Federated Learning for Site Aware Chest Radiograph Screening
2021 IEEE 18TH INTERNATIONAL SYMPOSIUM ON BIOMEDICAL IMAGING (ISBI)(2021)
摘要
The shortage of Radiologists is inspiring the development of Deep Learning (DL) based solutions for detecting cardio, thoracic and pulmonary pathologies in Chest radiographs through multi-institutional collaborations. However, sharing the training data across multiple sites is often impossible due to privacy, ownership and technical challenges. Although Federated Learning (FL) has emerged as a solution to this, the large variations in disease prevalence and co-morbidity distributions across the sites may hinder proper training. We propose a DL architecture with a Convolutional Neural Network (CNN) followed by a Graph Neural Network (GNN) to address this issue. The CNN-GNN model is trained by modifying the Federated Averaging algorithm. The CNN weights are shared across all sites to extract robust features while separate GNN models are trained at each site to leverage the local co-morbidity dependencies for multi-label disease classification. The CheXpert dataset is partitioned across five sites to simulate the FL set up. Federated training did not show any significant drop in performance over centralized training. The site-specific GNN models also demonstrated their efficacy in modelling local disease co-occurrence statistics leading to an average area under the ROC curve of 0.79 with a 1.74% improvement.
更多查看译文
关键词
Chest X-ray, Federated learning, Graph Neural Networks, Convolutional Neural Networks
AI 理解论文
溯源树
样例
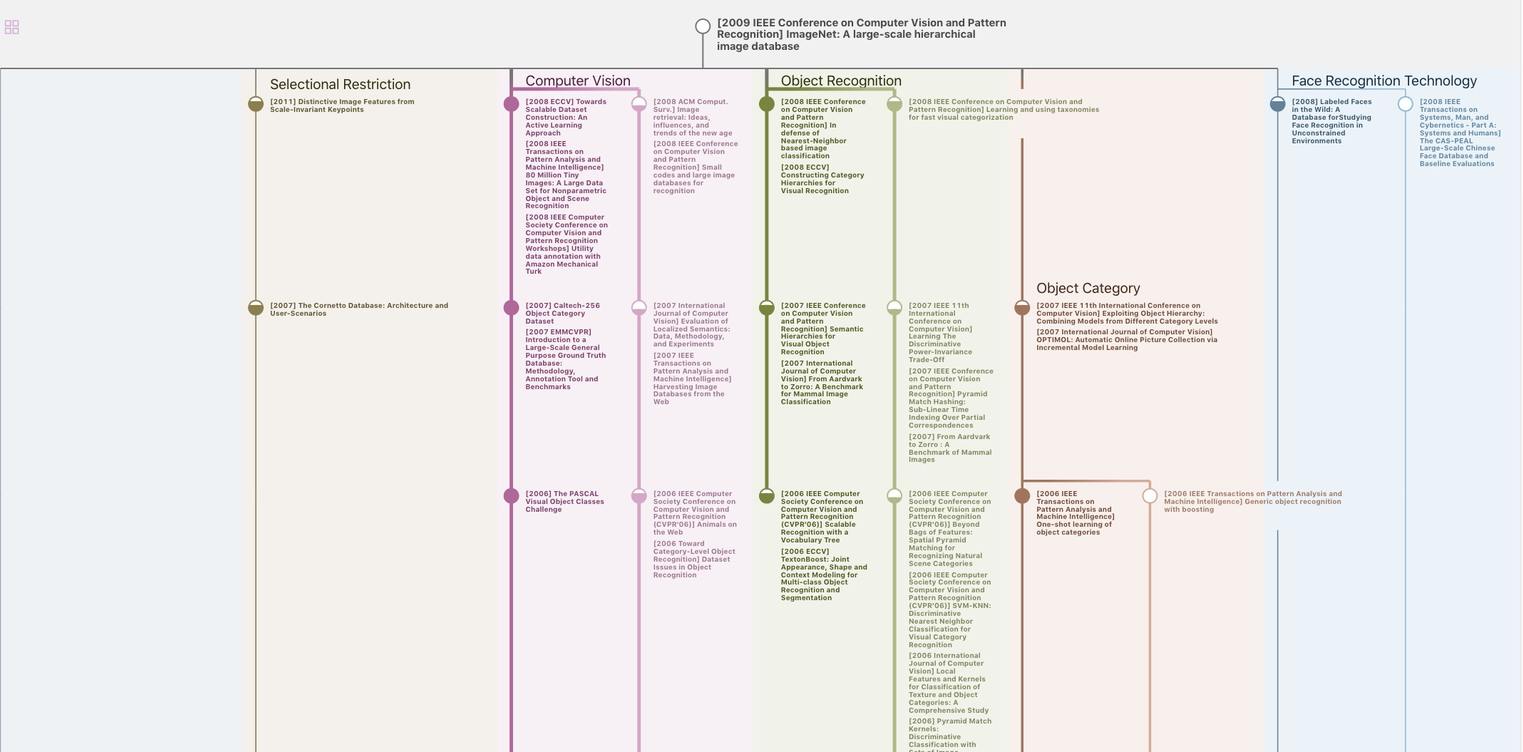
生成溯源树,研究论文发展脉络
Chat Paper
正在生成论文摘要