Reinforcement Learning for on-line Sequence Transformation
2022 17th Conference on Computer Science and Intelligence Systems (FedCSIS)(2022)
摘要
In simultaneous machine translation (SMT), an output sequence should be produced as soon as possible, without reading the whole input sequence. This requirement creates a trade-off between translation delay and quality because less context may be known during translation. In most SMT methods, this trade-off is controlled with parameters whose values need to be tuned. In this paper, we introduce an SMT system that learns with reinforcement and is able to find the optimal delay in training. We conduct experiments on Tatoeba and IWSLT2014 datasets against state-of-the-art translation architectures. Our method achieves comparable results on the former dataset, with better results on long sentences and worse but comparable results on the latter dataset.
更多查看译文
关键词
on-line sequence transformation,simultaneous machine translation,output sequence,SMT system,optimal delay,reinforcement learning
AI 理解论文
溯源树
样例
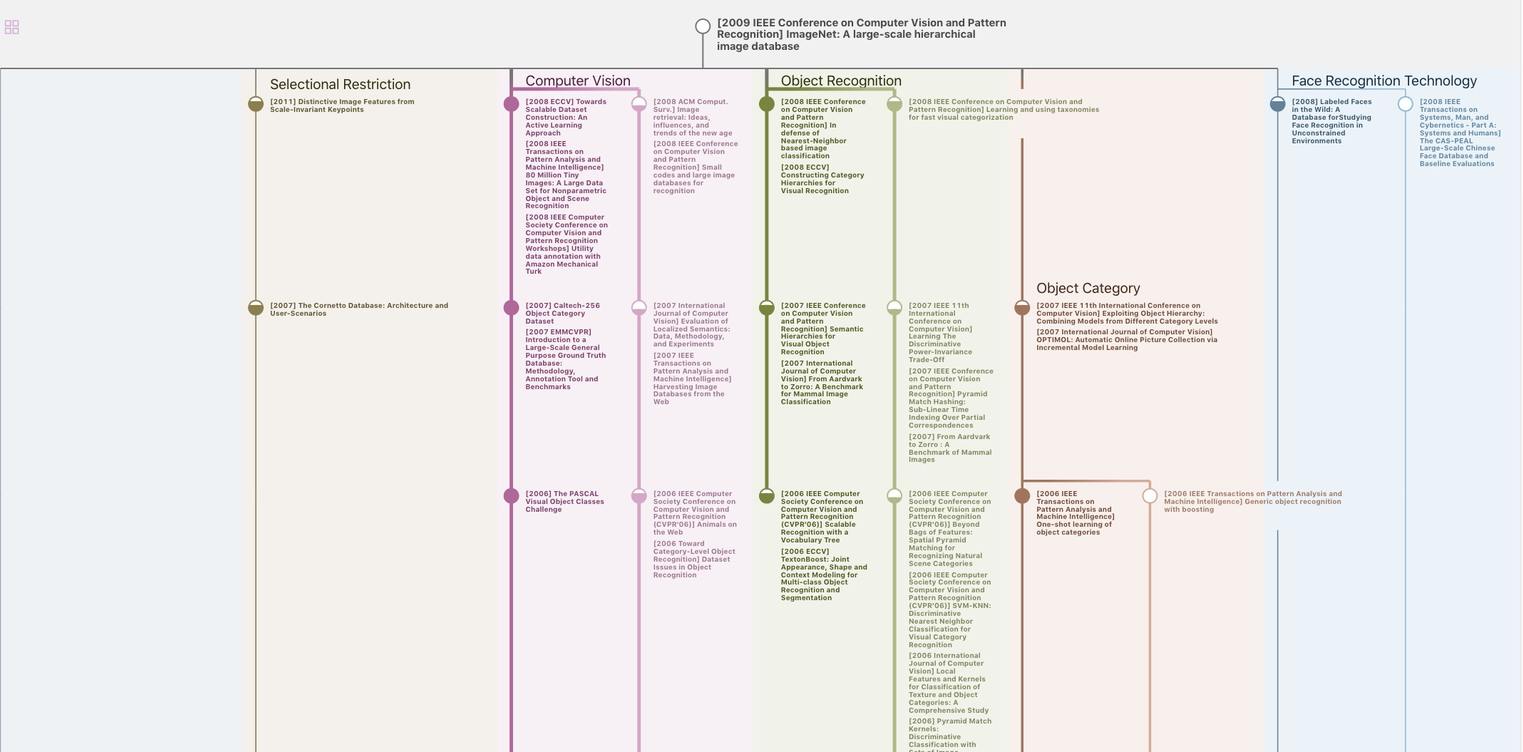
生成溯源树,研究论文发展脉络
Chat Paper
正在生成论文摘要