FAST WHOLE SLIDE IMAGE ANALYSIS OF CERVICAL CANCER USING WEAK ANNOTATION
2021 IEEE 18TH INTERNATIONAL SYMPOSIUM ON BIOMEDICAL IMAGING (ISBI)(2021)
摘要
Cervical cancer is the most common gynecological disease, which seriously endangers women's health. Histopathological diagnosis is the most reliable determinant for cervical cancer prognosis and treatment. However, it is a time-consuming and error-prone task for pathologists to manually analyze the whole slide image(WSI). Automated detection of cancer tissue and histopathological diagnosis are of great signification to reduce the workload for pathologists. So we proposed a method combining deep learning(DL) and traditional machine learning(ML) to realize the rapid analysis of the cervical cancer WSI. Our method first applied a patch-level network to detect the pathological patches and generate a heatmap that can highlight the tumor area. Considering the difficulty of obtaining pixel-level annotations, we designed a weak annotation strategy for training the network and verified the feasibility of this strategy on a public dataset. Besides, we used overlapping heatmap generation strategy(Overlapping-HMGS) and feature sharing strategy of fully convolutional neural networks(CNN) to balance the accuracy and speed of tumor region detection. Then features extracted from the heatmaps are fed into a traditional machine learning model for the WSI-level prediction. Our approach not only achieved excellent performance with 97% accuracy on our cervical cancer WSI dataset, but it is also efficient and interpretable.
更多查看译文
关键词
Cervical Cancer, Deep Learning, Weak Annotation, Whole Slide Image
AI 理解论文
溯源树
样例
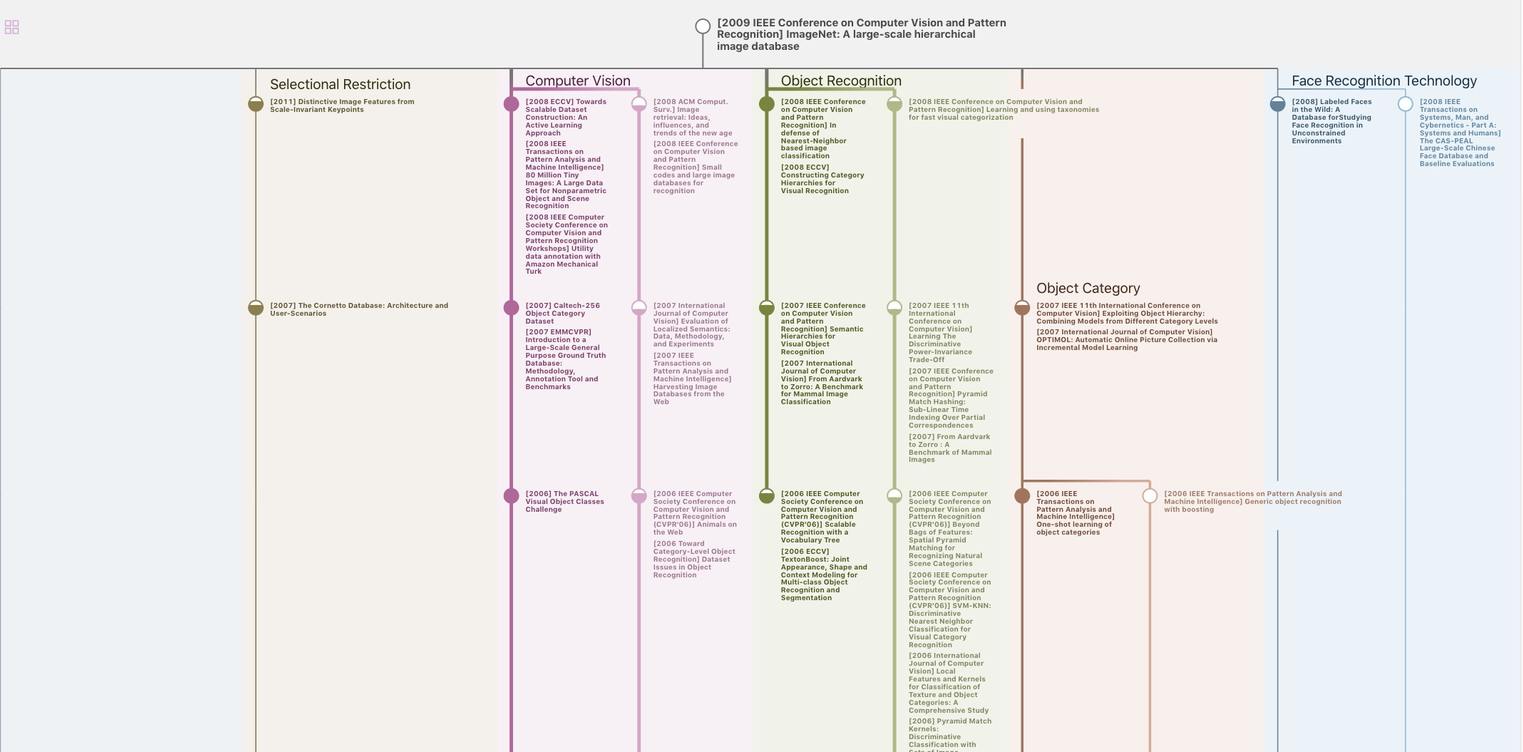
生成溯源树,研究论文发展脉络
Chat Paper
正在生成论文摘要