COMPARISON OF CLASSICAL MACHINE LEARNING & DEEP LEARNING TO CHARACTERISE FIBROSIS & INFLAMMATION USING QUANTITATIVE MRI
2021 IEEE 18TH INTERNATIONAL SYMPOSIUM ON BIOMEDICAL IMAGING (ISBI)(2021)
摘要
The quantitative MRI metric, T1, has been used to characterise fibroinflammation in the liver; however, the T1 value alone is unable to differentiate between fibrosis and inflammation. We evaluate the potential utility of classical machine learning techniques (K-Nearest Neighbours, Support Vector Machine and Random Forest) to address this problem using information in the T1 map. We also compare to transfer learning, utilising multiple methods to alleviate the effects of class imbalance. Random Forest with Adaptive Synthetic Sampling was superior to mean T1 in categorising fibroinflammation. Despite the relatively small number of samples (n=289) and large class imbalance, our results demonstrate potential in using the whole T1 map with machine learning for this task.
更多查看译文
关键词
T1 mapping, Liver, Random Forests, KNN, SVM, Transfer Learning, Wideresnet
AI 理解论文
溯源树
样例
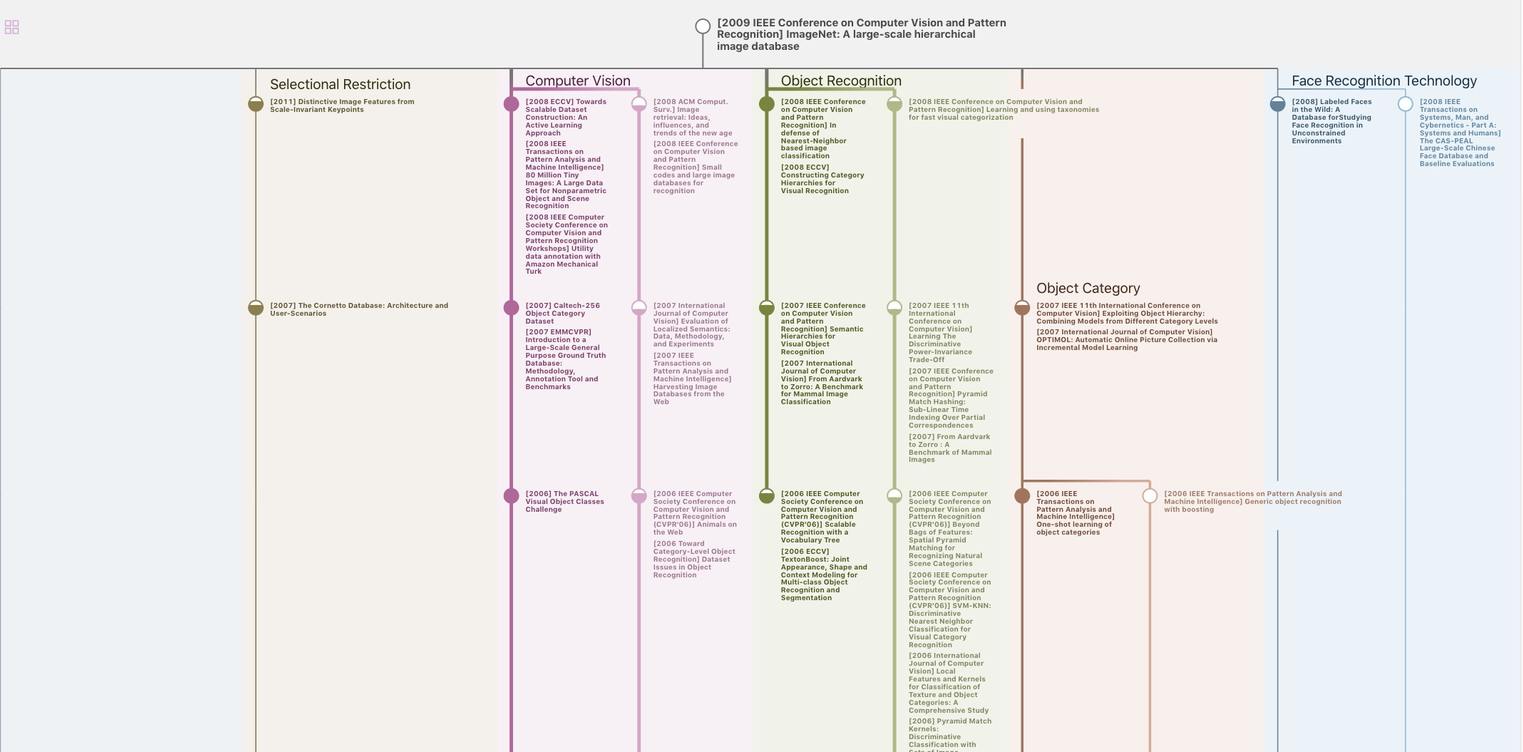
生成溯源树,研究论文发展脉络
Chat Paper
正在生成论文摘要