Annotation-Efficient 3d U-Nets For Brain Plasticity Network Mapping
2021 IEEE 18TH INTERNATIONAL SYMPOSIUM ON BIOMEDICAL IMAGING (ISBI)(2021)
摘要
A fundamental challenge in machine learning-based segmentation of large-scale brain microscopy images is the time and domain expertise required by humans to generate ground truth for model training. Weakly supervised and semi-supervised approaches can greatly reduce the burden of human annotation. Here we present a study of three-dimensional U-Nets with varying levels of supervision to perform neuronal nuclei segmentation in light-sheet microscopy volumes. We leverage automated blob detection with classical algorithms to generate noisy labels on a large volume, and our experiments show that weak supervision, with or without additional fine-tuning, can outperform resource-limited fully supervised learning. These methods are extended to analyze coincidence between multiple fluorescent stains in cleared brain tissue. This is an initial step towards automated whole-brain analysis of plasticity-related gene expression.
更多查看译文
关键词
Brain plasticity, deep learning, light sheet microscopy, neuron segmentation, weak supervision
AI 理解论文
溯源树
样例
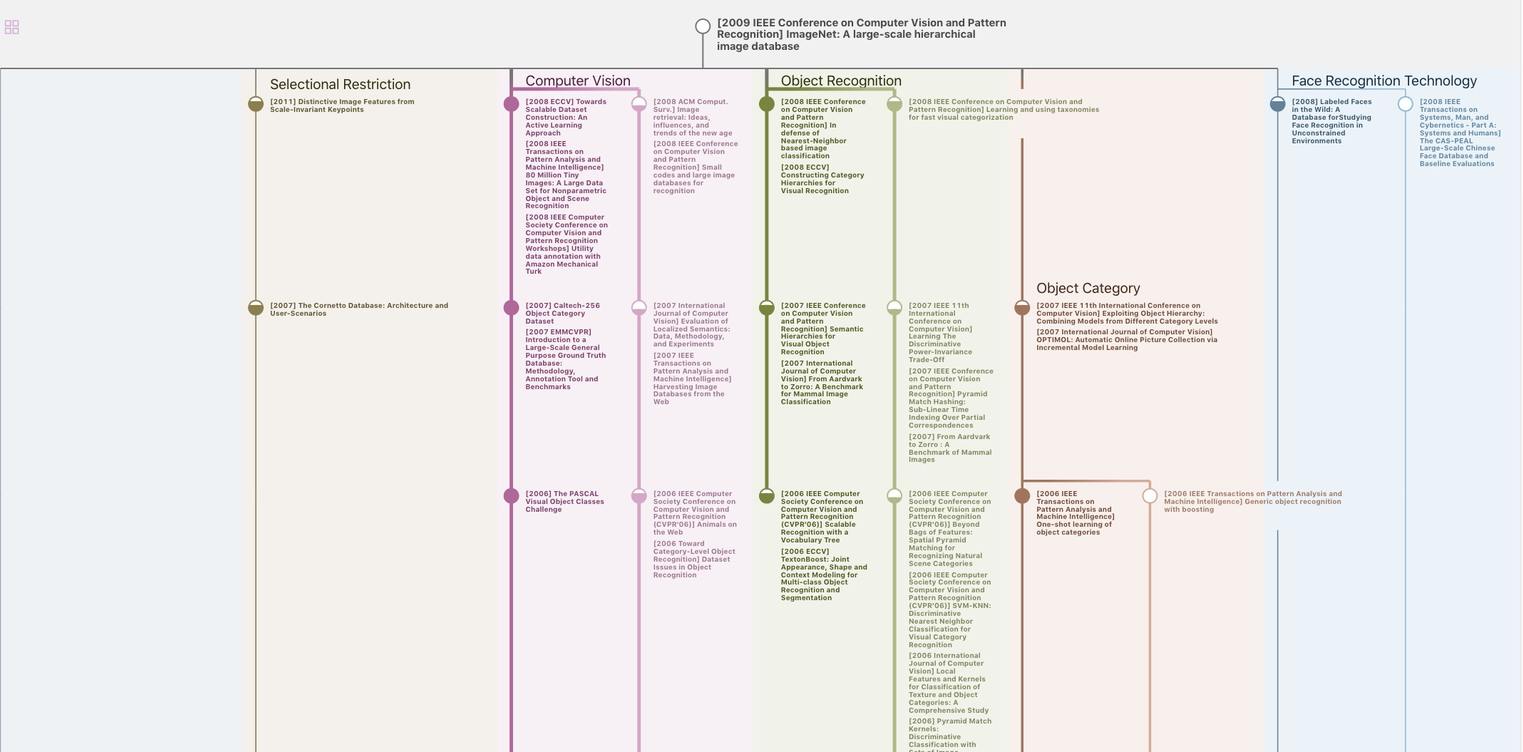
生成溯源树,研究论文发展脉络
Chat Paper
正在生成论文摘要