A Non-asymptotic Approach to Best-Arm Identification for Gaussian Bandits
INTERNATIONAL CONFERENCE ON ARTIFICIAL INTELLIGENCE AND STATISTICS, VOL 151(2022)
摘要
We propose a new strategy for best-arm identification with fixed confidence of Gaussian variables with bounded means and unit variance. This strategy, called EXPLORATION-BIASED SAMPLING, is not only asymptotically optimal: it is to the best of our knowledge the first strategy with non-asymptotic bounds that asymptotically matches the sample complexity. But the main advantage over other algorithms like TRACK-AND-STOP is an improved behavior regarding exploration: EXPLORATION-BIASED SAMPLING is biased towards exploration in a subtle but natural way that makes it more stable and interpretable. These improvements are allowed by a new analysis of the sample complexity optimization problem, which yields a faster numerical resolution scheme and several quantitative regularity results that we believe of high independent interest.
更多查看译文
关键词
identification,non-asymptotic,best-arm
AI 理解论文
溯源树
样例
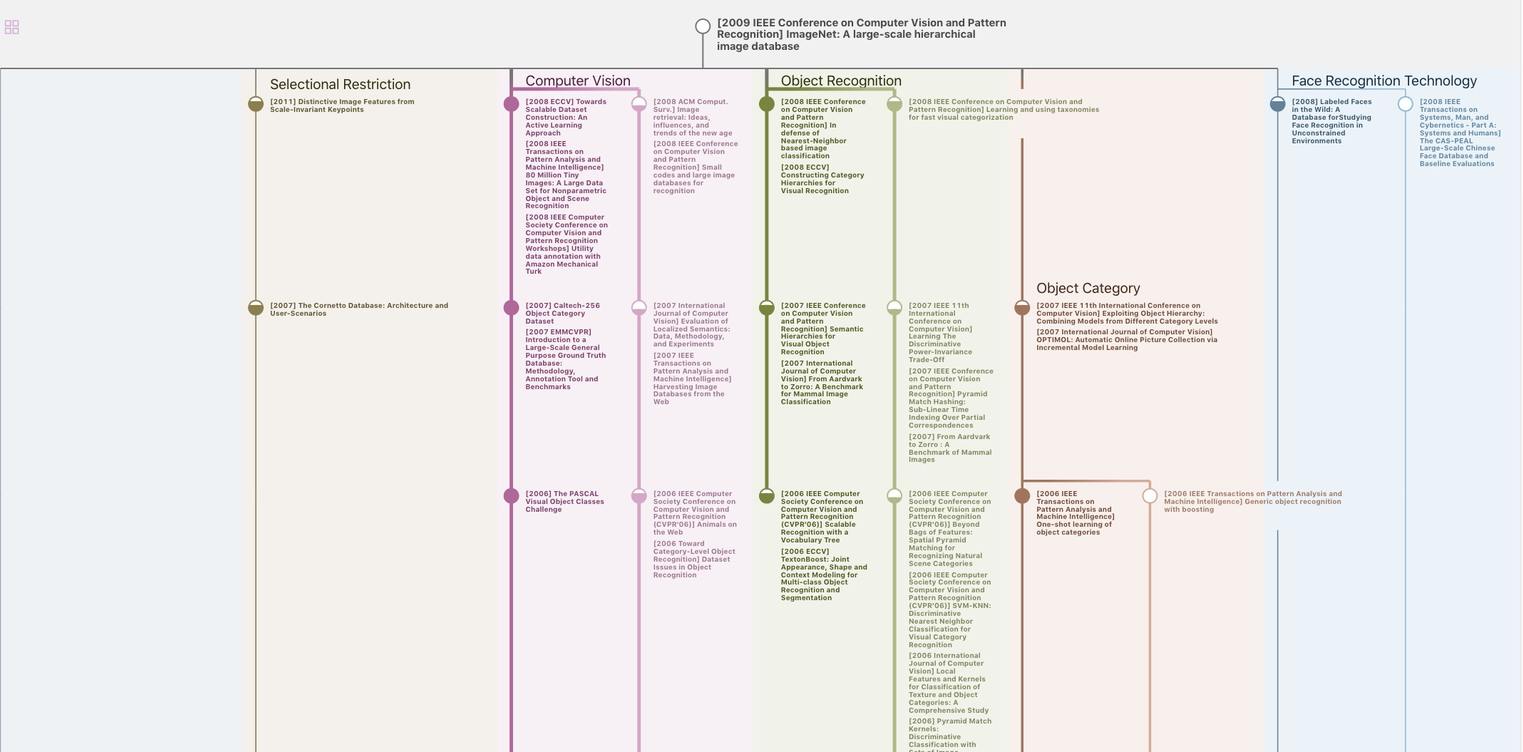
生成溯源树,研究论文发展脉络
Chat Paper
正在生成论文摘要