Training With Data Dependent Dynamic Learning Rates
CoRR(2021)
摘要
Recently many first and second order variants of SGD have been proposed to facilitate training of Deep Neural Networks (DNNs). A common limitation of these works stem from the fact that they use the same learning rate across all instances present in the dataset. This setting is widely adopted under the assumption that loss functions for each instance are similar in nature, and hence, a common learning rate can be used. In this work, we relax this assumption and propose an optimization framework which accounts for difference in loss function characteristics across instances. More specifically, our optimizer learns a dynamic learning rate for each instance present in the dataset. Learning a dynamic learning rate for each instance allows our optimization framework to focus on different modes of training data during optimization. When applied to an image classification task, across different CNN architectures, learning dynamic learning rates leads to consistent gains over standard optimizers. When applied to a dataset containing corrupt instances, our framework reduces the learning rates on noisy instances, and improves over the state-of-the-art. Finally, we show that our optimization framework can be used for personalization of a machine learning model towards a known targeted data distribution.
更多查看译文
关键词
learning,training,rates,data
AI 理解论文
溯源树
样例
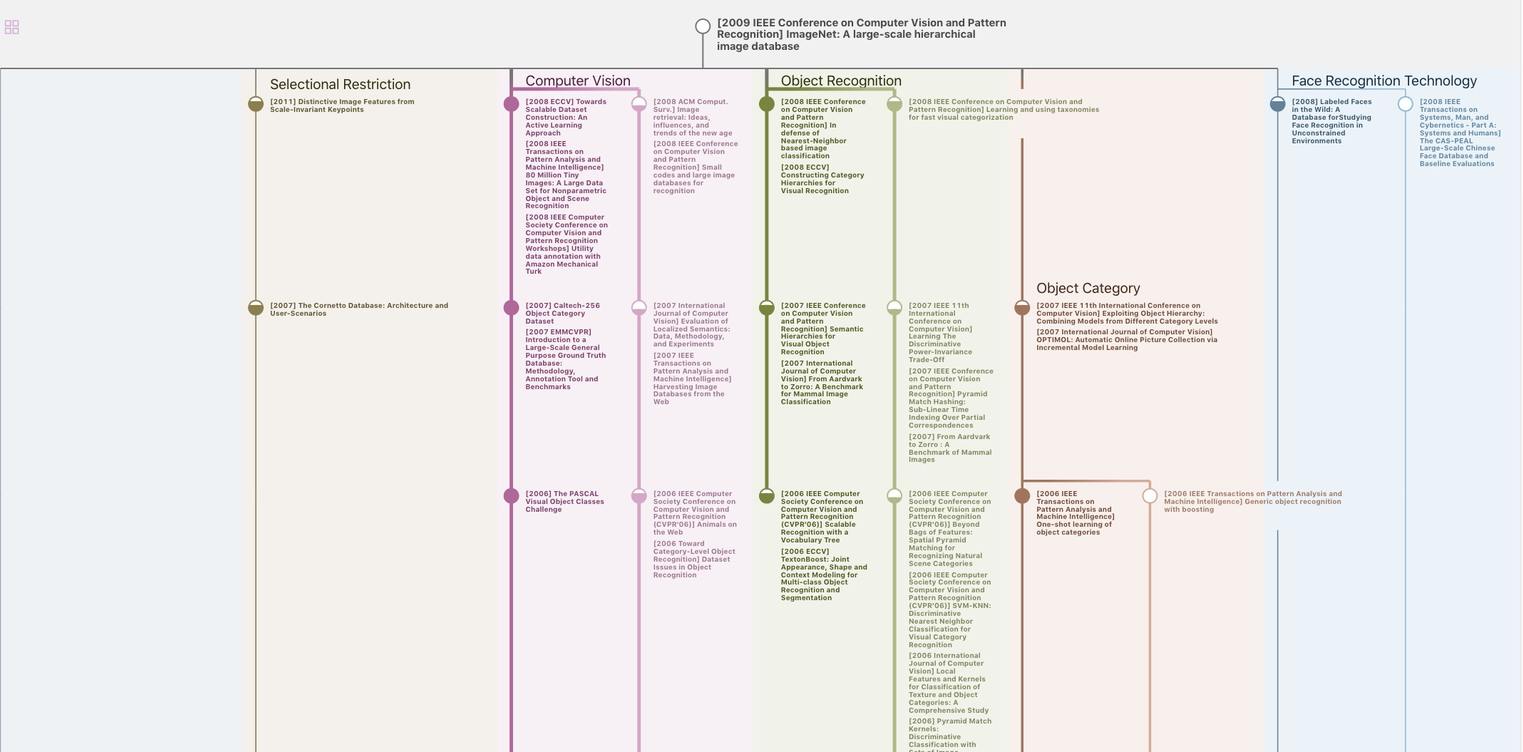
生成溯源树,研究论文发展脉络
Chat Paper
正在生成论文摘要