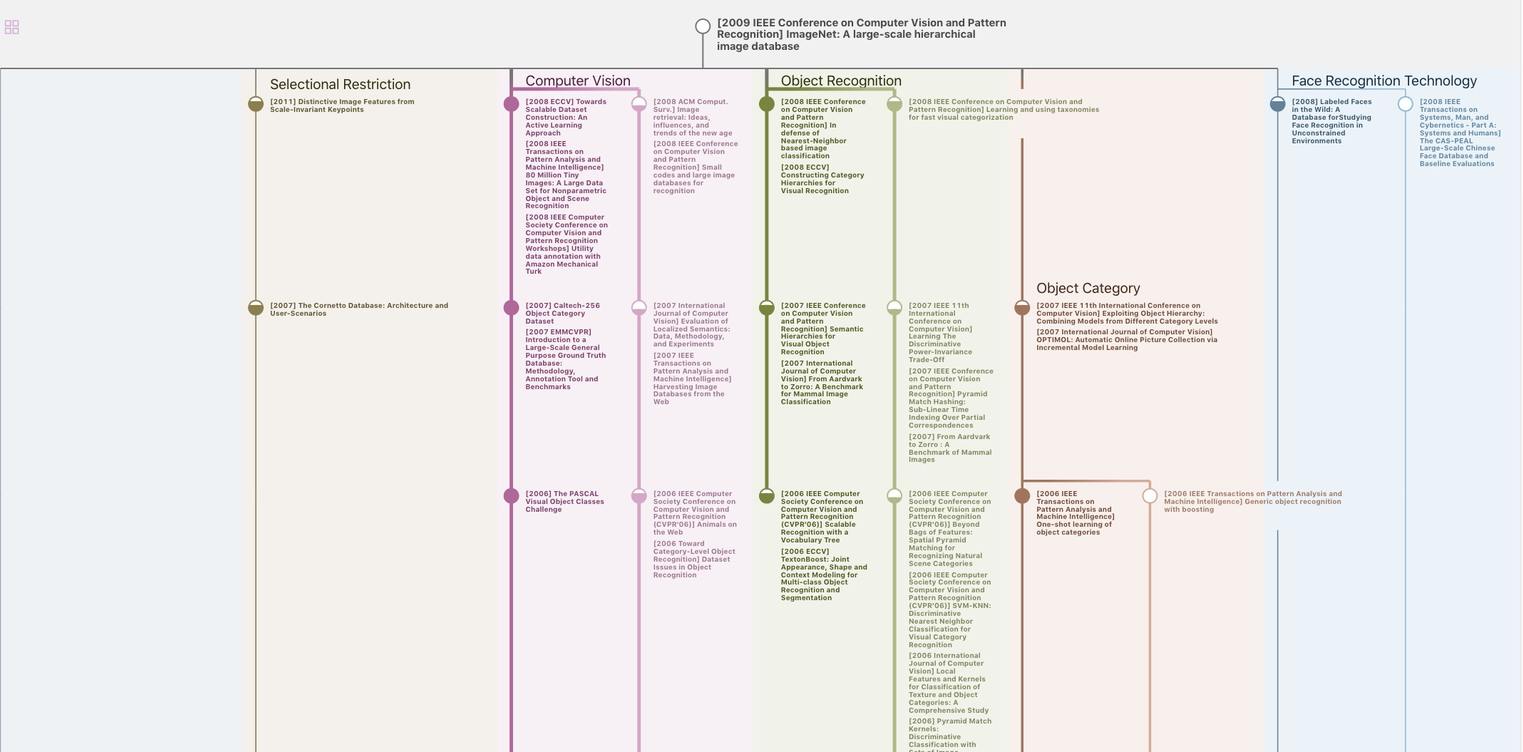
生成溯源树,研究论文发展脉络
Chat Paper
正在生成论文摘要