Transfer Learning for Sequence Generation: from Single-source to Multi-source.
Annual Meeting of the Association for Computational Linguistics(2021)
摘要
Multi-source sequence generation (MSG) is an important kind of sequence generation tasks that takes multiple sources, including automatic post-editing, multi-source translation, multi-document summarization, etc. As MSG tasks suffer from the data scarcity problem and recent pretrained models have been proven to be effective for low-resource downstream tasks, transferring pretrained sequence-to-sequence models to MSG tasks is essential. Although directly finetuning pretrained models on MSG tasks and concatenating multiple sources into a single long sequence is regarded as a simple method to transfer pretrained models to MSG tasks, we conjecture that the direct finetuning method leads to catastrophic forgetting and solely relying on pretrained self-attention layers to capture cross-source information is not sufficient. Therefore, we propose a two-stage finetuning method to alleviate the pretrain-finetune discrepancy and introduce a novel MSG model with a fine encoder to learn better representations in MSG tasks. Experiments show that our approach achieves new state-of-the-art results on the WMT17 APE task and multi-source translation task using the WMT14 test set. When adapted to document-level translation, our framework outperforms strong baselines significantly.
更多查看译文
关键词
sequence generation,learning,single-source,multi-source
AI 理解论文
溯源树
样例
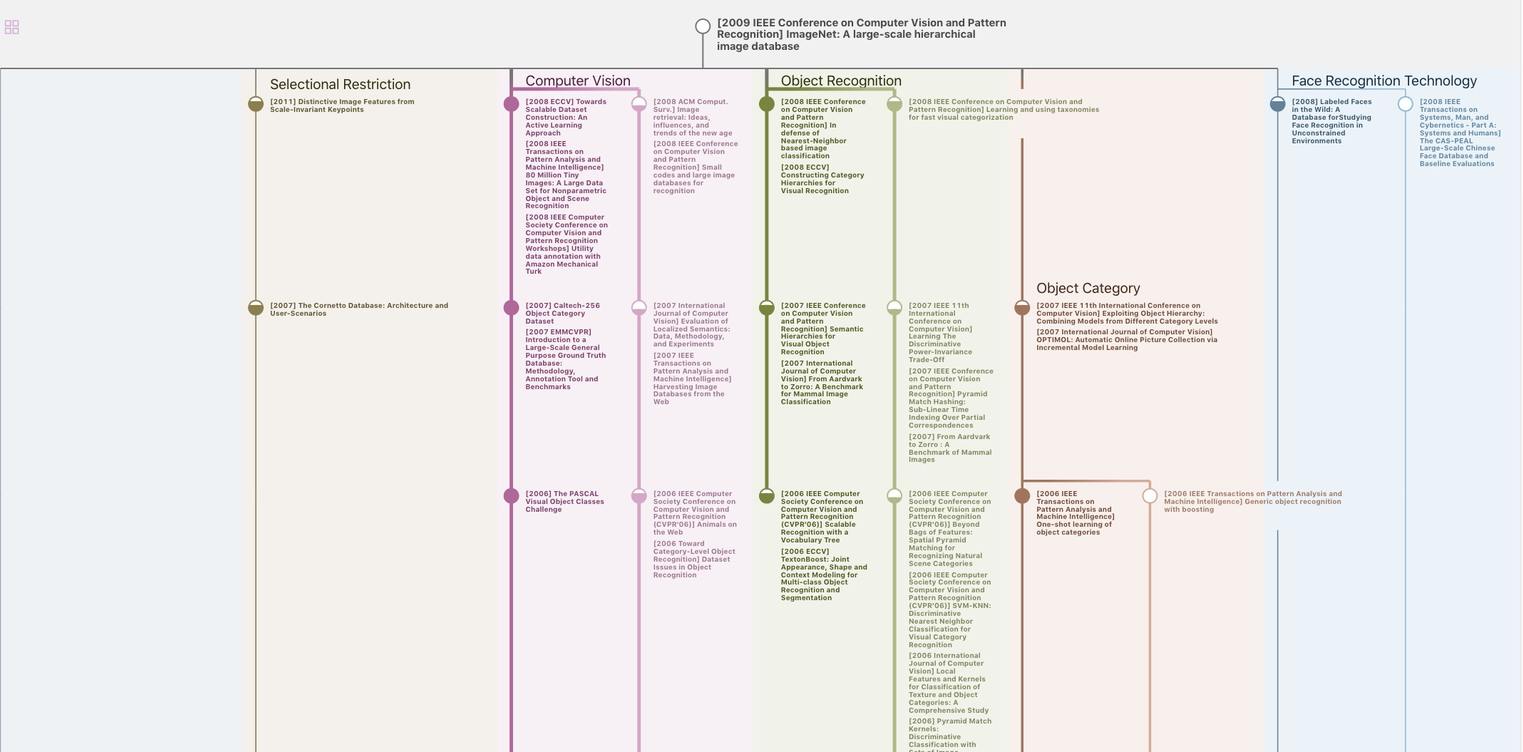
生成溯源树,研究论文发展脉络
Chat Paper
正在生成论文摘要