Fitting Structural Equation Models via Variational Approximations
arxiv(2021)
摘要
Structural equation models are commonly used to capture the relationship between sets of observed and unobservable variables. Traditionally these models are fitted using frequentist approaches but recently researchers and practitioners have developed increasing interest in Bayesian inference. In Bayesian settings, inference for these models is typically performed via Markov chain Monte Carlo methods, which may be computationally intensive for models with a large number of manifest variables or complex structures. Variational approximations can be a fast alternative; however, they have not been adequately explored for this class of models. We develop a mean field variational Bayes approach for fitting elemental structural equation models and demonstrate how bootstrap can considerably improve the variational approximation quality. We show that this variational approximation method can provide reliable inference while being significantly faster than Markov chain Monte Carlo.
更多查看译文
关键词
Approximate inference,confirmatory factor analysis,latent variables,mean field variational Bayes,nonparametric bootstrap
AI 理解论文
溯源树
样例
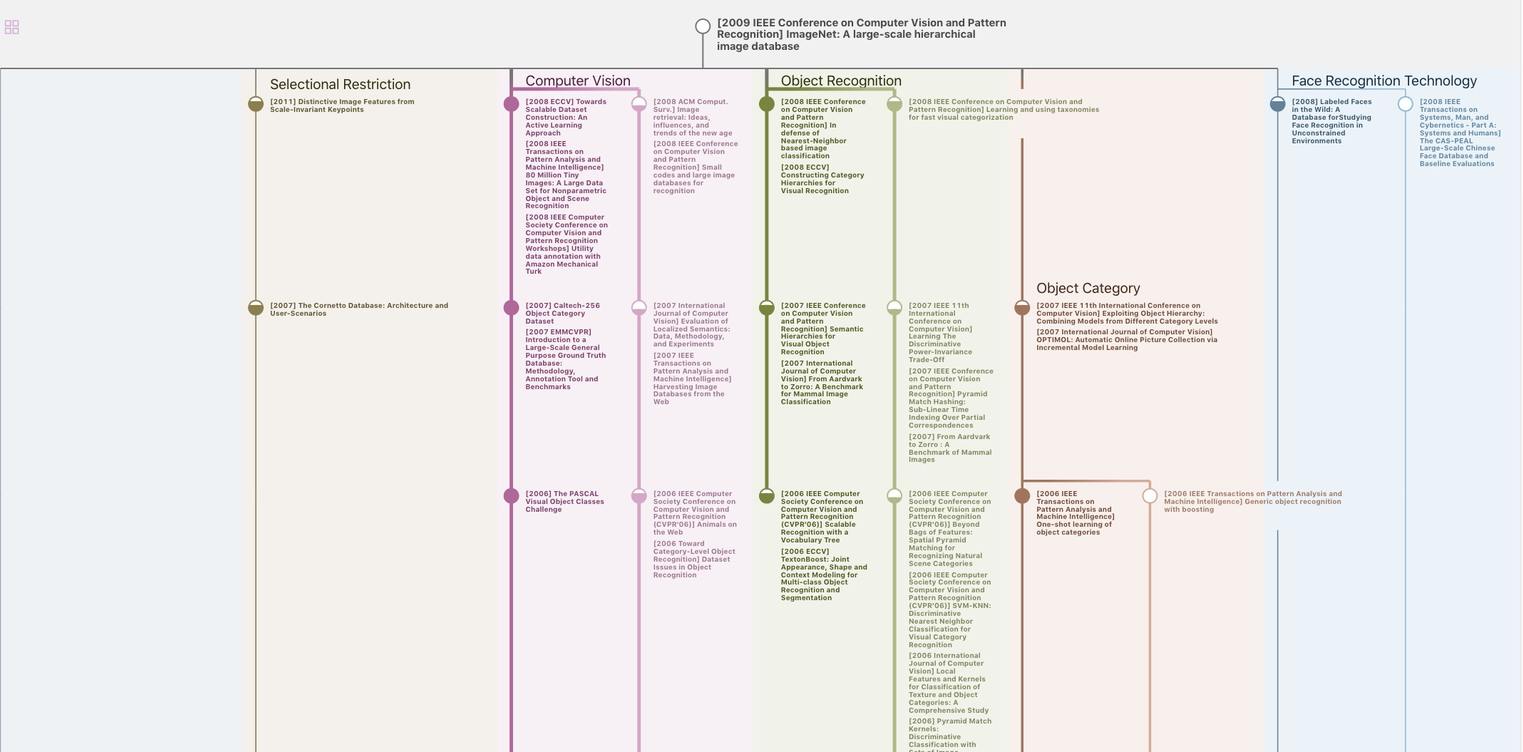
生成溯源树,研究论文发展脉络
Chat Paper
正在生成论文摘要