Deep Learning Improves the Temporal Reproducibility of Aortic Measurement
JOURNAL OF DIGITAL IMAGING(2021)
摘要
Imaging-based measurements form the basis of surgical decision making in patients with aortic aneurysm. Unfortunately, manual measurement suffer from suboptimal temporal reproducibility, which can lead to delayed or unnecessary intervention. We tested the hypothesis that deep learning could improve upon the temporal reproducibility of CT angiography-derived thoracic aortic measurements in the setting of imperfect ground-truth training data. To this end, we trained a standard deep learning segmentation model from which measurements of aortic volume and diameter could be extracted. First, three blinded cardiothoracic radiologists visually confirmed non-inferiority of deep learning segmentation maps with respect to manual segmentation on a 50-patient hold-out test cohort, demonstrating a slight preference for the deep learning method ( p < 1e-5). Next, reproducibility was assessed by evaluating measured change (coefficient of reproducibility and standard deviation) in volume and diameter values extracted from segmentation maps in patients for whom multiple scans were available and whose aortas had been deemed stable over time by visual assessment ( n = 57 patients, 206 scans). Deep learning temporal reproducibility was superior for measures of both volume ( p < 0.008) and diameter ( p < 1e-5) and reproducibility metrics compared favorably with previously reported values of manual inter-rater variability. Our work motivates future efforts to apply deep learning to aortic evaluation.
更多查看译文
AI 理解论文
溯源树
样例
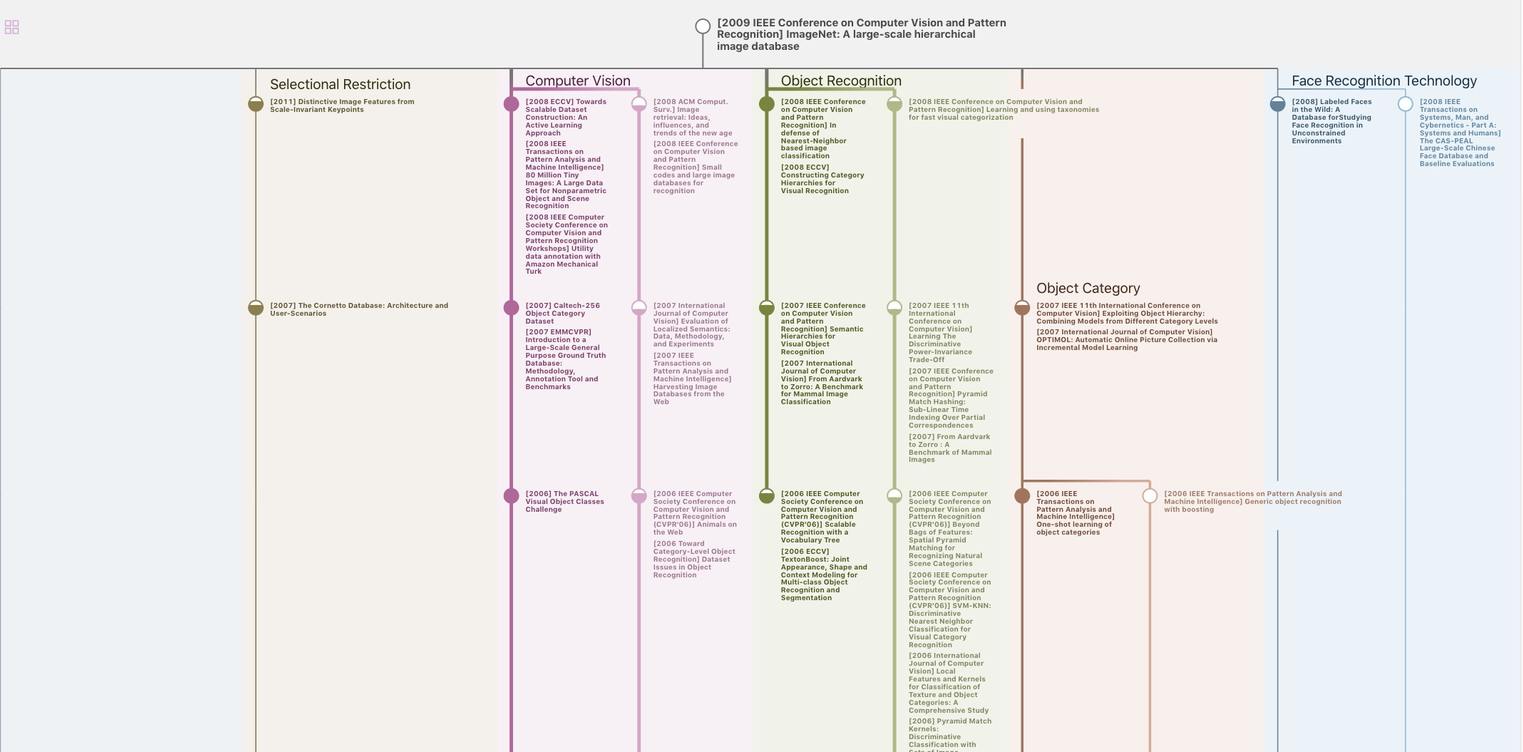
生成溯源树,研究论文发展脉络
Chat Paper
正在生成论文摘要