Self-Supervised Monocular Depth Learning In Low-Texture Areas
REMOTE SENSING(2021)
摘要
For the task of monocular depth estimation, self-supervised learning supervises training by calculating the pixel difference between the target image and the warped reference image, obtaining results comparable to those with full supervision. However, the problematic pixels in low-texture regions are ignored, since most researchers think that no pixels violate the assumption of camera motion, taking stereo pairs as the input in self-supervised learning, which leads to the optimization problem in these regions. To tackle this problem, we perform photometric loss using the lowest-level feature maps instead and implement first- and second-order smoothing to the depth, ensuring consistent gradients ring optimization. Given the shortcomings of ResNet as the backbone, we propose a new depth estimation network architecture to improve edge location accuracy and obtain clear outline information even in smoothed low-texture boundaries. To acquire more stable and reliable quantitative evaluation results, we introce a virtual data set in the self-supervised task because these have dense depth maps corresponding to pixel by pixel. We achieve performance that exceeds that of the prior methods on both the Eigen Splits of the KITTI and VKITTI2 data sets taking stereo pairs as the input.
更多查看译文
关键词
self-supervised, depth estimation, low-texture
AI 理解论文
溯源树
样例
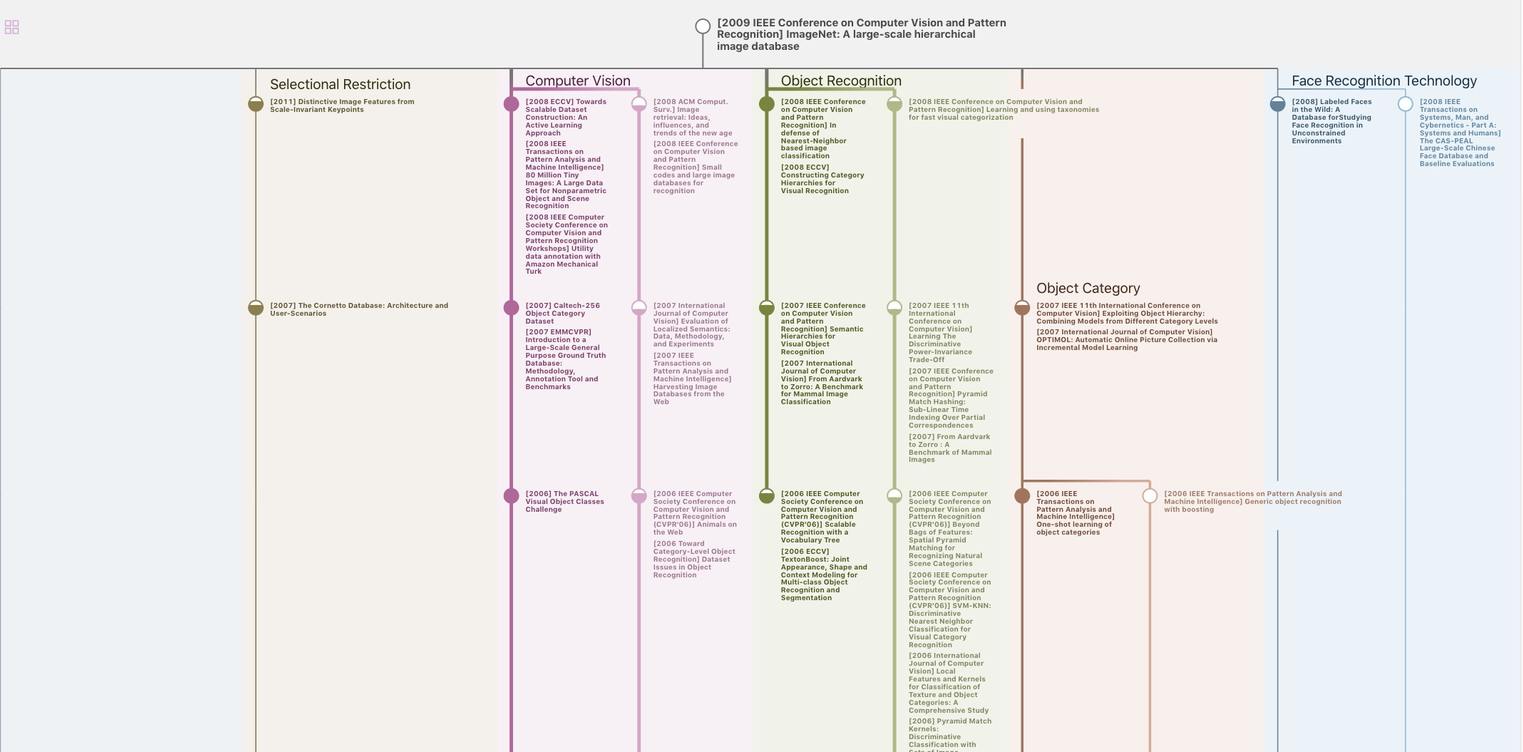
生成溯源树,研究论文发展脉络
Chat Paper
正在生成论文摘要