Multi-Scale Representation Learning on Hypergraph for 3D Shape Retrieval and Recognition.
IEEE TRANSACTIONS ON IMAGE PROCESSING(2021)
摘要
Effective 3D shape retrieval and recognition are challenging but important tasks in computer vision research field, which have attracted much attention in recent decades. Although recent progress has shown significant improvement of deep learning methods on 3D shape retrieval and recognition performance, it is still under investigated of how to jointly learn an optimal representation of 3D shapes considering their relationships. To tackle this issue, we propose a multi-scale representation learning method on hypergraph for 3D shape retrieval and recognition, called multi-scale hypergraph neural network (MHGNN). In this method, the correlation among 3D shapes is formulated in a hypergraph and a hypergraph convolution process is conducted to learn the representations. Here, multiple representations can be obtained through different convolution layers, leading to multi-scale representations of 3D shapes. A fusion module is then introduced to combine these representations for 3D shape retrieval and recognition. The main advantages of our method lie in 1) the high-order correlation among 3D shapes can be investigated in the framework and 2) the joint multi-scale representation can be more robust for comparison. Comparisons with state-of-the-art methods on the public ModelNet40 dataset demonstrate remarkable performance improvement of our proposed method on the 3D shape retrieval task. Meanwhile, experiments on recognition tasks also show better results of our proposed method, which indicate the superiority of our method on learning better representation for retrieval and recognition.
更多查看译文
关键词
Three-dimensional displays, Shape, Feature extraction, Correlation, Convolution, Task analysis, Neural networks, 3D shape retrieval, multi-scale representation, hypergraph neural network, recognition
AI 理解论文
溯源树
样例
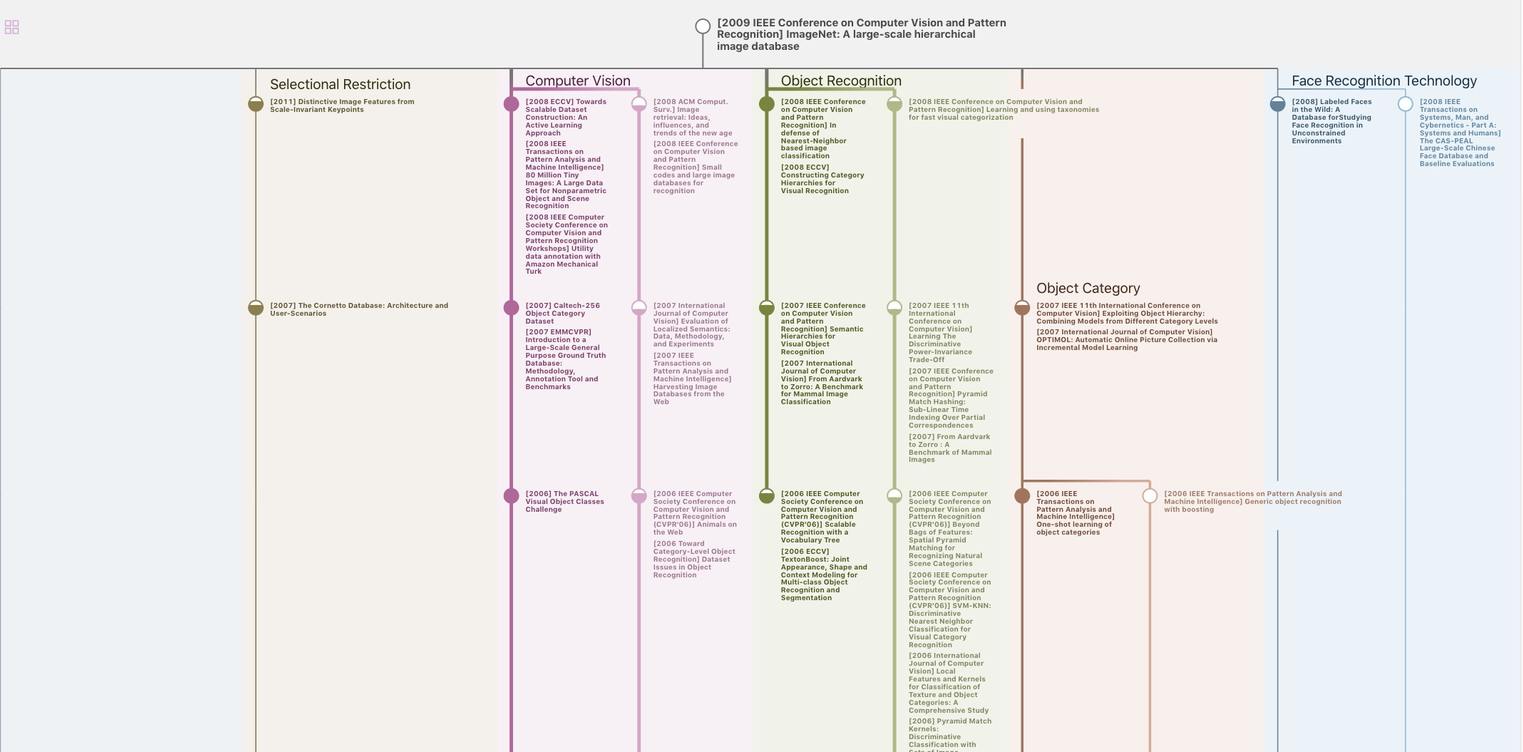
生成溯源树,研究论文发展脉络
Chat Paper
正在生成论文摘要