Detecting Message Modification Attacks on the CAN Bus with Temporal Convolutional Networks
PROCEEDINGS OF THE 7TH INTERNATIONAL CONFERENCE ON VEHICLE TECHNOLOGY AND INTELLIGENT TRANSPORT SYSTEMS (VEHITS)(2021)
摘要
Multiple attacks have shown that in-vehicle networks have vulnerabilities which can be exploited. Securing the Controller Area Network (CAN) for modern vehicles has become a necessary task for car manufacturers. Some attacks inject potentially large amount of fake messages into the CAN network; however, such attacks are relatively easy to detect. In more sophisticated attacks, the original messages are modified, making the detection a more complex problem. In this paper, we present a novel machine learning based intrusion detection method for CAN networks. We focus on detecting message modification attacks, which do not change the timing patterns of communications. Our proposed temporal convolutional network-based solution can learn the normal behavior of CAN signals and differentiate them from malicious ones. The method is evaluated on multiple CAN-bus message IDs from two public datasets including different types of attacks. Performance results show that our lightweight approach compares favorably to the state-of-the-art unsupervised learning approach. achieving similar or better accuracy for a wide range of scenarios with a significantly lower false positive rate.
更多查看译文
关键词
Vehicle Security, Intrusion Detection, Controller Area Network, Machine Learning, Temporal Convolutional Networks
AI 理解论文
溯源树
样例
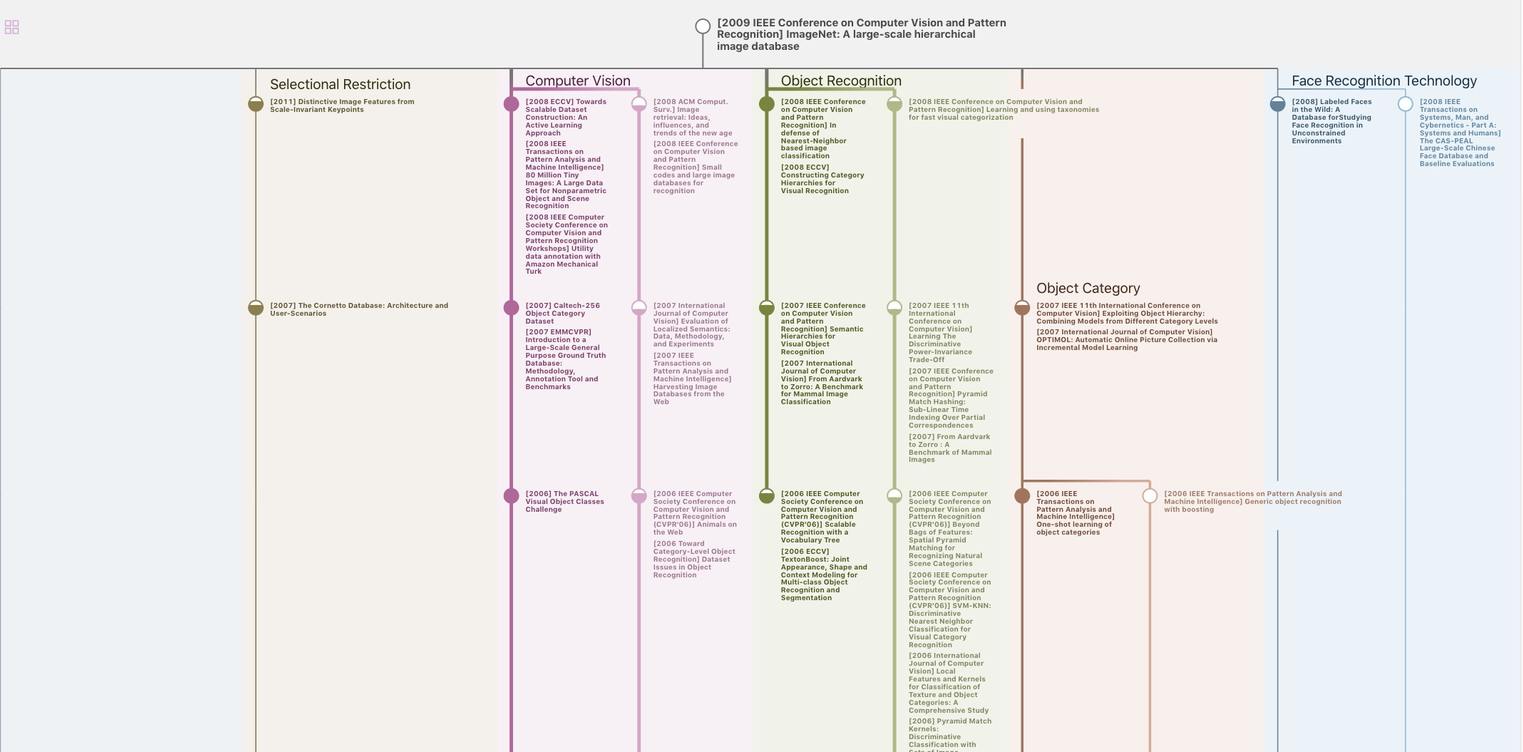
生成溯源树,研究论文发展脉络
Chat Paper
正在生成论文摘要