Globaltest Confidence Regions And Their Application To Ridge Regression
BIOMETRICAL JOURNAL(2021)
摘要
We construct confidence regions in high dimensions by inverting the globaltest statistics, and use them to choose the tuning parameter for penalized regression. The selected model corresponds to the point in the confidence region of the parameters that minimizes the penalty, making it the least complex model that still has acceptable fit according to the test that defines the confidence region. As the globaltest is particularly powerful in the presence of many weak predictors, it connects well to ridge regression, and we thus focus on ridge penalties in this paper. The confidence region method is quick to calculate, intuitive, and gives decent predictive potential. As a tuning parameter selection method it may even outperform classical methods such as cross-validation in terms of mean squared error of prediction, especially when the signal is weak. We illustrate the method for linear models in simulation study and for Cox models in real gene expression data of breast cancer samples.
更多查看译文
关键词
confidence regions, high dimensional, tuning parameter selection
AI 理解论文
溯源树
样例
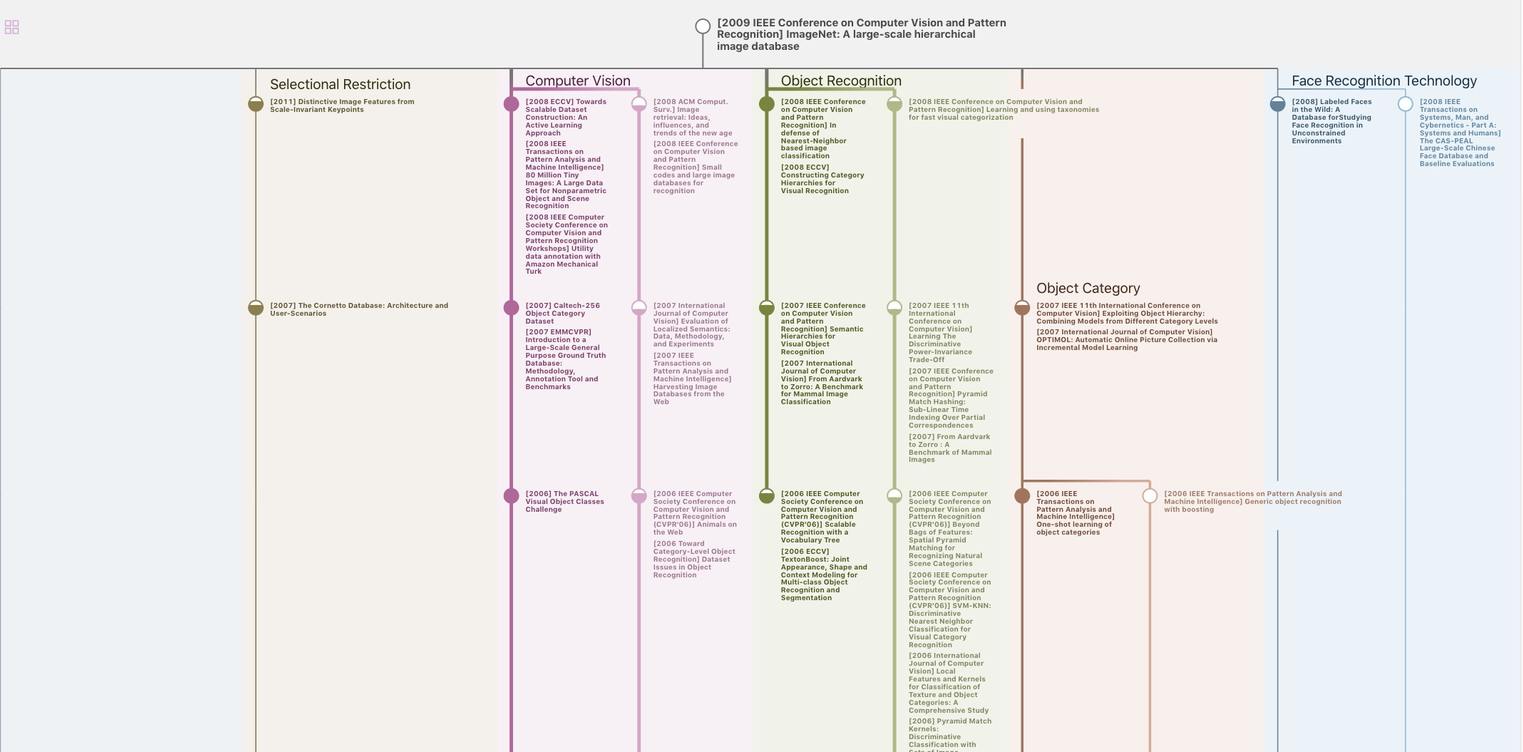
生成溯源树,研究论文发展脉络
Chat Paper
正在生成论文摘要