Hybrid Encoding for Generating Large Scale Game Level Patterns With Local Variations
IEEE TRANSACTIONS ON GAMES(2023)
摘要
Generative adversarial networks (GANs) are a powerful indirect genotype-to-phenotype mapping for evolutionary search. Much previous work applying GANs to level generation focuses on fixed-size segments combined into a whole level, but individual segments may not fit together cohesively. In contrast, segments in human designed levels are often repeated, directly or with variation, and organized into patterns (the symmetric eagle in Level 1 of The Legend of Zelda, or repeated pipe motifs in Super Mario Bros.). Such patterns can be produced with compositional pattern producing networks (CPPNs). CPPNs define latent vector GAN inputs as a function of geometry, organizing segments output by a GAN into complete levels. However, collections of latent vectors can also be evolved directly, producing more chaotic levels. We propose a hybrid approach that evolves CPPNs first, but allows latent vectors to evolve later, combining the benefits of both approaches. These approaches are evaluated in Super Mario Bros. and The Legend of Zelda. We previously demonstrated via a quality-diversity algorithm that CPPNs better cover the space of possible levels than directly evolved levels. Here, we show that the hybrid approach first, covers areas that neither of the other methods can, and second, achieves comparable or superior quality diversity (QD) scores.
更多查看译文
关键词
Games,Generators,Generative adversarial networks,Training,Encoding,Neural networks,Switches,Compositional pattern producing network (CPPN),generative adversarial network (GAN),indirect encoding,procedural content generation via machine learning
AI 理解论文
溯源树
样例
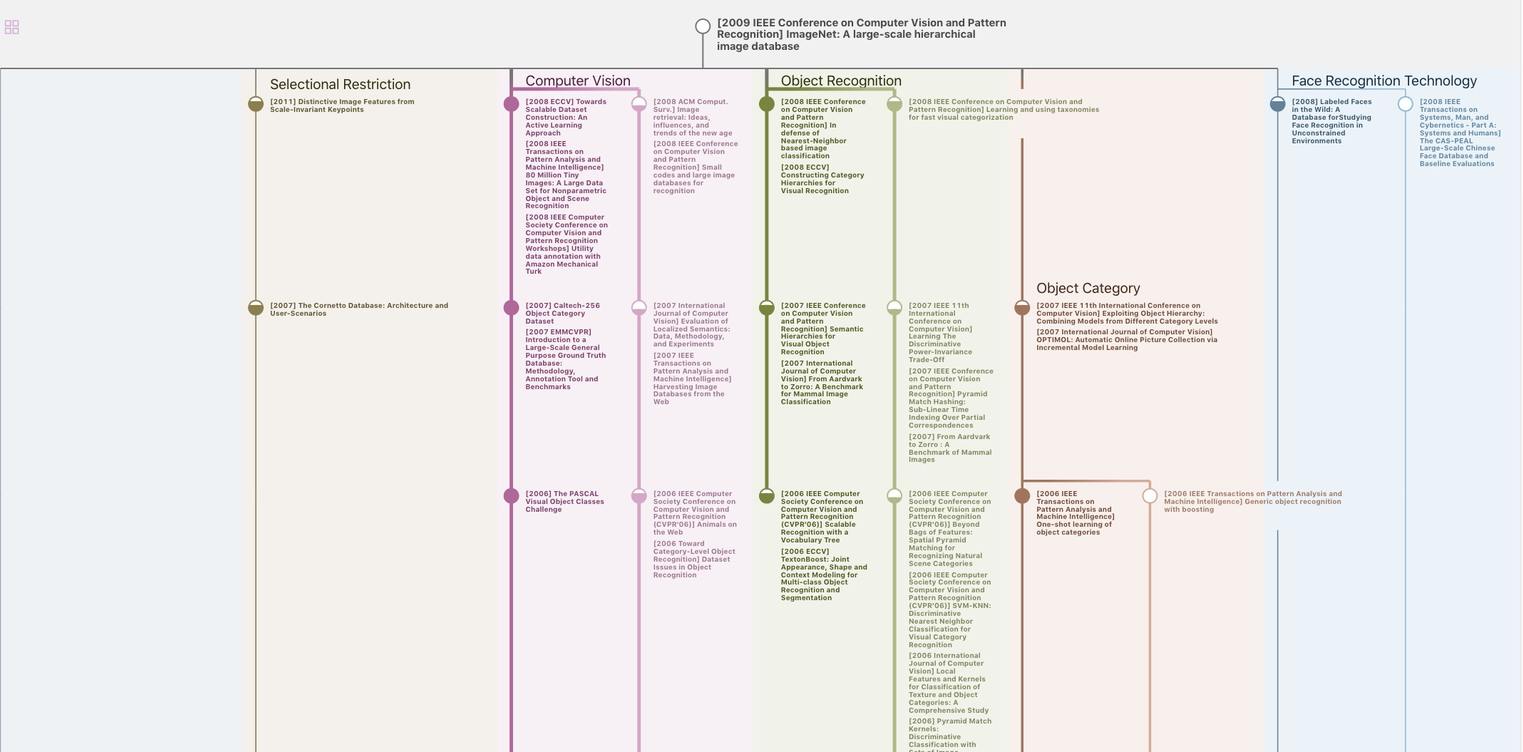
生成溯源树,研究论文发展脉络
Chat Paper
正在生成论文摘要