Bayesian Optimisation for Constrained Problems
ArXiv(2021)
摘要
Many real-world optimisation problems such as hyperparameter tuning in machine learning or simulation-based optimisation can be formulated as expensive-to-evaluate black-box functions. A popular approach to tackle such problems is Bayesian optimisation (BO), which builds a response surface model based on the data collected so far, and uses the mean and uncertainty predicted by the model to decide what information to collect next. In this paper, we propose a novel variant of the well-known Knowledge Gradient acquisition function that allows it to handle constraints. We empirically compare the new algorithm with four other state-of-the-art constrained Bayesian optimisation algorithms and demonstrate its superior performance. We also prove theoretical convergence in the infinite budget limit.
更多查看译文
关键词
constrained problems,optimisation
AI 理解论文
溯源树
样例
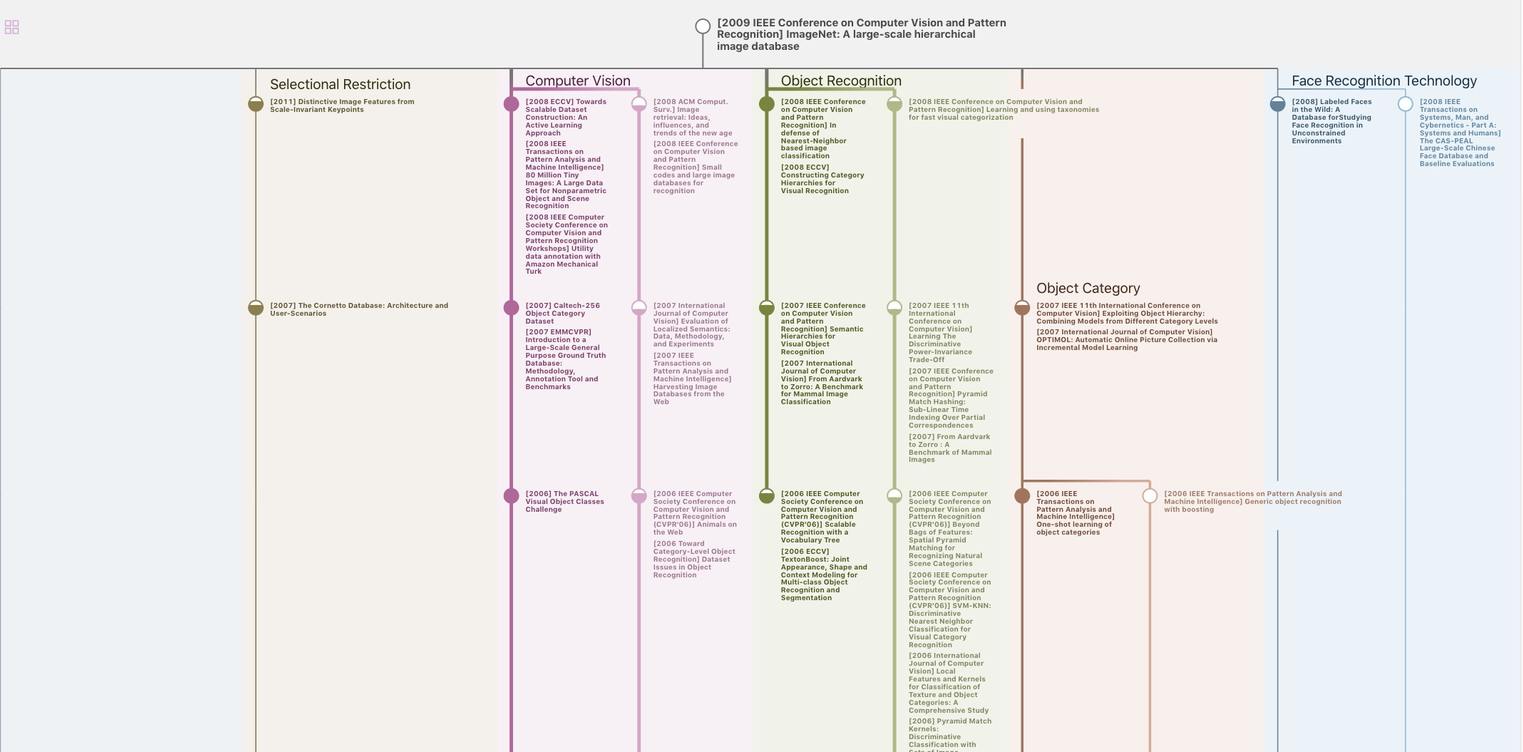
生成溯源树,研究论文发展脉络
Chat Paper
正在生成论文摘要