ACCURATE 3D KIDNEY SEGMENTATION USING UNSUPERVISED DOMAIN TRANSLATION AND ADVERSARIAL NETWORKS
2021 IEEE 18TH INTERNATIONAL SYMPOSIUM ON BIOMEDICAL IMAGING (ISBI)(2021)
摘要
Computed tomography urography imaging is routinely performed to evaluate the kidneys. Kidney 3D segmentation and reconstruction from urographic images provides physicians with an intuitive visualization way to accurately diagnose and treat kidney diseases, particularly used in surgical planning and outcome analysis before and after kidney surgery. While 3D fully convolution networks have achieved a big success in medical image segmentation, they get trapped in clinical unseen data and cannot be adapted in deferent modalities with one training procedure. This study proposes an unsupervised domain adaptation or translation method with 2D networks to deeply learn urographic images for accurate kidney segmentation. We tested our proposed method on clinical urography data. The experimental results demonstrate our proposed method can resolve the domain shift problem of kidney segmentation and achieve the comparable or better results than supervised learning based segmentation methods.
更多查看译文
关键词
Kidney segmentation, CT urography, unsupervised domain adaptation, deep learning
AI 理解论文
溯源树
样例
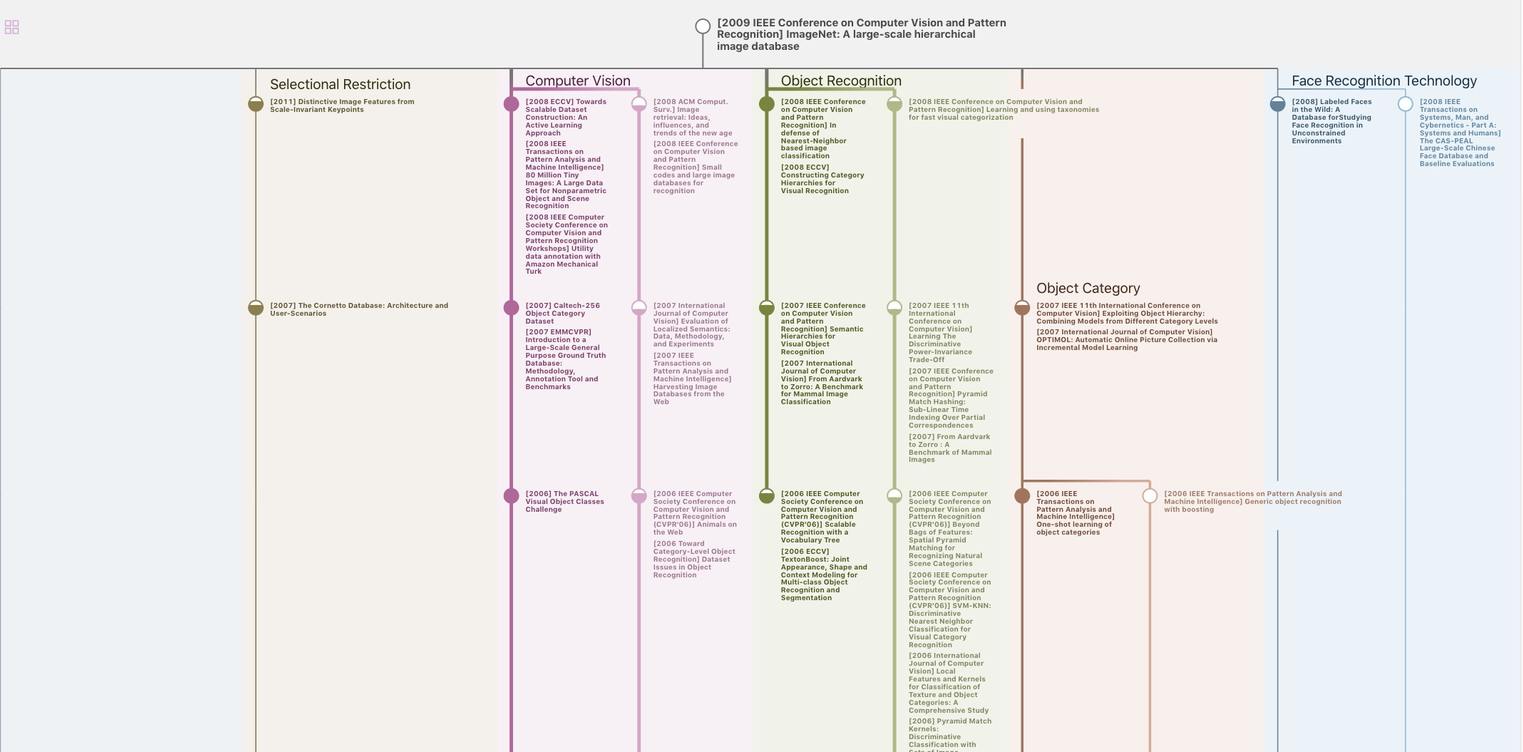
生成溯源树,研究论文发展脉络
Chat Paper
正在生成论文摘要